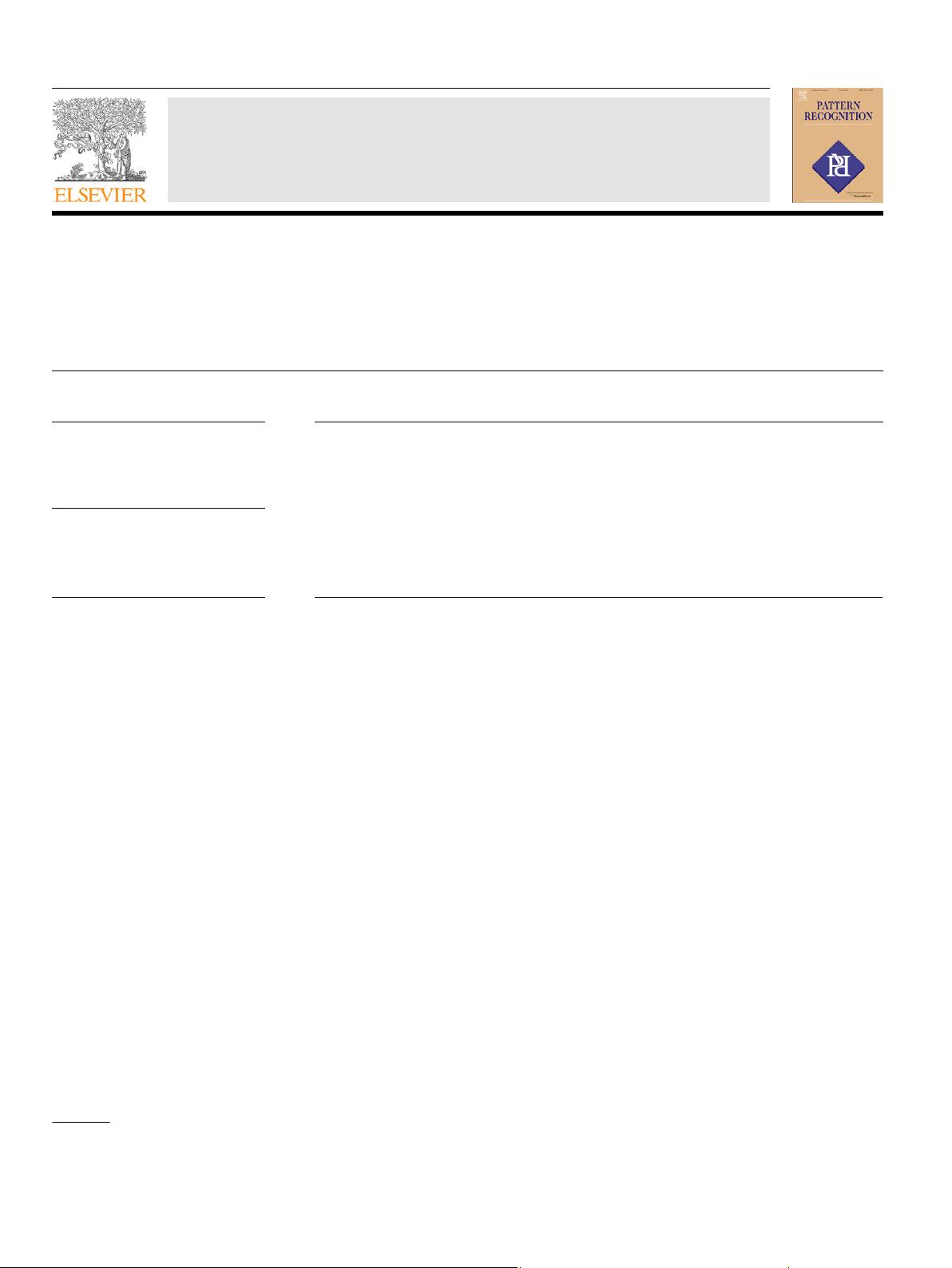
Perceptually motivated morphological strategies for shape retrieval
Rong-Xiang Hu
a,b
, Wei Jia
a,
n
, Yang Zhao
a,b
, Jie Gui
a
a
Hefei Institutes of Physical Science, CAS, P.O. Box 1130, Hefei 230031, China
b
Department of Automation, University of Science and Technology of China, Hefei 230027, China
article info
Article history:
Received 22 June 2011
Received in revised form
21 February 2012
Accepted 23 February 2012
Available online 5 March 2012
Keywords:
Shape retrieval
Shape contexts
Morphological operation
Perceptual custom
abstract
In this paper, two perceptually motivated morphological strategies (PMMS) are proposed to enhance
the retrieval performance of common shape matching methods. Firstly, two human perception customs
are introduced, which have important relations to shape retrieval. Secondly, these two customs are
properly modeled by morphological operations. Finally, the proposed PMMS is applied to improv e the
retrieval performances of a popular shape matching method named Inner-Distance Shape Contexts
(IDSC), and then the Locally Constrained Diffusion Process (LCDP) method is exploited to further
enhance the retrieval performance. This combination achieves a retrieval rate of 98.56% on MPEG-7
dataset. We also conduct the experiments on Swedish Leaf dataset, the ETH-80 dataset and the Natural
Silhouette dataset . The experimental results obtained from four datasets demonstrate clearly the
effectiveness of the proposed method.
