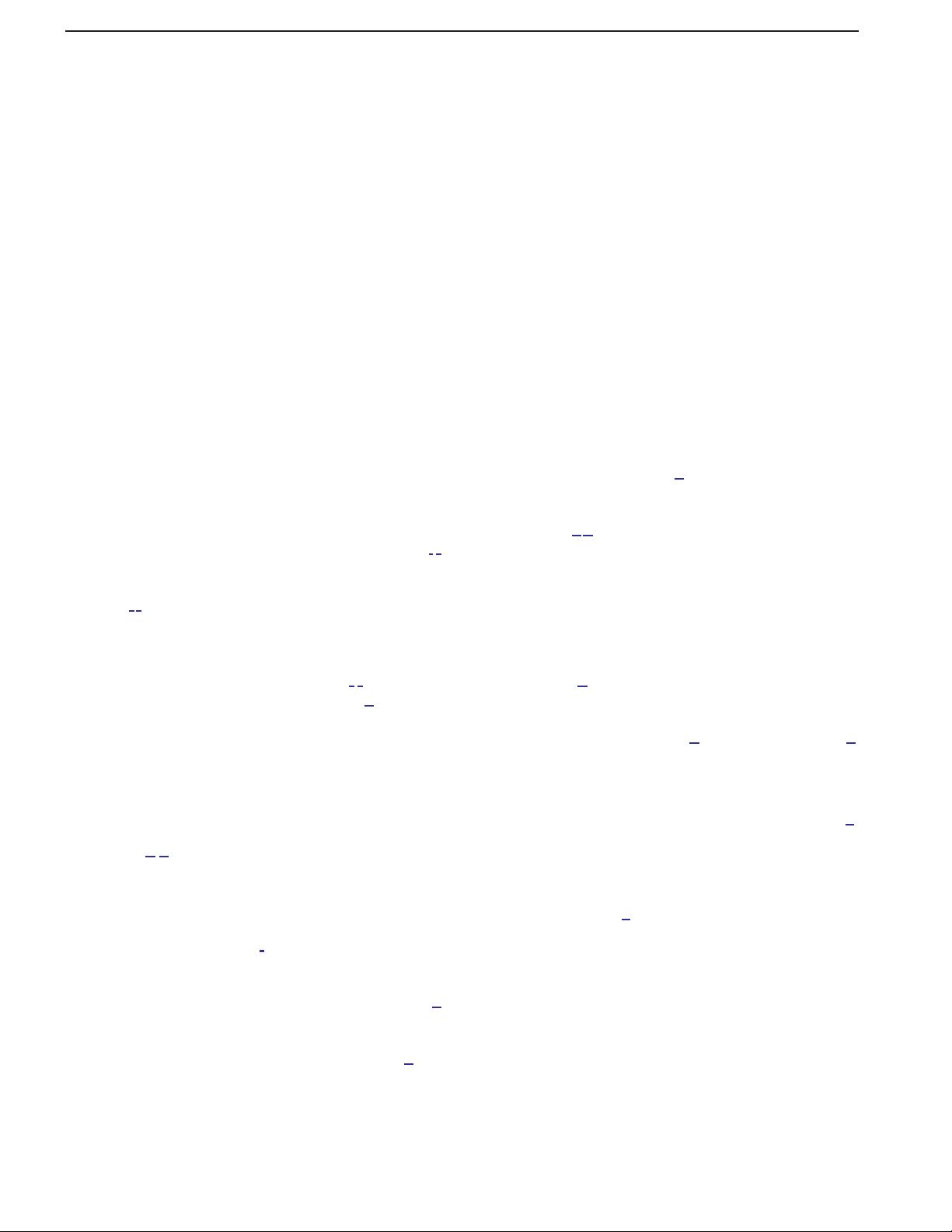
Experimental demonstration of 60 Gb/s optical OFDM
transmissions at 1550 nm over 100 m OM1 MMF IMDD
system with central launching
Jian Chen (陈 健), Ling Fang (方 玲), Qianwu Z hang (张倩武)*, Junjie Zhang (张俊杰),
Yingxiong Song (宋英雄), Yingchun Li (李迎春), and Min Wang ( 汪 敏)
Key Laboratory of Specialty Fiber Optics and Optical Access Networks, Shanghai University, Shanghai 200072, China
*Corresponding author: zhangqianwu@shu.edu.cn
Received September 6, 2016; accepted March 9, 2017; posted online April 10, 2017
Record-high 60 Gb/s optical orthogonal frequency division multiplexing (OFDM) transmissions over intensity
modulation and direct-detection (IMDD)-based 100 m optical mode (OM1) multi-mode fiber (MMF) links are
experimentally demonstrated, utilizing 10 GHz electro-absorption modulated laser intensity modulators at a
single 1550 nm wavelength. Adaptive bit loading and a simple central launching scheme of the proposed scheme
show an effective way for combating the channel fading and simplifying the system structure. It shows good
potential in short reach data center interconnections.
