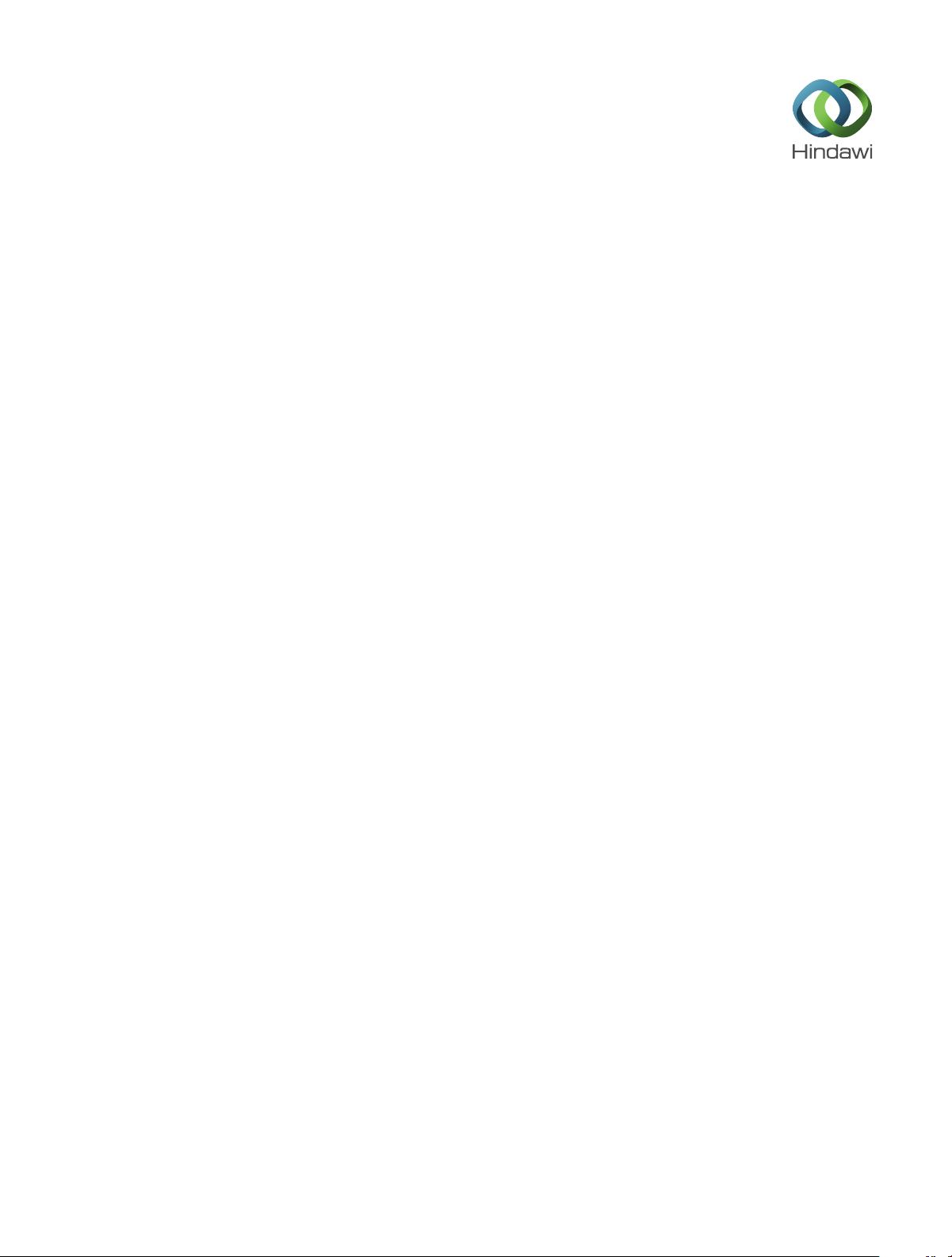
Hindawi Publishing Corporation
Journal of Applied Mathematics
Volume , Article ID , pages
http://dx.doi.org/.//
Research Article
Multi-Innovation Stochastic Gradient Identification Algorithm
for Hammerstein Controlled Autoregressive Autoregressive
Systems Based on the Key Term Separation Principle and on
the Model Decomposition
Huiyi Hu,
1
Xiao Yongsong,
1
and Rui Ding
2
1
Key Laboratory of Advanced Process Control for Light Industry (Ministry of Education), Jiangnan University, Wuxi 214122, China
2
School of Internet of ings Engineering, Jiangnan University, Wuxi 214122, China
Correspondence should be addressed to Rui Ding; rding@.com
Received June ; Revised August ; Accepted September
Academic Editor: Reinaldo Martinez Palhares
Copyright © Huiyi Hu et al. is is an open access article distributed under the Creative Commons Attribution License, which
permits unrestricted use, distribution, and reproduction in any medium, provided the original work is properly cited.
An input nonlinear system is decomposed into two subsystems, one including the parameters of the system model and the other
including the parameters of the noise model, and a multi-innovation stochastic gradient algorithm is presented for Hammerstein
controlled autoregressive autoregressive (H-CARAR) systems based on the key term separation principle and on the model
decomposition, in order to improve the convergence speed of the stochastic gradient algorithm. e key term separation principle
can simplify the identication model of the input nonlinear system, and the decomposition technique can enhance computational
eciencies of identication algorithms. e simulation results show that the proposed algorithm is eective for estimating the
parameters of IN-CARAR systems.
1. Introduction
ere exist many nonlinear systems in process control [–
].Anonlinearsystemcanbemodeledbyinputnonlinear
systems [] and output nonlinear systems [], input-output
nonlinear systems [], feedback nonlinear systems [], and so
on. Input nonlinear systems, which are called Hammerstein
systems [], include input nonlinear equation error type sys-
tems and input nonlinear output error type systems. Recently,
many identication algorithms have been developed for input
nonlinear systems, such as the iterative methods [–], the
separable least squares methods [, ], the blind methods
[], the subspace methods [], and the overparameteriza-
tion methods [, ]. Some methods require paying much
extra computation.
e stochastic gradient (SG) algorithm is widely applied
to parameter estimation. For example, Wang and Ding pre-
sented an extended SG identication algorithm for Hammer-
stein-Wiener ARMAX systems [],butitiswellknownthat
the SG algorithm has slower convergence rates. In order to
improvetheconvergencerateoftheSGalgorithm,Xiaoetal.
presented a multi-innovation stochastic gradient parameter
estimation algorithm for input nonlinear controlled autore-
gressive (IN-CAR) models using the over-parameterization
method []; Chen et al. proposed a modied stochastic
gradient algorithm by introducing a convergence index in
order to improve the convergence rate of the parameter
estimation []; Han and Ding developed a multi-innovation
stochastic gradient algorithm for multi-input single-output
systems [];Liuetal.studiedtheperformanceofthestochas-
tic gradient algorithm for multivariable systems [].
e decomposition identication techniques include
matrix decomposition and model decomposition. Hu and
Ding presented a least squares based iterative identication
algorithm for controlled moving average systems using the
matrix decomposition []; Ding derived an iterative least
squares algorithm to estimate the parameters of output
error systems, and the matrix decomposition can enhance
computational eciencies []. Ding also divided a Ham-
merstein nonlinear system into two subsystems based on the