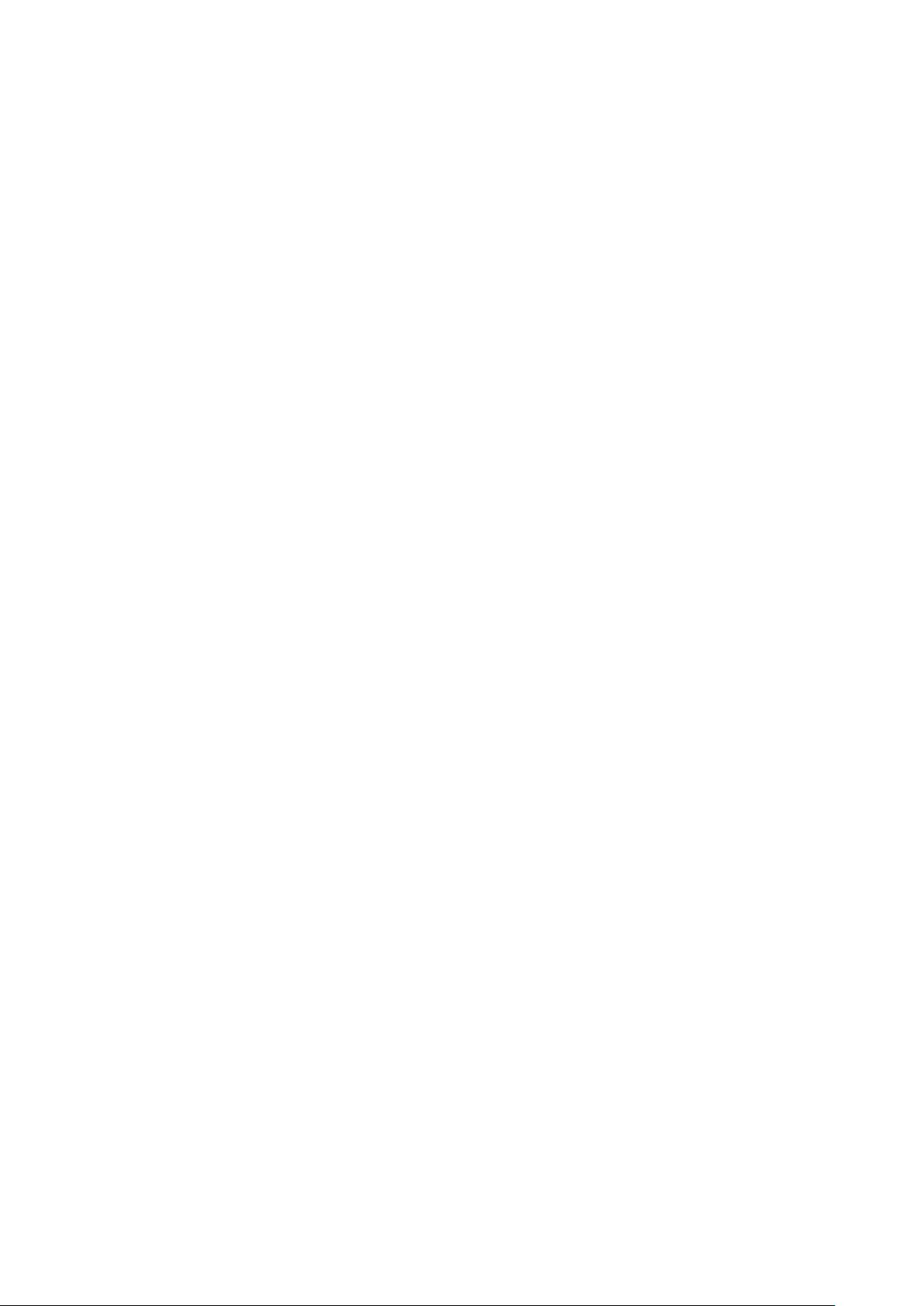
1010 CHINESE OPTICS LETTERS / Vol. 8, No. 10 / Octob er 10, 2010
Sparse Bayesian reconstruction method for multispectral
bioluminescence tomography
Jinchao Feng (冯冯冯金金金超超超)
1
, Kebin Jia (贾贾贾克克克斌斌斌)
1
, Chenghu Qin (秦秦秦承承承虎虎虎)
2
, Shouping Zhu (朱朱朱守守守平平平)
2
,
Xin Yang (杨杨杨 鑫鑫鑫)
2
, and Jie Tian (田田田 捷捷捷)
2,3∗
1
College of Electronic Information and Control Engineering, Beijing University of Technology, Beijing 100124, China
2
Medical Image Pro cessing Group, Institute of Automation, Chinese Academy of Sciences, Beijing 100190, China
3
Life Science Research Center, Xidian University, Xi’an 710071, China
∗
E-mail: tian@ieee.org
Received March 1, 2010
We present a sparse Bayesian reconstruction method based on multiple types of a priori information
for multispectral bioluminescence tomography (BLT). In the Bayesian approach, five kinds of a priori
information are incorp orated, reducing the ill-posedness of BLT. Specifically, source sparsity characteristic
is considered to promote reconstruction results. Considering the computational burden in the multispectral
case, a series of strategies is adopted to improve computational efficiency, such as optimal p ermissible
source region strategy and node model of the finite element method. The performance of the proposed
algorithm is validated by a heterogeneous three-dimensional (3D) micron scale computed tomography atlas
and a mouse-shaped phantom. Reconstructed results demonstrate the feasibility and effectiveness of the
prop osed algorithm.
