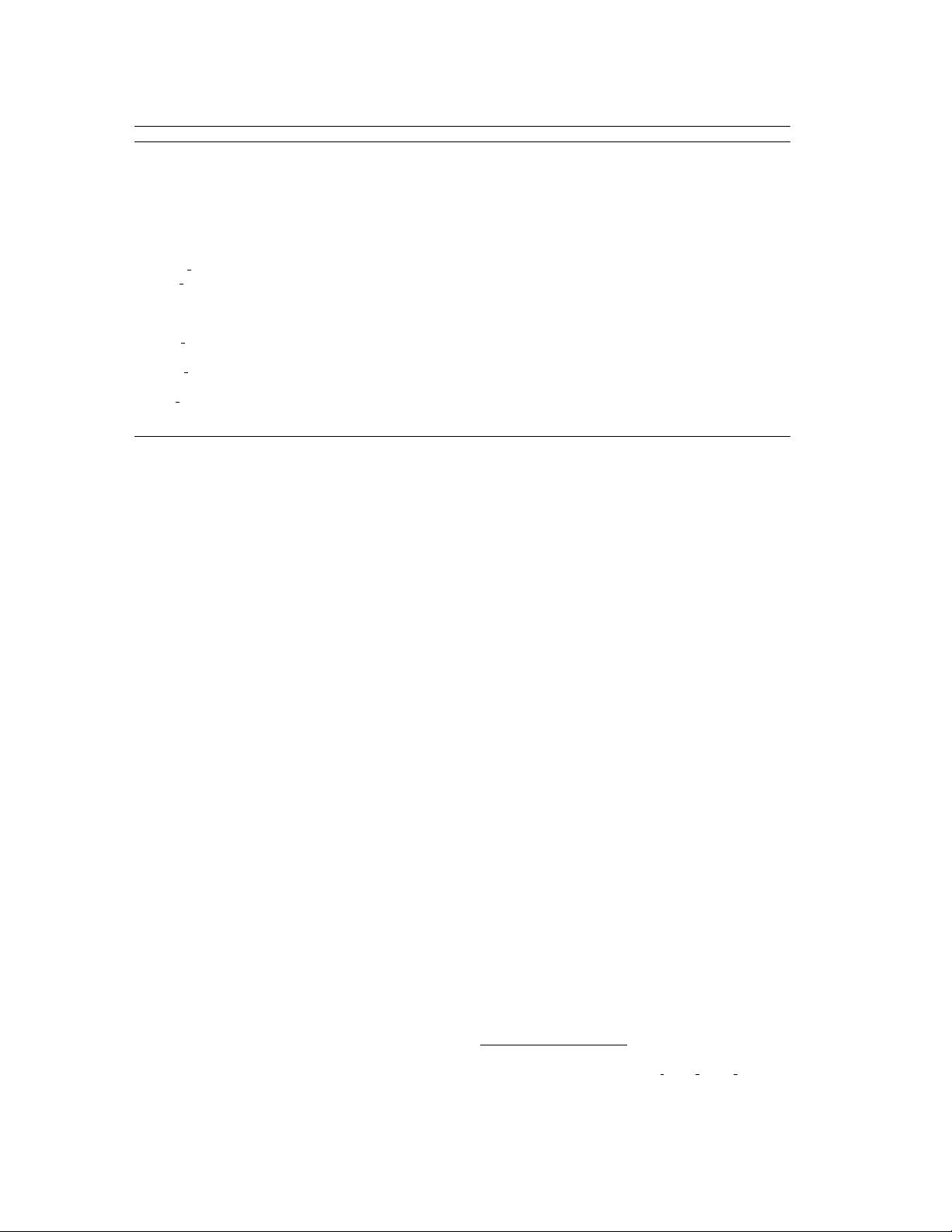
Table 2. Visible and infrared image fusion algorithms that have been integrated in VIFB.
