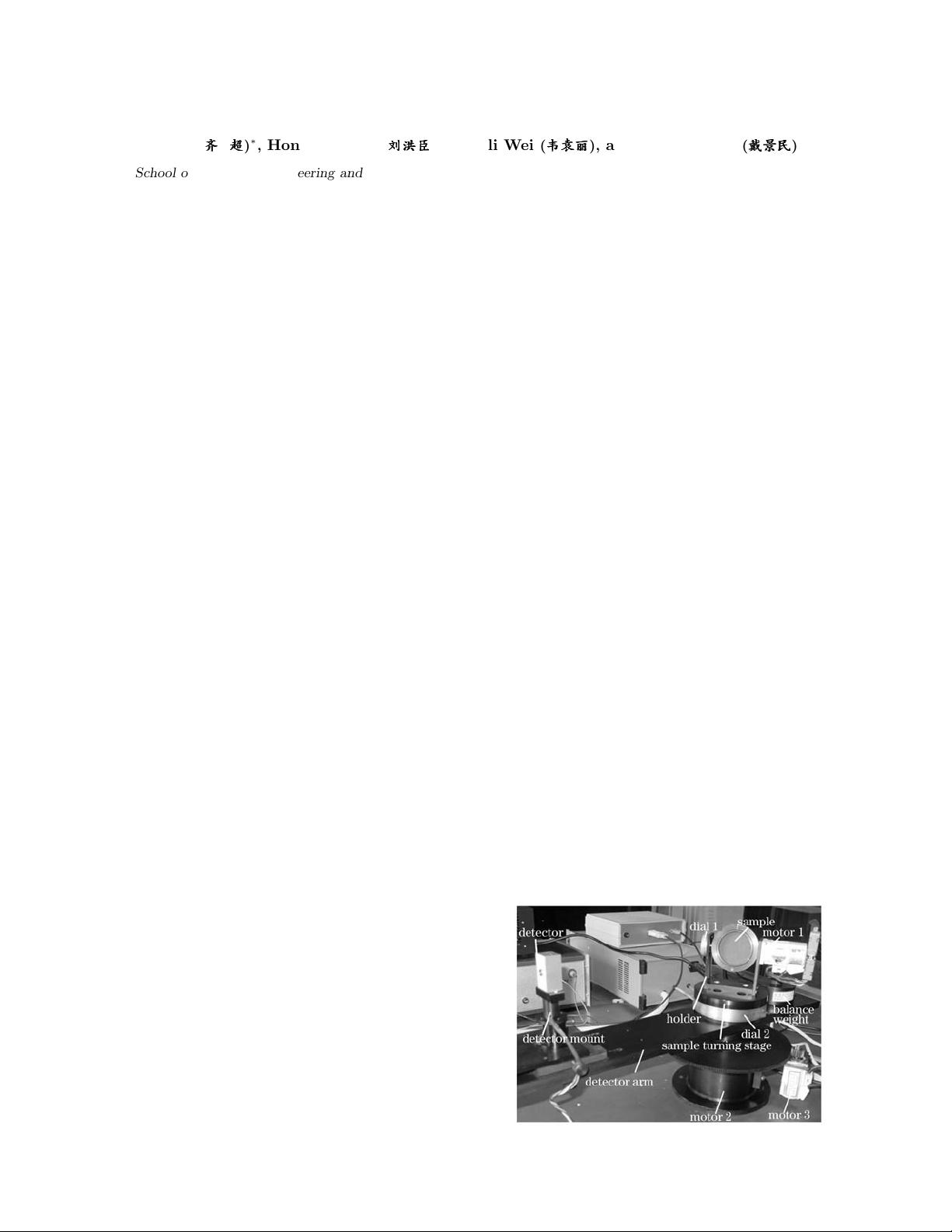
May 10, 2009 / Vol. 7, No. 5 / C HINESE OPTICS LETTERS 403
Design and experimental research of angle
self-compensation setup for BSDF measurement
Chao Qi (
)
∗
, Hongchen Liu (
), Yu anl i Wei (
ÛÛÛ
), and Jingmin Dai (
)
School of Electrical Engineering and Automation, Harbin Institute of Technology, Harbin 150001, China
∗
E-mail: qichao@hit.edu.cn
Received July 11, 2008
When using a single reference to measure the bi-directional scattering distribution function (BSDF), the
incident zenith angle of the tested sample must b e identical to that of the reference. In order to get
the hemisphere space scattering characteristic on the sample surface, usually a motor drives the sample
tilting, then the incident zenith angle is changed and needs to be the compensated by another motor. We
mathematically deduce the expression of compensation angle when the incident zenith angle is changed by
the rotation of motor. After the incident angle is compensated, the scattering zenith angle and azimuth
angle are deduced too. The uncertainty of the system is 0.75%. Scattering measurements are performed
on copper sample with visible light under different temperatures.
