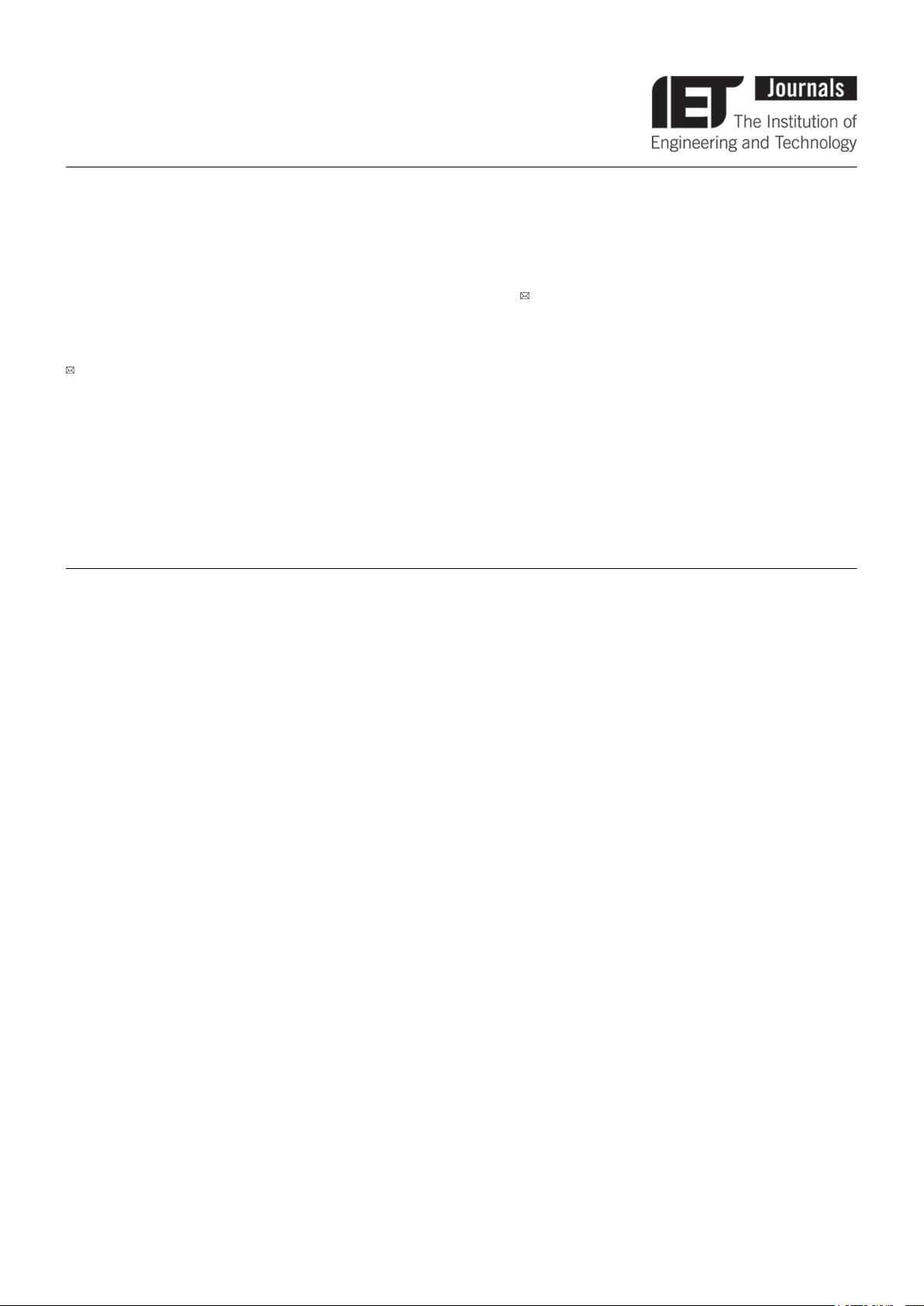
IET Image Processing
Research Article
View's dependency and low-rank background-
guided compressed sensing for multi-view
image joint reconstruction
ISSN 1751-9659
Received on 5th April 2019
Revised 6th July 2019
Accepted on 29th July 2019
doi: 10.1049/iet-ipr.2019.0295
www.ietdl.org
Xuan Fei
1,2
, Lei Li
1
, Heling Cao
1
, Jianyu Miao
1
, Renping Yu
3
1
College of Information Science and Engineering, Henan University of Technology, Zhengzhou 450001, People's Republic of China
2
Key Laboratory of Grain Information Processing and Control, Ministry of Education, Zhengzhou 450001, People's Republic of China
3
School of Electrical Engineering, Zhengzhou University, Zhengzhou 450001, People's Republic of China
E-mail: yurenping@zzu.edu.cn
Abstract: Compressed sensing (CS) multi-camera network reconstruction has attracted much attention in the field of distributed
CS networks. However, many multi-camera network reconstructions based on CS usually recover every image separately; the
view's dependency and geometrical structure among these multi-view images could be rarely considered in this way, which will
result in some unsatisfied joint reconstruction results. Here, the authors introduce to extract the multiple view geometry from
multi-view images to construct the view's dependency observation model. Based on the proposed parametric transformation
observation model, they propose a novel CS joint reconstruction method of multi-view image that guided by the spatial
correlation and low-rank background constraints. The eventual optimisation model could be relaxed to a series of convex
optimisation problems, which could be efficiently solved by combining the variable splitting and alternate iteration technique. The
extended experimental results indicate that they proposed method has achieved a remarkable improvement in both objective
criterion and visual fidelity compared with other competitive reconstruction methods.
