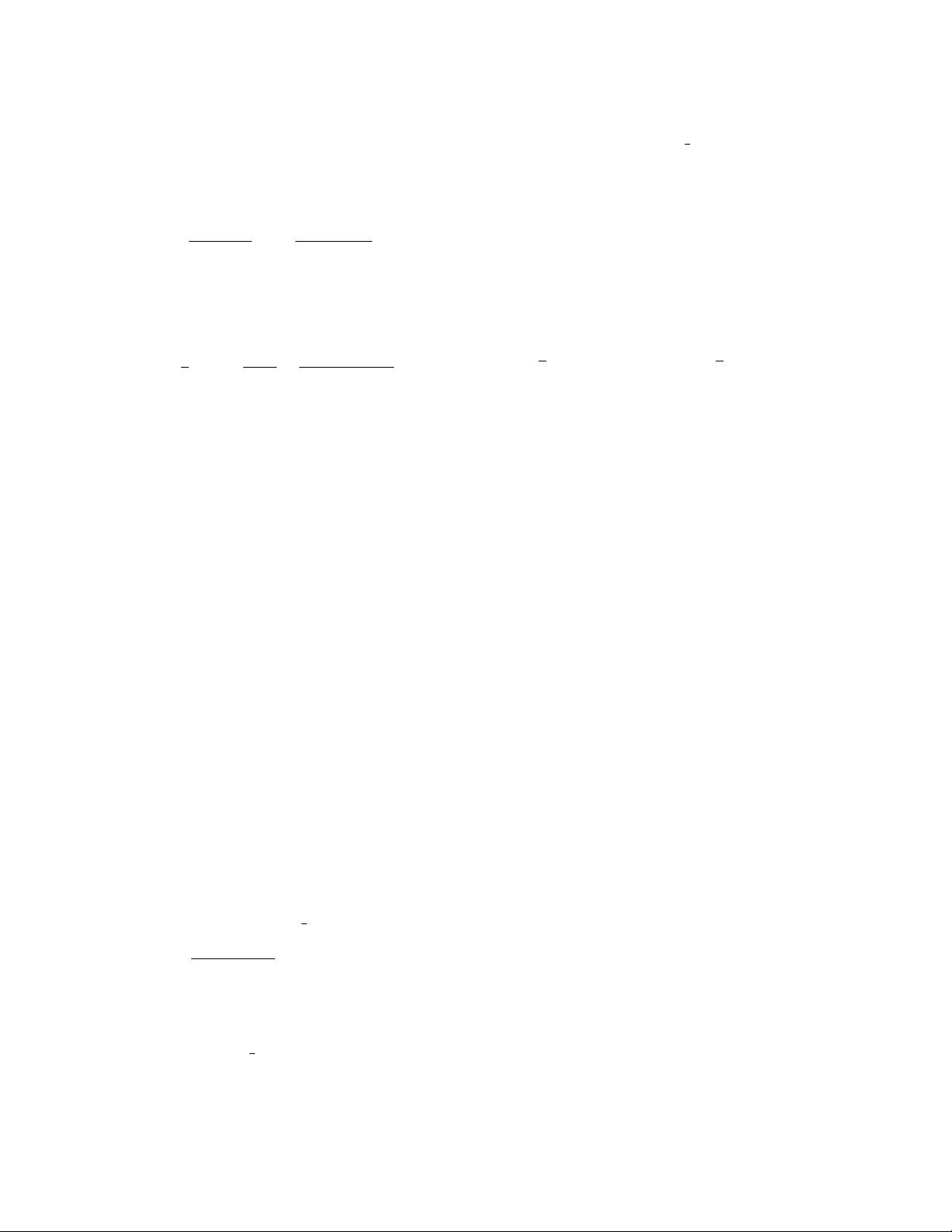
5
Later, we will use the following first-order approximation:
Exp(φ + δφ) ≈ Exp(φ) Exp(J
r
(φ)δφ). (7)
The term J
r
(φ) is the right Jacobian of SO(3) [43, p.40] and
relates additive increments in the tangent space to multiplica-
tive increments applied on the right-hand-side (Fig. 1):
J
r
(φ) = I −
1−cos(kφk)
kφk
2
φ
∧
+
kφk−sin(kφk)
kφ
3
k
(φ
∧
)
2
. (8)
A similar first-order approximation holds for the logarithm:
Log
Exp(φ) Exp(δφ)
≈ φ + J
−1
r
(φ)δφ. (9)
Where the inverse of the right Jacobian is
J
−1
r
(φ) = I +
1
2
φ
∧
+
1
kφk
2
+
1 + cos(kφk)
2kφk sin(kφk)
(φ
∧
)
2
.
The right Jacobian J
r
(φ) and its inverse J
−1
r
(φ) reduce to the
identity matrix for kφk=0.
