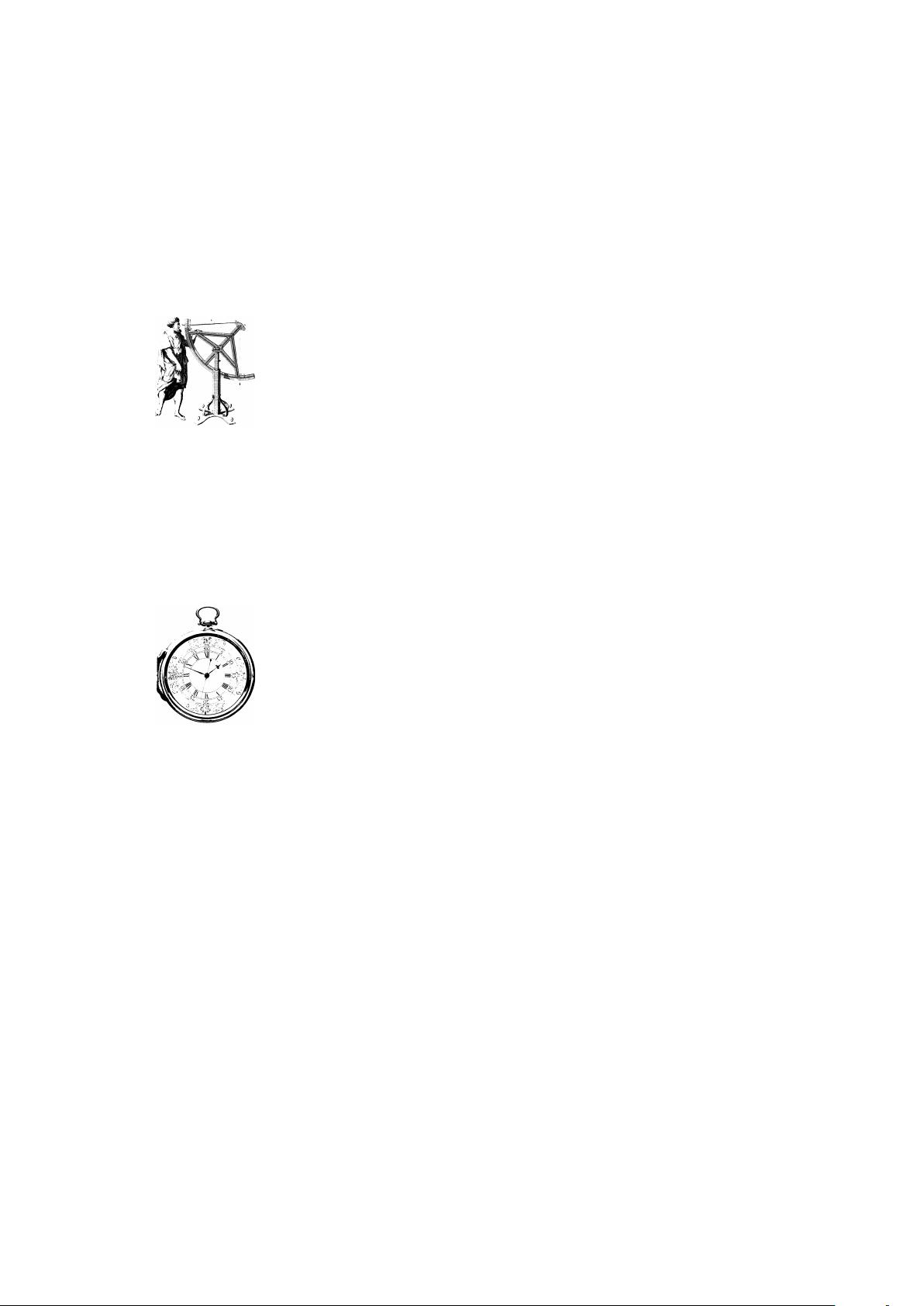
2 Introduction
crude measurements of direction to be made. Together with coarse
nautical charts, the compass made it possible to sail along rhumb lines
between key destinations (i.e., following a compass beari ng) . A seriesFigure 1.1
Quadrant. A tool
used to measure
angles.
of instruments was then gradual ly invented that made it possible to
measure the angle between distant p oi nts (i.e., cross-sta↵, astrolabe ,
quadrant, sextant, theodolite) with increasing accuracy.
These instruments allowed latitude to be determined at sea fairly
readily usi ng celestial navigation. For example, in the Nort h er n Hemi-
sphere, the angle between the North Star, Polaris, and the horizon
provides the latitude. Longitude, however, was a much more difficult
problem. It was known early on that an accurate tim ep ie ce was the
missing piece of the puzzle for the determination of longitude. The be-
haviours of key celestial bodies appear di↵erently at di↵erent locations
on the Earth. Knowing the time of day therefore allows longitude to
be inferred. In 1764, British clockmaker John Harrison buil t the firstFigure 1.2
Harrison’s H4. The
first clock able to
keep accurate time
at sea, enabling
determination of
longitude.
accurate portable timepiec e that e↵ectively solved the longitude prob-
lem; a ship’s longitude could be determined to within about 10 nautical
miles.
Estimation theory also finds its roots in astronomy. The method of
least squares was pionee re d
1
by Gauss, who developed t he t e chnique to
Carl Friedrich
Gauss (1777-1855)
was a German
mathematician
who contributed
significantly to
many fields
including statistics
and estimation.
minimize the impact of measur eme nt error in the prediction of orbits.
Gauss reportedly used least squares to predict the position of t h e dwarf
planet Ceres after passing behind the Sun, accurate to within half a
degree (about nine months after it was last seen). The year was 1801,
and Gauss was 23. Later, in 1809, he proved that the least-squares
method is optimal under the assumption of normally distributed errors.
Most of the classic estimation techniques in use today can be directly
related to Gauss’ least-squares method.
The idea of fitting models to minimize the impact of measurement
error carried forward, but it was not until the middle of the twenti-
eth century that estimation really took o↵. This was likely correlated
with the dawn of the compute r age. In 1960, K alm an pub l is he d two
Rudolf Emil
K´alm´an
(1930-2016) was a
Hungarian-born
American electrical
engineer,
mathematician,
and inventor.
landmark papers that have defined much of what has followed in the
field of state estimation. First, he introduced the notion of observability
(Kalman, 1960a), which tells us when a state can be i n fe r re d from a
set of measurements in a dynamic system. Second, he introduced an
optimal framework for estimating a system’s state in the presence of
measurement noise (Kalman, 1960b); this classic technique for linear
systems (whose measurements are corrupted by Gaussian noise ) is fa-
mously known as the Kalman filter, and has been the workhorse of
estimation for the more than 50 years since its inception. Although
used in many fields, it has been widel y adopted in aerospace appli-
1
There is some debate as to whether Adrien Marie Legendre might have come up with
least squares before Gauss.