OCIS codes: 060.2330, 060.3510, 060.0060, 060.4080.
doi: 10.3788/COL201715.060603.
Multi-mode fibers (MMFs) have been widely used in
broadband local area networks (LANs) to provide cost-
effective transmissions due to their large core diameters,
which usually enable significant cost reduction in compo-
nent manufacturing, installation, and maintenance
[l–3]
.
In addition, the low cost, high capacity, and short reach
MMF transmission systems attract more and more
attention
[4,5]
due to the rapid growth of bandwidth inten-
sive applications, like interconnections in datacenters
and cloud computing. However, some disadvantages, such
as narrow modulation bandwidth and variations in trans-
mission system spectral characteristics
[6–9]
, limit the
practical implementation of MMF systems
[10]
. Intensity
modulation and direct-detection (IMDD) optical ortho-
gonal frequency division multiplexing (OFDM) is a
promising candidate for the abovementioned high capac-
ity MMF transmission systems because of its unique
capability, such as excellent resistance to a large amount
of differential mode delay (DMD), strong resilience to
fiber dispersion, and great potential for cost-effective
deployment
[11–17]
. Making use of adaptive subcarrier bit
and subcarrier/sub-band power loading, 20.125 Gb/s
real-time dual-band optical OFDM transmissions over
100 m optical mode (OM2)-only MMF systems and
20 Gb/s over 100 m OM1-only MMFs are experimentally
demonstrated by Salas et al.
[6]
. The 25.25 Gb/s real-time
end-to-end optical OFDM transmissions over an IMDD-
based 300 m OM2 MMF link are experimentally demon-
strated with tri-sub-band transceiver architecture
[18]
.
Mode-division multiplexing of a 30 Gb/s 2 × 2 direct-de-
tection OFDM transmission over a 200 m OM3 conven-
tional MMF link is demonstrated by Luo et al.
[19]
.Xu
et al. experimentally investigated a 25.5 Gb/s 2 × 2 mode
group diversity multiplexing (MGDM) OFDM transmis-
sion over a 200 m OM1 MMF with a 4.25 GHz modulation
bandwidth for each channel
[20]
. In add ition, some out-
standing works on >100 Gb∕s optical OFDM [or discrete
multi-tone (DMT)] in single-mode fiber (SMF) links have
been reported
[21,22]
. However, such transmission capacity
achievement in MMF systems is much lower than in
the SMF system.
In this Letter, record-high 60 Gb/s optical OFDM
transmissions over IMDD-based 100 m OM1 MMF links
are experimentally demonstrated, utilizing a 10 GHz
electro-absorption modulated laser (EML) intensity mod-
ulators at a single 1550 nm wavelength. Adaptive subcar-
rier bit loading
[23]
is also employed to combat the channel
fading, which is mainly caused by intermodal dispersion,
mode noise, and bandwidth limit of optoelectronic (O-E)
devices. Both central launching
[24]
and offset launching
[25]
conditions are considered during measurements, and good
potential in short reach data center interconnections
is shown.
Our experimental setup is shown schematically in Fig.
1,
the proposed 60 Gb/s OFDM transmission system in
which all digital signal processing (DSP) procedures for
both transmitter and receiver are realized by the offline
approach. Detailed transceiver and system key parameters
can be found in Table
1. At the transmitter side, the input
pseudo random data (PRBS15) is first mapped into par-
allel complex data by using a four quaternatry amplitude
modulation (4-QAM), 16-QAM, 32-QAM, or 64-QAM
enco der. A 64 point inverse fast Fourier transform (IFFT)
module is then used for the generation of OFDM time-do-
main symbols, where 31 of them can be used to allocate
user data in order to satisfy the Hermitian symmetry
for a real-valued IMDD OOFDM system. A cyclic prefix
(CP) of 16 samples is inserted into each OFDM symbol to
avoid inter-symbol interference (ISI) during transmission.
Each frame is composed of 100 OFDM symbols, where the
COL 15 (6), 060603(2017) CHINESE OPTICS LETTERS June 10, 2017
1671-7694/2017/060603(4) 060603-1 © 2017 Chinese Optics Letters