Another useful property of the exponential map is:
R Exp(φ) R
T
= exp(Rφ
∧
R
T
) = Exp(Rφ) (10)
⇔ Exp(φ) R = R Exp(R
T
φ). (11)
b) Special Euclidean Group: SE(3) describes the group
of rigid motion in 3D, which is the semi-direct product of
SO(3) and R
3
, and it is defined as SE(3)
.
= {(R, p) : R ∈
SO(3), p ∈ R
3
}. Given T
1
, T
2
∈ SE(3), the group operation
is T
1
· T
2
= (R
1
R
2
, p
1
+ R
1
p
2
), and the inverse is T
−1
1
=
(R
T
1
, −R
T
1
p
1
). The exponential map and the logarithm map
for SE(3) are defined in [44]. However, these are not needed
in this paper for reasons that will be clear in Section III-C.
B. Uncertainty Description in SO(3)
A natural definition of uncertainty in SO(3) is to define a
distribution in the tangent space, and then map it to SO(3) via
the exponential map (6) [44, 46, 47]:
˜
R = R Exp(), ∼ N (0, Σ), (12)
where R is a given noise-free rotation (the mean) and is a
small normally distributed perturbation with zero mean and
covariance Σ.
To obtain an explicit expression for the distribution of
˜
R,
we start from the integral of the Gaussian distribution in R
3
:
Z
R
3
p()d =
Z
R
3
αe
−
1
2
kk
2
Σ
d = 1, (13)
where α = 1/
p
(2π)
3
det(Σ) and kk
2
Σ
.
=
T
Σ
−1
is the
squared Mahalanobis distance with covariance Σ. Then, ap-
plying the change of coordinates = Log(R
−1
˜
R) (this is the
inverse of (12) when kk < π), the integral (13) becomes:
Z
SO(3)
β(
˜
R) e
−
1
2
k
Log(R
−1
˜
R)
k
2
Σ
d
˜
R = 1, (14)
where β(
˜
R) is a normalization factor. The normalization factor
assumes the form β(
˜
R) = α/| det(J (
˜
R)|, where J (
˜
R)
.
=
J
r
(Log(R
−1
˜
R)) and J
r
(·) is the right Jacobian (8); J (
˜
R) is a
by-product of the change of variables, see [46] for a derivation.
From the argument of (14) we can directly read our “Gaus-
sian” distribution in SO(3):
p(
˜
R) = β(
˜
R) e
−
1
2
k
Log(R
−1
˜
R)
k
2
Σ
. (15)
For small covariances we can approximate β ' α, as
J
r
(Log(R
−1
˜
R)) is well approximated by the identity matrix
when
˜
R is close to R. Note that (14) already assumes relatively
a small covariance Σ, since it “clips” the probability tails
outside the open ball of radius π (this is due to the re-
parametrization = Log(R
−1
˜
R), which restricts to kk < π).
Approximating β as a constant, the negative log-likelihood of
a rotation R, given a measurement
˜
R distributed as in (15), is:
L(R) =
1
2
Log(R
−1
˜
R)
2
Σ
+const =
1
2
Log(
˜
R
−1
R)
2
Σ
+const,
(16)
which geometrically can be interpreted as the squared angle
(geodesic distance in SO(3)) between
˜
R and R weighted by
the inverse uncertainty Σ
−1
.
C. Gauss-Newton Method on Manifold
A standard Gauss-Newton method in Euclidean space
works by repeatedly optimizing a quadratic approximation
of the (generally non-convex) objective function. Solving the
quadratic approximation reduces to solving a set of linear
equations (normal equations), and the solution of this local
approximation is used to update the current estimate. Here we
recall how to extend this approach to (unconstrained) optimiza-
tion problems whose variables belong to some manifold M.
Let us consider the following optimization problem:
min
x∈M
f(x), (17)
where the variable x belongs to a manifold M; for the sake
of simplicity we consider a single variable in (17), while the
description easily generalizes to multiple variables.
Contrarily to the Euclidean case, one cannot directly ap-
proximate (17) as a quadratic function of x. This is due
to two main reasons. First, working directly on x leads to
an over-parametrization of the problem (e.g., we parametrize
a rotation matrix with 9 elements, while a 3D rotation is
completely defined by a vector in R
3
) and this can make the
normal equations under-determined. Second, the solution of
the resulting approximation does not belong to M in general.
A standard approach for optimization on manifold [45, 48],
consists of defining a retraction R
x
, which is a bijective map
between an element δx of the tangent space (at x) and a
neighborhood of x ∈ M. Using the retraction, we can re-
parametrize our problem as follows:
min
x∈M
f(x) ⇒ min
δx∈R
n
f(R
x
(δx)). (18)
The re-parametrization is usually called lifting [45]. Roughly
speaking, we work in the tangent space defined at the current
estimate, which locally behaves as an Euclidean space. The use
of the retraction allows framing the optimization problem over
an Euclidean space of suitable dimension (e.g., δx ∈ R
3
when
we work in SO(3)). We can now apply standard optimization