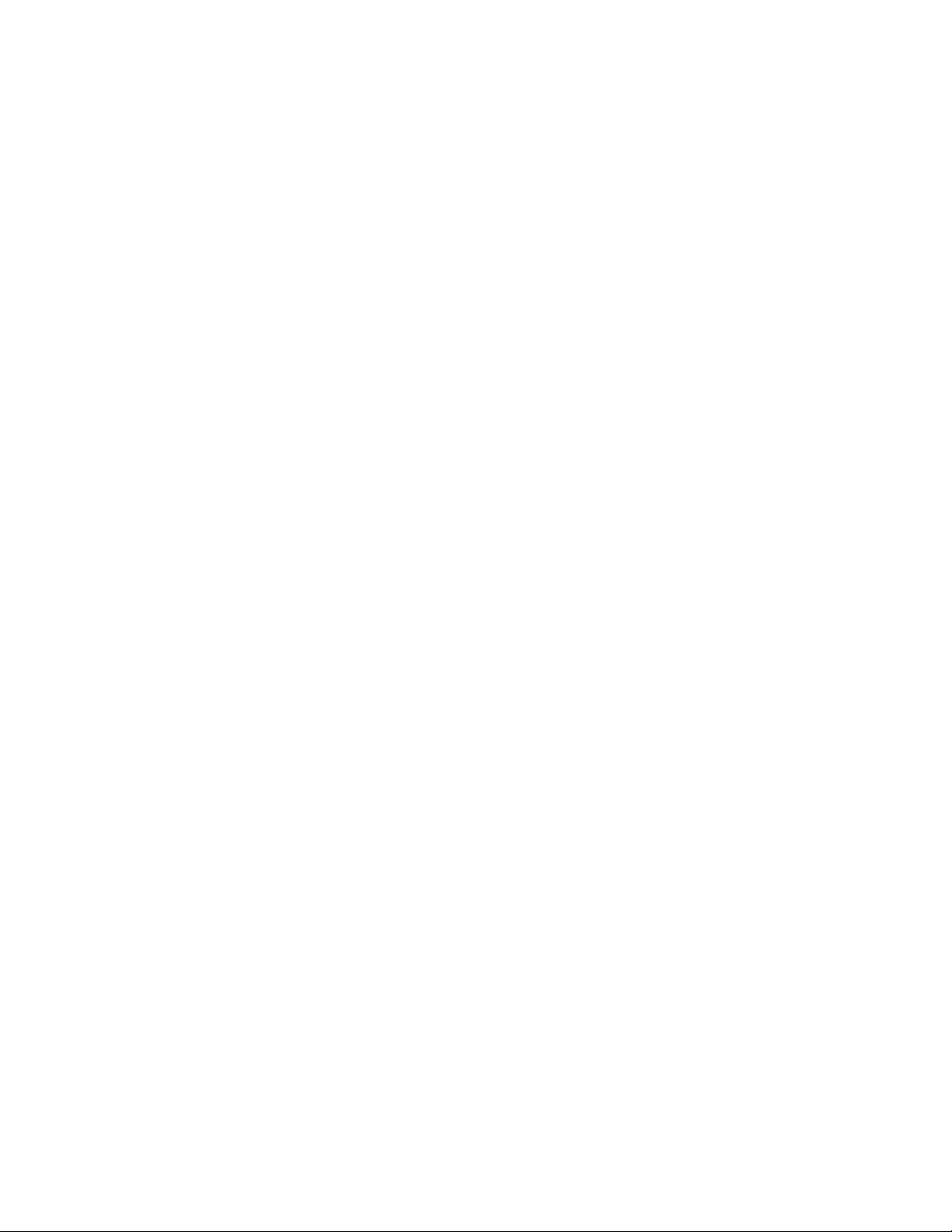
A Code-aided and Moment-based Joint SNR
Estimation for
𝑀 -APSK over AWGN Channels
Rui He
∗
,DeweiYang
∗
, Hua Wang
∗
, Jingming Kuang
∗
, and Xiaojie Wen
†
∗
School of Information and Electronics, Beijing Institute of Technology Beijing, China
†
Beijing Institute of Satellite Information Engineering, Beijing, China
Abstract—In this paper, a code-aided maximum-likelihood and
moment-based joint SNR estimator is proposed for 𝑀 -ary am-
plitude phase shift keying (APSK) signals over AWGN channels.
The proposed estimator significantly improves the performance
at low SNRs by utilizing the syndrome in the LDPC codes
to act as a reference measurement of estimation performance.