OCIS codes: 120.5820, 220.4830, 070.4560.
doi: 10.3788/COL20090705.0403.
Most objects have anisotropic reflectance, which can
be described by the bi-directional scattering distri-
bution function (BSDF). It is very important to ob-
tain high quality bi-directional scattering data sets
for BSDF research and applications. Those data
sets may be used to validate the present various bi-
directional reflectance/transmittance distribution func-
tion (BRDF/BTDF) models and retrieve the sur-
face characteristics of the objects in remote sensing
applications
[1,2]
. According to Ref. [3], the BSDF is
a ratio of the scattering radiance to the incident irra-
diance. It mostly depends on four angles (two for the
incident radiation and two for the scattering radiation)
and is a function of the wavelength, surface roughness,
material properties, and temperature. The characteris-
tics of spatial light scattering from material surface can
be effectively described by BSDF, so BSDF is gener-
ally applied in several fields, such as national defense,
military affairs, soil resource remote sensing, vegetation
monitoring, environment and climate monitoring, stray
light suppression, etc.
[4−9]
.
There are two ways to obtain the entire zenith angle
and azimuth angle which are covered by light on the sam-
ple. The first is to mount the light source on a movable
arm or to use a turning mirror on a movable arm, and to
use a detector to measure the distribution of scattering
light by moving it around the entire hemisphere. To
measure the full BSDF, this process must be repeated
many times, moving the light source again and again to
measure different incident angles. So this method has
been discarded. The second is to fix the light source and
to move the sample or its roll and yaw axes, so that the
scattering light goes into the constrained detector which
can only move in one plane.
Based on the second method described above, we de-
signed the angle self-compensation setup with three de-
grees of freedom for scattering measurement, as shown in
Fig. 1. The crankshaft of the No. 1 motor is parallel to
the table-board and perpendicular to the normal of the
sample plane. This mototr drives the sample running
within the range from −180
◦
to +180
◦
.Atthesame
time, the crankshaft of the No. 2 motor intersects that
of the No.1 motor at the center of the sample, plumbs
in the bottom, and drives the No. 1 motor and the sam-
ple rotating together. It is obvious that the direction
of the No. 1 motor crankshaft is variable in the space
plane during the process of running. In addition, the
scope that the No. 2 motor drives the sample turntable
running is also from −180
◦
to +180
◦
, and the angles
of sloping running and rotating are indicated by dial 1
and dial 2, respectively. The combination of the No.
1 and the No. 2 motors can implement the changes of
and the incident zenith angle θ
i
and the azimuth angle
ϕ
i
. The No. 3 motor crankshaft is parallel to that of
No. 2, which drives the detector arm rotating around
the turntable axis within the range from −180
◦
to +180
◦
via a decelerating gear. A detector is located on an ad-
justable bracket in the hopper chute of the detector arm,
and the distance from the detector to the sample can be
adjusted, so the scattering radiance which comes from
the sample can be measured as the function of space scat-
tering angle (θ
S
,ϕ
S
). The control of the sample direction
and the acquisition of the measured data are completed
by the computer automatically, therefore we can study
the space scattering property and spectral characteristic
of the objective material.
Fig. 1. BSDF measurement setup.
1671-7694/2009/050403-04
c
2009 Chinese Optics Letters