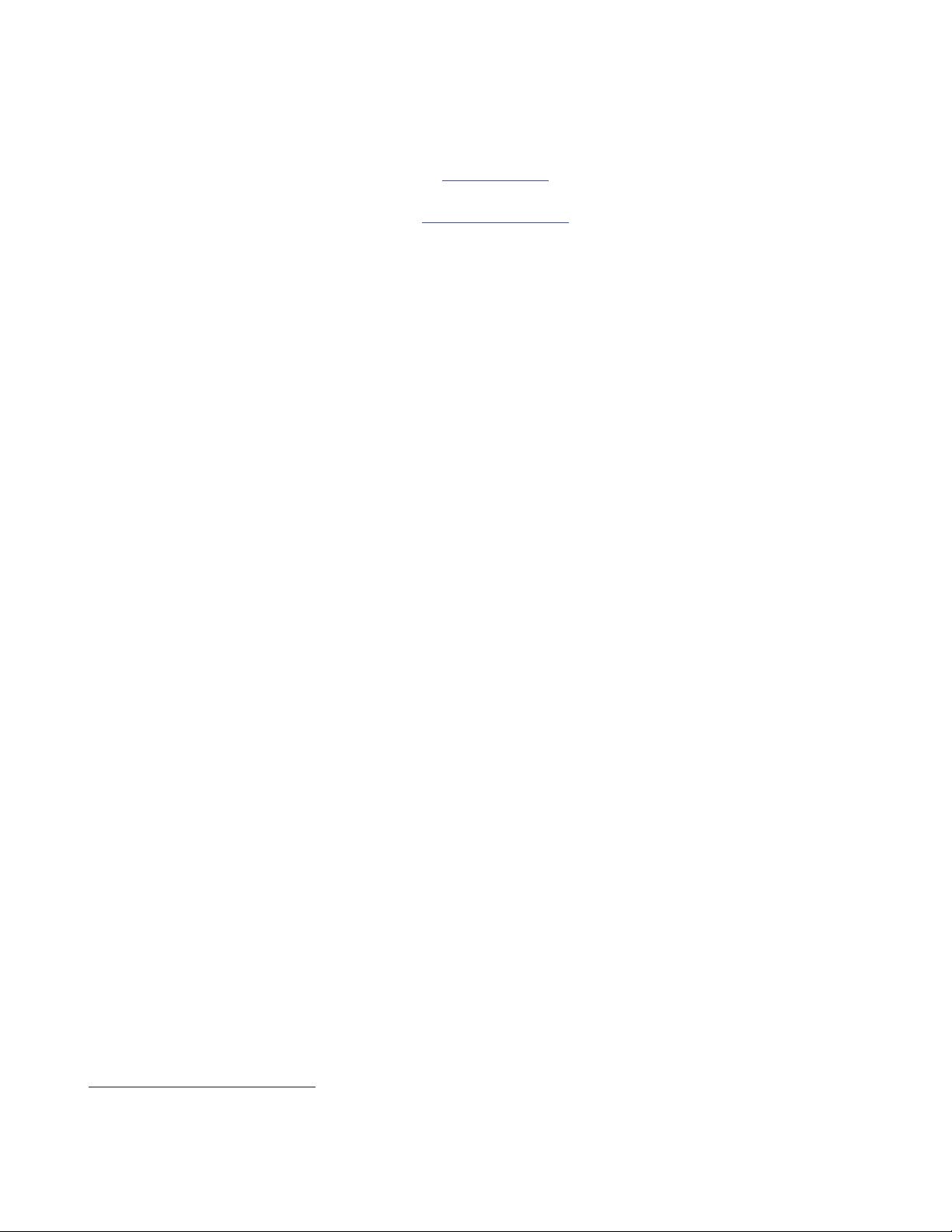
1 Introduction
With the development of science and technology,
some controlled objects we are facing, such as process
industries, navigation and robots, are increasingly
complex due to their strong nonlinear, time-varying,
uncertain dynamics or multiple operating modes. As to
the processes with great jump operating modes, neither
robust control, nor the conventional adaptive control,
which has good performance in controlling the objects
with slowly changing parameters, can effectively handle
the wide range of changing parameters or structures. On
the contrary, by decomposing complex process into a
series of simple models, Multiple model control is a
good scheme to solve this problem, which attracts
increasing interests of researchers. Therefore, K.S.
Narendra called it one of the most promising adaptive
control methods
[1]
.
Formulating model set is one of key tasks in designing
multi-model controller. Generally speaking, there are
two categories of multiple model modeling methods:
online modeling and offline modeling. For the former,
one way is deriving model set by analyzing the
controlled objects’ operating modes or balance points
[2]
.
another way is to formulating model set by clustering
input-output data of the various operating modes and
modeling each clustering data
[3]
. For the latter, the
dynamic model set is acquired by employing online
training algorithms, data analysis technology, as well as
model set storage technology
[4,5]
. With offline modeling
method high modeling accuracy and low real-time
requirements can be archived. Its drawback is, if the data
collected is insufficient or the controlled object is
The project is supported by the National Natural Science Foundation of
hin
11
11
changing, the model set established offline may not
longer meet new requirements. With online modeling
method changes of the controlled object can be easily
traced, but these kind of methods need heavy computing
burden to meet the demands of online control. In
addition, such methods can not necessarily guarantee
complete covering uncertain space by model set.
The creature’s immune system can generate different
antibodies to counter different antigens. The antibody
discovery process is similar to multiple model
identification. In immune system, a hierarchical
identification mechanism is employed to construct the
model set to recognize antigens invaded: firstly dividing
huge uncertain space into small ones by antibody library,
and then, searching highly precise antibodies through
online learning. With this method, not only can antigen
uncertain space be easily covered, but also higher speed
of antibody learning can be obtained. Therefore,
referring to antigen’s recognition mechanism of the
immune system, an online hierarchical multi-model
identification method is presented in this paper.
Simulation results show the method’s effectiveness.
2 Mechanism of Antigen Recognition
Antibodies in creature’s body are secreted by B-cells.
There are a mass of B-cells in immune system, which
come from the multipotential stem cells located in bone
marrow. These B-cells get maturity gradually after
differentiation, then come into the spleen and lymph
nodes, and finally are distributed in the spleen summary,
splenic cord and lymphoid nodule, lymphatic cord and
gastrointestinal submucosal lymph nodules. Under
antigenic stimulus, the B-cells, functioning as humoral
immunity, can differentiate and proliferate into plasma
Hierarchical Multiple Model Identification for Complex Objects
Xu Xuesong
1
Wang Zhonglun
2
1. School of Electrical and Electronic Engineering, ECJTU, Nanchang 330013
E-mail: cedarxu@163.com
2. School of Electrical and Electronic Engineering, ECJTU, Nanchang 330013
E-mail: johnwong1992@163.com
Abstract: In order to solve the modeling problem of complex objects with jump parameters, a hierarchical multiple model
online identification method based on mechanism of antigen identification was proposed. In this paper, through training
the input-output data the model set was acquired to act as a classifier to divide the uncertain space of parameters into
several small subspaces. In these subspaces, the RLS algorithm was employed to identify the precise parameters online.
The algorithm training model set and the procedure of online identification were presented. The method combined the
advantages of prior knowledge and online training. Its simulation on identification for a industry process with jump
operating mode was carried out. Results showed the method has good identification performance for the objects with
jump parameters.
Key Words: Multiple Model Identification; Model Set Training; Mechanism of Immune System
6466
978-1-4799-7016-2/15/$31.00
c
2015 IEEE