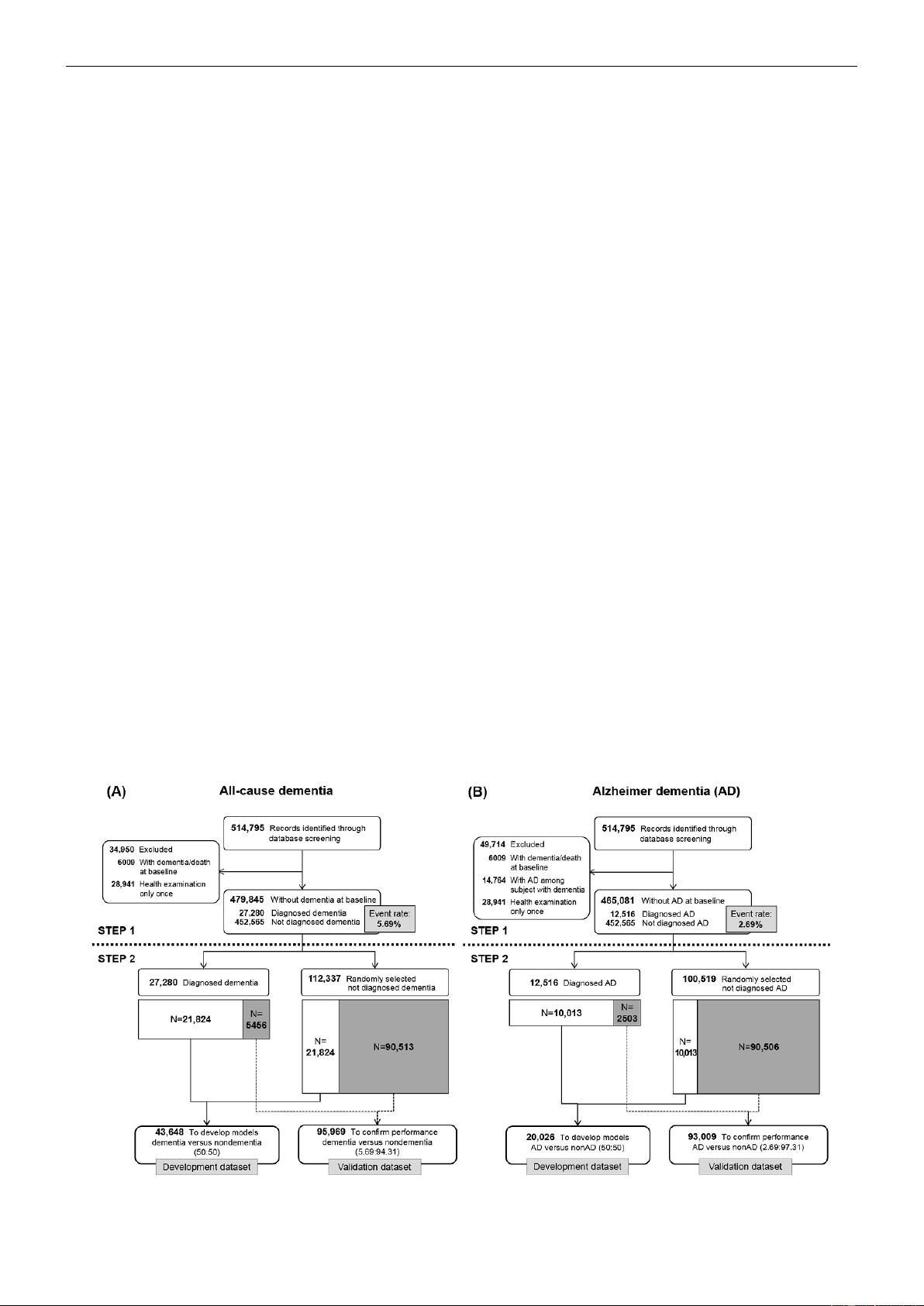
Figure 1 shows the sample selection process in 2 steps (with
all-cause dementia and Alzheimer dementia separately labeled
as A and B). The first step describes the selection of candidate
individuals from the NHIS-HEALS cohort whose data could
be used for predictive modeling. The second step describes the
process of dividing the data into development and validation
datasets for machine learning. The development datasets were
used to fit the parameters of classifiers (ie, criteria that helped
to discriminate individuals who developed dementia during the
study period from those who did not develop dementia) in each
model. The validation datasets were used to assess the
generalization error of the final models.
To create the development and validation datasets for all-cause
dementia and Alzheimer dementia, we first identified 514,795
individuals with records of a health examination in the baseline
year (2002-2003). To analyze all-cause dementia, we excluded
individuals with records of all-cause dementia or death at
baseline and those with no further health examinations after the
baseline year. Of the remaining 479,845 individuals, 27,280
developed all-cause dementia during the study period, resulting
in an event rate of 5.69% (Figure 1). We applied the same
procedure to analyze Alzheimer dementia among 465,081
individuals and found an event rate of 2.69% (Figure 1).
The deep learning method has the advantage that it can identify
patterns in each outcome (eg, yes or no; or event or nonevent).
Deep learning is considered to have high predictive accuracy
in classification studies; however, an extremely imbalanced
dataset can pose a challenge to the detection of patterns in
outcome variables. The fundamental cause of that problem is
that smaller amount of data provides less concrete evidence for
specific patterns than larger amounts of data. Thus, we attempted
to deal with this limitation by generating 1:1 allocation through
undersampling, which has been used in previous studies [24,25].
To build a precise and predictive deep learning model, we used
undersampling to adjust the imbalance between the number of
dementia cases and the number of nondementia cases in the
development datasets, resulting in a more precise and predictive
deep learning model. The numbers of cases in the validation
datasets still reflected the actual event rates in the NHIS-HEALS
cohort.
To finish the construction of the development and validation
datasets for all-cause dementia, we divided the 27,280
individuals who developed all-cause dementia into 2 datasets
with a size ratio of 8:2, corresponding to the development and
validation datasets. The development dataset of 43,648
individuals consisted of 21,824 with dementia (80.00% of
27,280 individuals with dementia) and 21,824 without dementia
as a 1:1 ratio to solve the imbalance problem in classification.
The validation dataset included 5456 individuals who developed
all-cause dementia (20.00% of 27,280 who developed all-cause
dementia) along with 90,513 randomly selected individuals who
did not develop all-cause dementia, for a total of 95,969
individuals. In the development dataset, there were 946 deaths
(4.30%) among the 21,824 individuals who did not develop
all-cause dementia. In the validation dataset, there were 3905
deaths (4.30%) among the 90,513 individuals who did not
develop all-cause dementia. Thus, the event rates of all-cause
dementia in the development and validation datasets were
50.00% and 5.69%, respectively.
We constructed the development and validation datasets for
Alzheimer dementia by the same process. The event rates of
Alzheimer dementia in the development and validation datasets
were 50.00% (n=20,026) and 2.69% (n=93,009), respectively.
Secondary analyses by age group are presented in Multimedia
Appendix 1.
Figure 1. Study design and sample selection. (A) All-cause dementia; (B) Alzheimer dementia.
JMIR Med Inform 2019 | vol. 7 | iss. 3 | e13139 | p. 3http://medinform.jmir.org/2019/3/e13139/
(page number not for citation purposes)
Kim et alJMIR MEDICAL INFORMATICS
XSL
•
FO
RenderX