1 Introduction
Building on the premise of distributed vision-based sensing and
processing, multi-camera network (MCN) [1] enable many novel
applications in smart environments, such as deep space
exploration, unmanned surveillance, dangerous area monitoring,
behaviour analysis, patient and elderly care, ambient intelligence,
and so on. User interactivity and context awareness, with the
possibility of fusion with other sensing modalities, offer additional
richness in multi-camera networks and create opportunities for
developing user-centric applications. For instance, multi-camera
surveillance system has the advantage of wide range of monitoring,
broad angle of observing, capturing comprehensive information, so
it is applied into a lot of places by many organisations. However,
with the cost of image sensors, embedded processing, and
supporting network infrastructure decreasing, the potential for a
dramatic increase in the scale of camera networks can be realised,
increasing the difficulty of data acquisition, storage, transmission,
and processing.
In truth, according to the Nyquist sampling theorem, the
sampling rate of traditional multi-camera network must be greater
than two times of the signal bandwidth, in order to achieve
accurate signal reconstruction. Therefore, a high-quality
reconstruction of complex scenes must require a large amount of
sampling data and the corresponding transmission cost of data. Due
to the redundancy of the signal itself, it is necessary to bring
forward a high-level compression before transmission, and a
corresponding high-quality decoding at the receiving end.
However, this typical signal processing mode is not enough to meet
the actual requirements of the multi-camera network that
constrained with limited bandwidth and low power consumption.
How to reduce the number of distributed sampling measurements
(encoder) and improve the quality of the reconstructed image
(decoder) simultaneously becomes an important problem to be
solved urgently in the multi-camera network.
Recently, inspired by distributed source coding principles [2, 3]
and compressed sensing (CS) theory [4–6], CS-based multi-view
image reconstruction was proposed [7], in which each camera
independently captures and compresses one view of the same scene
by taking a small number of random linear measurements. It breaks
the limitation of traditional signal sampling, compressing as well as
the imaging theory that all based on the Nyquist sampling theorem,
and provides a new way for the research of the low power and
bandwidth limited CS camera network.
Compared with traditional multi-camera network
reconstruction, CS-based multi-view image reconstruction has
some benefits as following: (a) the signal only needs to be linear
projected with a random observation matrix, and then the
corresponding compressed observation vector can be achieved, so
the computational complexity is low at the encoder step. (b) For N-
dimensional original signal with k-sparsity, only M-dimensional
observation vector (M is much <N) is enough to reconstruct it. (c)
For the same coding scheme, different decoding techniques can be
employed, so the encoding and decoding processes are
independent. These above advantages make CS especially suitable
for the resource constrained multi-camera network.
In this paper, we consider a distributed CS multi-view image
encoder and aim at developing an effective sparsity-aware decoder
to recover the multi-view image data from the CS measurements.
The contributions of our work lie in three aspects. First, as the
multi-view data originate from the same scene, the spatial
correlation and sparsity of the multi-view imagery can be exploited
for regularised representation from four aspects: spatial correlation
prior between multi-view images, low-rank prior between
background images, TV-based background image sparsity prior,
Haar-wavelet-transformation-based foreground image sparsity
prior. Then, we propose a view's dependency and low-rank
background-guided CS optimisation model for multi-view image
joint reconstruction. Finally, we design a solution algorithm based
on variable splitting and alternating direction method of
multipliers. Experimental results show that the proposed model and
algorithm can reconstruct multi-view images effectively, especially
for edge and texture parts.
The remainder of this paper is organised as follows. Section 2 is
an overview of the related works on CS-based multi-view imaging
system and reconstruction. In Section 3, four types of multi-view
image spatial correlation and sparsity priors are introduced into
reconstructed model. In Section 4, the proposed view's dependency
and low-rank background-guided joint reconstruction minimisation
decoder is developed with a detailed description of the solution
IET Image Process.
© The Institution of Engineering and Technology 2019
1