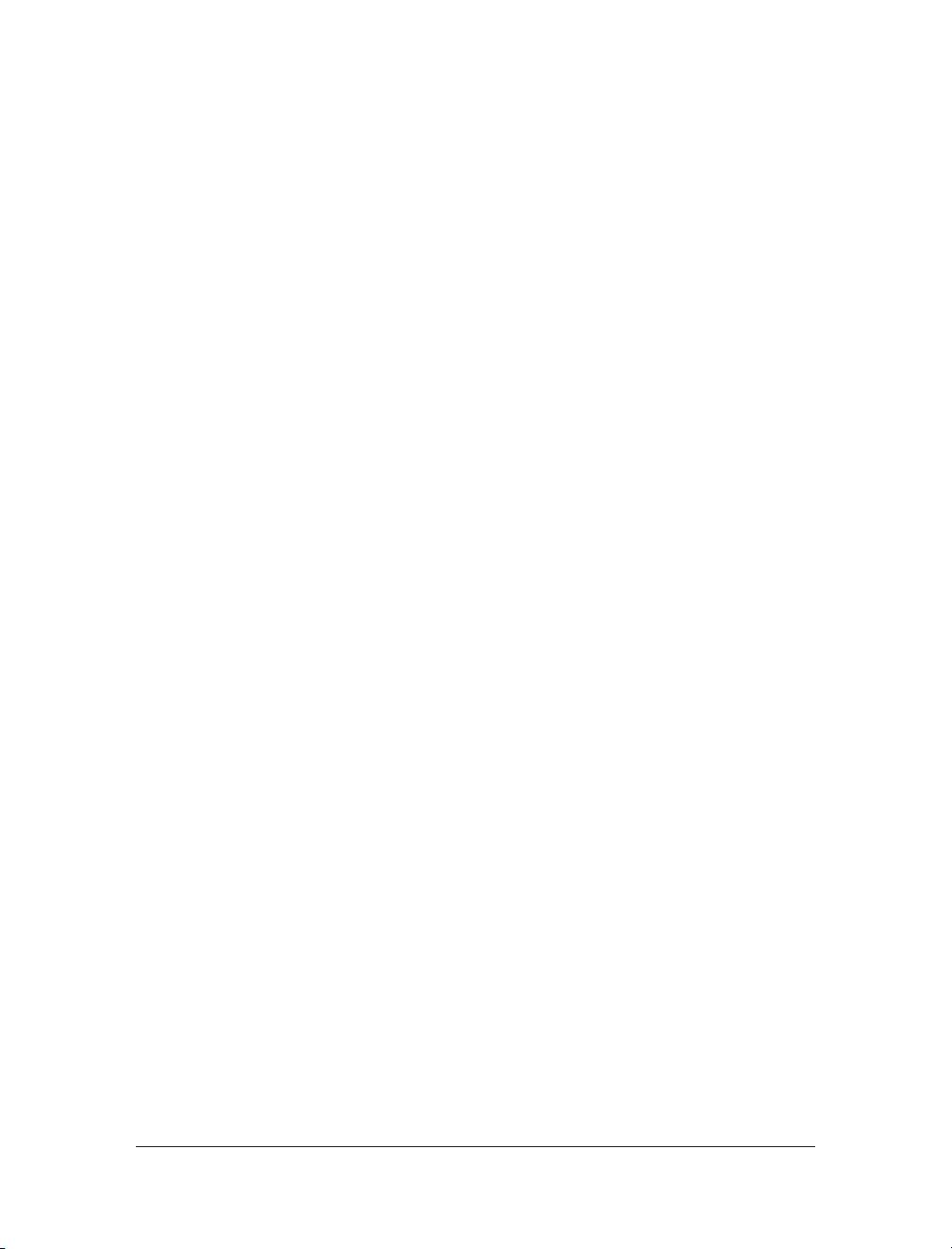
matter how you define data science, you’ll find practitioners for whom the definition
is totally, absolutely wrong.
Nonetheless, we won’t let that stop us from trying. We’ll say that a data scientist is
someone who extracts insights from messy data. Today’s world is full of people trying
to turn data into insight.
For instance, the dating site OkCupid asks its members to answer thousands of ques‐
tions in order to find the most appropriate matches for them. But it also analyzes
these results to figure out innocuous-sounding questions you can ask someone to
find out how likely someone is to sleep with you on the first date.
Facebook asks you to list your hometown and your current location, ostensibly to
make it easier for your friends to find and connect with you. But it also analyzes these
locations to identify global migration patterns and where the fanbases of different
football teams live.
As a large retailer, Target tracks your purchases and interactions, both online and in-
store. And it uses the data to predictively model which of its customers are pregnant,
to better market baby-related purchases to them.
In 2012, the Obama campaign employed dozens of data scientists who data-mined
and experimented their way to identifying voters who needed extra attention, choos‐
ing optimal donor-specific fundraising appeals and programs, and focusing get-out-
the-vote efforts where they were most likely to be useful. It is generally agreed that
these efforts played an important role in the president’s re-election, which means it is
a safe bet that political campaigns of the future will become more and more data-
driven, resulting in a never-ending arms race of data science and data collection.
Now, before you start feeling too jaded: some data scientists also occasionally use
their skills for good—using data to make government more effective, to help the
homeless, and to improve public health. But it certainly won’t hurt your career if you
like figuring out the best way to get people to click on advertisements.
Motivating Hypothetical: DataSciencester
Congratulations! You’ve just been hired to lead the data science efforts at DataScien‐
cester, the social network for data scientists.
Despite being for data scientists, DataSciencester has never actually invested in build‐
ing its own data science practice. (In fairness, DataSciencester has never really inves‐
ted in building its product either.) That will be your job! Throughout the book, we’ll
be learning about data science concepts by solving problems that you encounter at
work. Sometimes we’ll look at data explicitly supplied by users, sometimes we’ll look
at data generated through their interactions with the site, and sometimes we’ll even
look at data from experiments that we’ll design.
2 | Chapter 1: Introduction