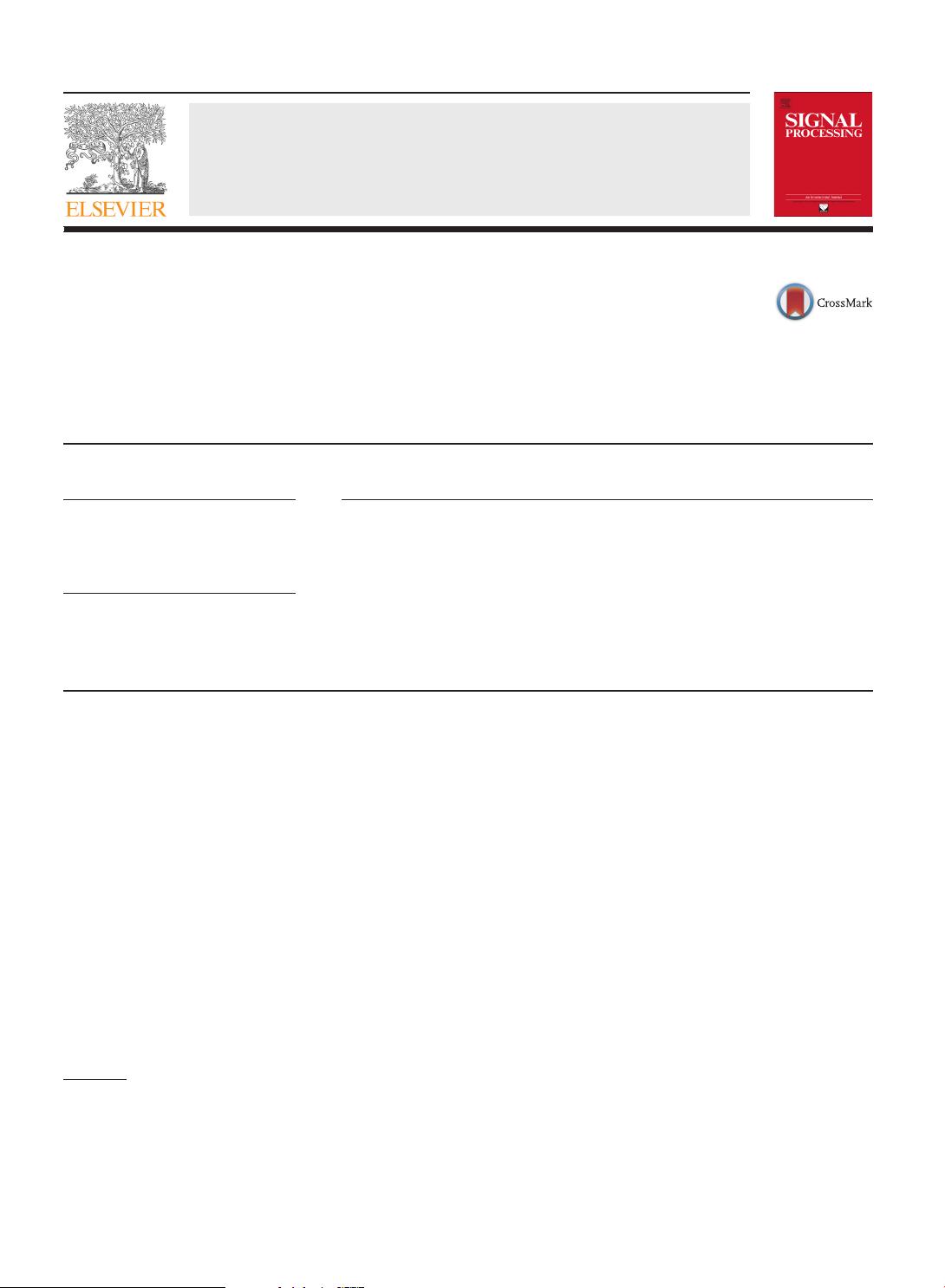
Unbiased estimation of Markov jump systems
with distributed delays
Shuping He
a,b,
n
, Jun Song
a
, Fei Liu
b
a
School of Electrical Engineering and Automation, Anhui University, Hefei 230601, PR China
b
Key Laboratory of Advanced Process Control for Light Industry (Ministry of Education), Institute of Automation, Jiangnan University,
Wuxi 214122, PR China
article info
Article history:
Received 17 April 2013
Received in revised form
29 October 2013
Accepted 29 December 2013
Available online 8 January 2014
Keywords:
Markov jump systems (MJSs)
Unbiased H
1
filtering
Distributed time-delays
Linear matrix inequality
abstract
The unbiased H
1
filtering problem is considered for a class of Markov jump systems
(MJSs) with distributed time-delays. Based on the selected Lyapunov–Krasovskii func-
tional, it gives a sufficient condi tion for the existence of the mode-dependent unbiased
H
1
filter such that the filtering error dynamic MJSs is stochastically stable and satisfies a
prescribed level of H
1
disturbance attenuation in an infinite time-interval. The design
criterions are presented in the form of linear matrix inequality techniques, and then are
described as the optimization problems. At last, two numerical examples are employed to
illustrate the effectiveness of the developed techniques.
& 2014 Elsevier B.V. All rights reserved.
1. Introduction
In the past several decades, dynamical systems with
distributed time-delays have received considerate atten-
tions. Many efforts have been made to study the stability
and control problems of this kind of systems. Among these
results, an important and popular approach is to construct
a Lyapunov–Krasovskii function for the distributed time-
delays. Then by using the bonding techniques to the cross-
term, delay-dependent or delay-independent criteria are
obtained. Comparing with the delay-independent criteria,
the delay-dependent ones are less conservative conditions
for the stability analysis and controller synthesis. And the
measuring index is the maximum allowable upper bound
in the delays, for instance [1–6]. But for filtering schemes,
the conservative conditions are seldom considered. The
filtering problem is to estimate the unavailable state
variables of a known system by using past measurement.
During the past several years, H
1
filtering problems have
been investigated extensively. By comparison with the
traditional Kalman filtering scheme [7], the noise sources
in the H
1
setting are arbitrary signals with bounded
energy or average power, and no exact statistical proper-
ties are required to be known. For more results on this
topic, readers are referred to Refs. [8–12]. It should point
out that the dimensions of the filtering error system,
which is constructed by the dynamics and the filter, are
twice that of the dynamics. This may bring the computa-
tional complexity, especially in practical engineering pro-
cess where the number of states is numerous. For these,
the so-called unbiased conditions [13] can provide a good
help. Under this condition, the dimension of the unbiased
filtering error dynamics equals to that of the dynamic
system. Moreover, no restriction is imposed on the stabi-
lity of original dynamics while taking into account the
unbiased condition. So this filtering approach has exten-
sive application in unstable systems. For more results on
this topic, we refer readers to [14–19] and the references
therein.
In general, the parameters of many dynamical systems
are subject to random abrupt changes due to, for example,
sudden environment changes, subsystem switching, system
Contents lists available at ScienceDirect
journal ho mepage: www.elsevier.com/locate/sigpro
Signal Processing
0165-1684/$ - see front matter & 2014 Elsevier B.V. All rights reserved.
http://dx.doi.org/10.1016/j.sigpro.2013.12.030
n
Corresponding author at: School of Electrical Engineering and
Automation, Anhui University, Hefei 230601, PR China.
Tel./fax: þ86 551 63861413.
E-mail addresses: shuping.he@ahu.edu.cn (S. He),
sjky5211@163.com (J. Song), fliu@jiangnan.edu.cn (F. Liu).
Signal Processing 100 (2014) 85–92