& 2012 Elsevier Ltd. All rights reserved.
1. Introduction
Shape is one of the most important features of an object.
It plays a key role in human perception, and has been widely
exploited for many computer vision applications such as object
classification, object recognition and object retrieval. Generally, in
a shape-based object retrieval application, given a query object,
the most similar objects will be retrieved from a dataset accord-
ing to certain similarity or distance measures, which are gener-
ated by shape matching algorithms. In the past decade, many
shape matching algorithms have been proposed [1–10]. Designing
a suitable shape matching method for robust shape retrieval,
however, is a very difficult task. Some researchers therefore start
to explore new perspectives to enhance the retrieval performances.
Recently, many novel shape retrieval methods providing new
perspectives have been proposed [11–19], and have achieved
promising retrieval performances. Here, for convenience’s sake,
we call them as enhancing methods. Further, they could be divided
into three categories, i.e., context-based, knowledge-based and
fusion-based.
Context-based methods are the most widely studied enhan-
cing methods so far. Traditionally, in a common shape matching
method, matching a pair of shapes would generate a similarity or
distance measure, which has no relationship with other shapes.
Recently, it is believed that all shapes should be considered as a
group rather than pairs of shapes in shape retrieval. Precisely,
when the target similarity or distance measure is computed
between one pair of shapes, all similarity or distance measures
between other shapes within the group could provide useful and
complementary information to further correct the target measure.
In this way, more satisfactory retrieval results could be obtained.
Here, the similarity or distance measures between other shapes
within the group are regarded as the context of the target
measure. Thus, those methods based on this strategy are called
as context-based methods. Yang et al. [11,15] proposed a method
to improve the shape retrieval performance through graph
transduction by propagating the model through all existing
shapes. They took advantage of the manifold formed by the
existing shapes, and used an unsupervised graph transduction
approach to learn a better measure for retrieval. Kontschieder
et al. [12] proposed a modified mutual K nearest neighbor graph
to consider the underlying structure of the shape manifold.
The manifold is estimated from the shape similarity measures
between all the shapes within a dataset. Yang et al. [13] later
proposed the method of Locally Constrained Diffusion Process
(LCDP) to better enhance the retrieval performance, in which the
influence of other shapes is propagated as a diffusion process on a
graph formed by the given set of shapes. However, the classical
diffusion process is unstable for sparse space, so they add some
local constraints using K nearest neighbor graph to achieve a
more robust diffusion process. Egozi et al. [16] proposed a meta-
shape-similarity approach to characterize a given shape by its
similarity to its K nearest neighbors. This approach does not
propagate similarities, but aims to compare local graph structures
as intrinsic similarity measures. Recently, Yang et al. [19]
proposed a novel affinity learning method using Tensor Product
Contents lists available at SciVerse ScienceDirect
journal homepage: www.elsevier.com/locate/pr
Pattern Recognition
0031-3203/$ - see front matter & 2012 Elsevier Ltd. All rights reserved.
doi:10.1016/j.patcog.2012.02.020
n
Corresponding author. Tel./fax: þ 86 5515591108.
E-mail address: icg.jiawei@gmail.com (W. Jia).
Pattern Recognition 45 (2012) 3222–3230