Method Year Journal/Conference Category
ADF [23] 2016 IEEE Sensors Journal Multi-scale
CBF [24] 2015 Signal, image and video processing Multi-scale
CNN [22] 2018 International Journal of Wavelets, Multiresolution and Information Processing DL-based
DLF [21] 2018 International Conference on Pattern Recognition DL-based
FPDE [25] 2017 International Conference on Information Fusion Subspace-based
GFCE [26] 2016 Applied Optics Multi-scale
GFF [27] 2013 IEEE Transactions on Image Processing Multi-scale
GTF [9] 2016 Information Fusion Other
HMSD GF[26] 2016 Applied Optics Multi-scale
Hybrid MSD [28] 2016 Information Fusion Multi-scale
IFEVIP [29] 2017 Infrared Physics & Technology Other
LatLRR [30] 2018 arXiv Saliency-based
MGFF [31] 2019 Circuits, Systems, and Signal Processing Multi-scale
MST SR [32] 2015 Information Fusion Hybrid
MSVD [33] 2011 Defense Science Journal Multi-scale
NSCT SR [32] 2015 Information Fusion Hybrid
ResNet [34] 2019 Infrared Physics & Technology DL-based
RP SR [32] 2015 Information Fusion Hybrid
TIF [35] 2016 Infrared Physics & Technology Saliency-based
VSMWLS [36] 2017 Infrared Physics & Technology Hybrid
2. Related Work
In this section, we briefly review recent visible and in-
frared image fusion algorithms. In addition, we summarize
existing visible and infrared image datasets.
2.1. Visible-infrared fusion methods
A lot of visible and infrared image fusion methods have
been proposed. Before deep learning is introduced to the
image fusion community, main image fusion methods can
be generally grouped into several categories, namely multi-
scale transform-, sparse representation-, subspace-, and
saliency-based methods, hybrid models, and other methods
according to their corresponding theories [37].
In the past few years, a number of image fusion methods
based on deep learning have emerged [38, 39, 40, 37]. Deep
learning can help to solve several important problems in
image fusion. For example, deep learning can provide bet-
ter features compared to handcrafted ones. Besides, deep
learning can learn adaptive weights in image fusion, which
is crucial in many fusion rules. Regarding methods, convo-
lutional neural network (CNN) [41, 4, 5, 2, 8], generative
adversarial networks (GAN) [42], Siamese networks [22],
autoencoder [43] have been explored to conduct image fu-
sion. Apart from image fusion methods, the image quality
assessment, which is critical in image fusion performance
evaluation, has also benefited from deep learning [44]. It is
foreseeable that image fusion technology will develop in the
direction of machine learning, and an increasing number of
research results will appear in the coming years.
2.2. Existing dataset
Although the research on image fusion has begun for
many years, there is still not a well-recognized and com-
monly used dataset in the community of visible and infrared
image fusion. This differs from the visual tracking commu-
nity where several well-known benchmarks have been pro-
posed and widely utilized, such as OTB [45, 46] and VOT
[47]. Therefore, it is common that different image pairs
are utilized in visible and infrared image fusion literature,
which makes the objective comparison difficult.
At the moment, there are several existing visible and
infrared image fusion datasets, including OSU Color-
Thermal Database [48]
2
, TNO Image fusion dataset
3
,
and VLIRVDIF [49]
4
. The main information about these
datasets are summarized in Table 1. Actually, apart from
OSU, the number of image pairs in TNO and VLIRVDIF
is not small. However, the lack of code library, evaluation
metrics as well as results on these datasets make it difficult
to gauge the state-of-the-art based on them.
3. Visible and Infrared Image Fusion Bench-
mark
3.1. Dataset
The dataset in VIFB, which is a test set, includes 21 pairs
of visible and infrared images. The images are collected by
the authors from the Internet
5
and fusion tracking dataset
[62, 48, 13]. These images cover a wide range of environ-
ments and working conditions, such as indoor, outdoor, low
illumination, and over-exposure. Each pair of visible and in-
frared image has been registered to make sure that the image
fusion can be successfully performed. There are various im-
2
http://vcipl-okstate.org/pbvs/bench/
3
https://figshare.com/articles/TN Image Fusion Dataset/1008029
4
http://www02.smt.ufrj.br/ fusion/
5
https://www.ino.ca/en/solutions/video-analytics-dataset/