OCIS co des: 100.3010, 100.3190, 170.3010, 170.6960.
doi: 10.3788/COL20100810.1010.
The emergence of new imaging modalities and ap-
proaches that provide cellular and molecular informa-
tion has led to the nascent field of molecular imag-
ing. Methods based on detecting optical signals in a
small animal have been leading this field
[1,2]
. The light-
emitting enzymes, and the technologies for detecting
their weak bioluminescent signals with a highly sensi-
tive charge-coupled device (CCD) camera in living sub-
jects, comprise the method called in vivo bioluminescence
imaging
[3]
.
Much effort has been devoted to transforming biolumi-
nescence imaging from a planar imaging technique into a
truly three-dimensional (3D) tomographic modality ap-
plication to small animals, because planar bioluminescent
imaging cannot provide depth information
[3]
. The reso-
lution of planar bioluminescent imaging is also limited
due to the nature of surface imaging. Therefore, biolu-
minescence tomography (BLT) is developed to explore
depth information and enhance bioluminescence imaging
resolution. As a result, BLT is particularly attractive for
in vivo applications.
Generally, BLT is an ill-posed problem and the unique-
ness of the BLT solution has been proven theoretically
[4]
.
The uniqueness theory is not only instructional in recon-
structing a bioluminescent source distribution, but also
presents that the ill-posedness of BLT could be overcome
with sufficient a priori information.
Since the concept of BLT was introduced, it has
made great progress in modeling and reconstruction
algorithms
[5−11]
. Currently, BLT reconstruction-based
spectrum information has gained much attention as
BLT reconstruction results have improved, with hyper-
spectral and multisp ectral methods
[5−8]
. In addition, the
importance of multiple types of a priori information has
been recognized. The more the a priori information one
applies, the better the results obtained.
The Bayesian approach provides a framework to in-
corporate multiple types of a priori information. We
have proposed a BLT reconstruction algorithm based on
the Bayesian approach to reconstruct a bioluminescent
source, in which a generalized adaptive Gaussian Markov
random field (GGMRF) prior model for unknown source
density estimation is presented
[9]
. However, the sparse
nature of the internal source to promote reconstructed
quality is not considered in the prior model
[10]
. Further-
more, the permissible source region used in the previous
algorithm is inferred through the light p ower distribu-
tion on the surface, which is hardly determined when
the underlying source is located in a deep position. The
large-scale reconstruction data also significantly affect
reconstructed efficiency in the multispectral case.
In this letter, we describe a sparse Bayesian approach
for multispectral BLT. Furthermore, multiple types of
a priori information are incorp orated into the Bayesian
framework to reduce the ill-posedness of the BLT prob-
lem, specifically the sparsity of source and the optimal
permissible source region.
In bioluminescence imaging, photon propagation can
be described by the steady-state diffusion equation and
Robin boundary condition. Taking into account the in-
fluence of light wavelength λ on tissue optical property,
the following model is thus given:
−∇ · [D(r, λ)∇Φ(r, λ)] + µ
a
(r, λ)Φ(r, λ)
= x(r, λ) (r ∈ Ω), (1)
Φ(r, λ) + 2 A(r; n, n
0
)D(r, λ)[ν(r)
·∇Φ(r, λ)] = 0 (r ∈ ∂Ω), (2)
where Ω is a bounded smooth domain; ∂Ω is the cor-
responding boundary; Φ(r,λ) denotes the photon flux
1671-7694/2010/101010-05
c
° 2010 Chinese Optics Letters