Moreover, a methodology to measure the performance of the
estimation by the syndrome of the LDPC codes is derived and the
simulation results reveal that the number of 0s in the syndrome
presents a positive correlation with the SNR. Compared with
code-aided maximum-likelihood (ML) estimators and moment-
based estimators for 𝑀 -APSK signals, it is validated that the
proposed joint SNR estimator has integrated the advantages of
the classical approaches and simulation results also show that the
proposed estimator exploiting the decision metric of the selector
performs better at the SNR estimation range.
Index Terms—syndrome, SNR estimation, LDPC code, APSK.
I. INTRODUCTION
S
IGNAL-TO-NOISE (SNR) ratio plays an indispensable
role in the applications such as adaptive coding and
modulation of Digital Video Broadcasting Second Generation
(DVB-S2) [1], low density parity check (LDPC) decoding [2]
and iterative demodulation. Precise estimation is essential to
an excellent performance. Generally, there are two categories
of SNR estimation techniques, namely data-aided (DA) and
non-data-aided (NDA). The former has a more excellent
performance but requires pilot symbols or training sequences
with sacrifice of the throughput, while the latter doesn’t need
additional data and tends to perform blind estimation.
NDA approaches, studied and applied extensively, can be
usually divided into maximum-likelihood algorithm (ML)
and moment-based method (MM). ML-based estimators are
based on solving the parameters that maximize the likelihood
functions established on the system model. At first, classical
ML schemes, like RxDA [3], were only suited for some
low-order constellations. After plenty of effort, an iterative
form named as Expectation Maximisation (EM) [4], [5] was
derived from ML schemes to deal with some situations without
closed-form solutions. And for the accuracy an increasing
number of schemes on ML algorithm start to take specific and
unideal factors into consideration, such as 𝑝𝑟𝑖𝑜𝑟 and 𝑝𝑜𝑠𝑡𝑒𝑟𝑖𝑜𝑟
information. Hereinto, the code-aided ML estimator [7], [8],
This work is supported by the “National Science Foundation of China
(NSFC)” (No.61471037, 61401030) and the “Foundation for Basic Research
of Beijing Institute of Technology” (20140542010).
Corresponding Author: Dewei Yang(davidyang@bit.edu.cn)
exploiting the 𝑝𝑜𝑠𝑡𝑒𝑟𝑖𝑜𝑟 information from the decoding mod-
ule, becomes one of the most common schemes. Dissimilarly,
MM is to find the relational formula between statistical mo-
ments and the SNR value. At the very start, moment-based
estimators like 𝑀
1
𝑀
2
and 𝑀
2
𝑀
4
were rarely competent for
complex-noise channels and non-constant constellations, such
as 16APSK or 32APSK, hence much progress has been made
for a better applicability, series of advanced schemes have
been brought out in succession. 𝑀
2
𝑀
4
𝑀
6
estimator [9] was
proposed with a promoted behaviour and the author gives the
optimal parameter for 16APSK. Then eighth-order statistics
(EOS) [10] estimator, compatible with 16APSK and 32APSK,
was put forward, which tries to utilize multiple weight factors
to minimize the variance to get a promoted behaviour at high
SNRs. Currently, a method named eigenvalue decomposition
[11] was newly proposed with the knowledge of statistical
moments for modulations in DVB-S2. Ordinarily, ML and
MM estimators work better in distinctive intervals of SNR,
but correspondingly have a deteriorated performance at the
other range, which happens to complement one another.
In this paper, we propose a new code-aided mixed method
for 𝑀-APSK, which integrates a LPDC code-aided maximum-
likelihood algorithm and a moment-based method and give
its performance simulation and analysis. This method uses
two sub-estimators to estimate the value at the same time,
and then take the syndrome vector of the LDPC code as a
decision measure. At low SNRs, the estimated value from MM
estimator plays a major role, while the ML estimation i s put
into good use at high SNRs. Moreover, the classical estimators
and their simplified models are given inductively in Section
III.
II. S
YSTEM MODEL
It is assumed that the perfect synchronization has been
achieved and the samples of a coded 𝑀-APSK signal trans-
mitted over additive white Gaussian noise (AWGN) channel
from the matched filter output can be express as
𝑟
𝑘
= ℎ ⋅ 𝑥
𝑘
+ 𝑤
𝑘
𝑘 =1, ⋅⋅⋅𝐾. (1)
where ℎ is a channel gain, 𝑥
𝑘
are transmitted symbols from
the 𝑀 -APSK constellation set, which has been normalized
as 𝐸
∣𝑥
𝑘
∣
2
=1.And𝑤
𝑘
are independent and identically
distributed (i.i.d) samples of white Gaussian random process
with zero mean and variance 𝜎
2
.
978-1-5090-5932-4/17/$31.00 ©2017 IEEE