
梦想飞
- 粉丝: 3
- 资源: 12
最新资源
- 多功能HTML网站模板:手机电脑适配与前端源码
- echarts实战:构建多组与堆叠条形图可视化模板
- openEuler 22.03 LTS专用openssh rpm包安装指南
- H992响应式前端网页模板源码包
- Golang标准库深度解析与实践方案
- C语言版本gRPC框架支持多语言开发教程
- H397响应式前端网站模板源码下载
- 资产配置方案:优化资源与风险管理的关键计划
- PHP宾馆管理系统(毕设)完整项目源码下载
- 中小企业电子发票应用与管理解决方案
- 多设备自适应网页源码模板下载
- 移动端H5模板源码,自适应响应式网页设计
- 探索轻量级可定制软件框架及其Http服务器特性
- Python网站爬虫代码资源压缩包
- iOS App唯一标识符获取方案的策略与实施
- 百度地图SDK2.7开发的找厕所应用源代码分享
资源上传下载、课程学习等过程中有任何疑问或建议,欢迎提出宝贵意见哦~我们会及时处理!
点击此处反馈


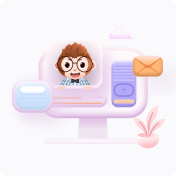