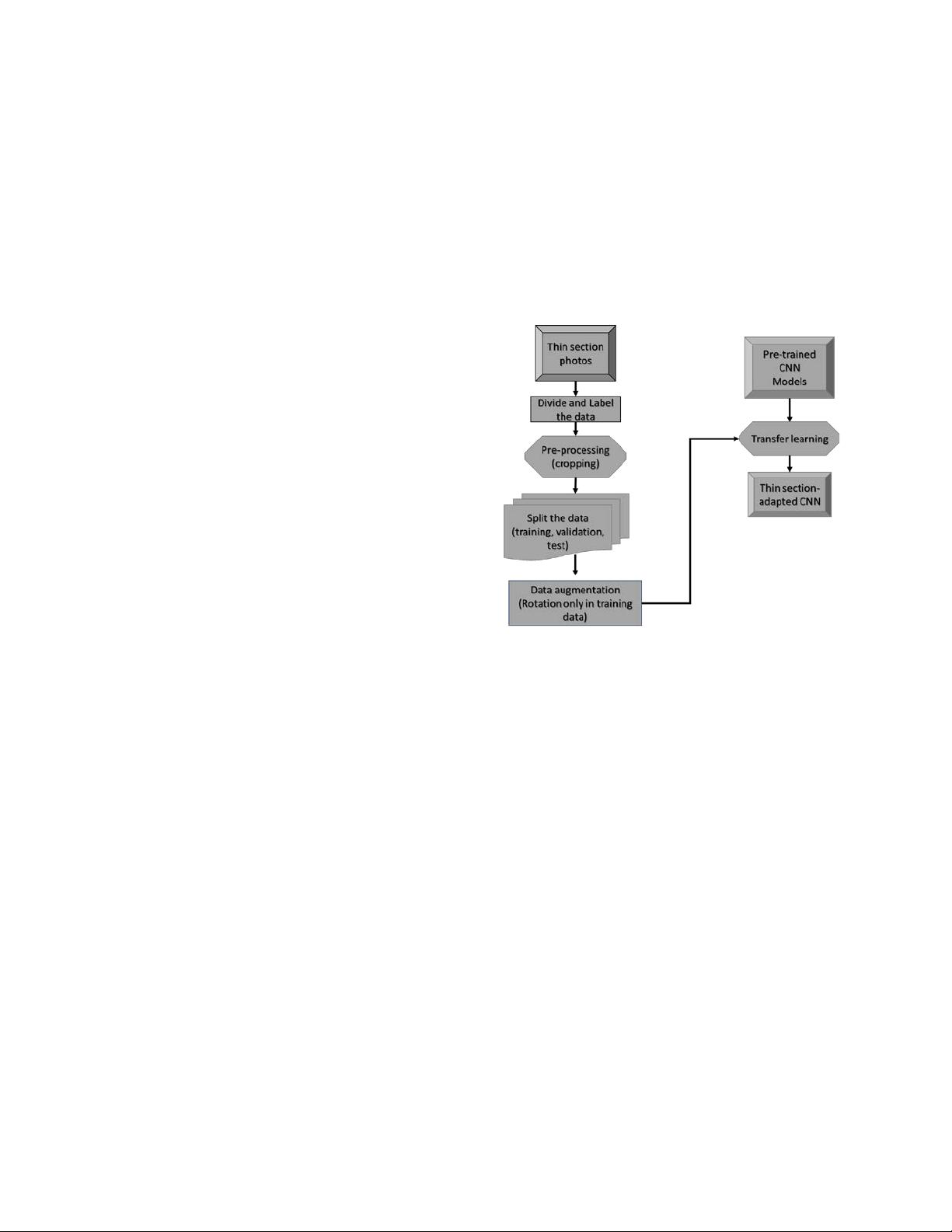
Deep convolutional neural networks as an estimator of porosity in thin-section images for
unconventional reservoirs.
David Duarte-Coronado, Jerson Tellez-Rodriguez*, Rafael Pires de Lima, Kurt Marfurt and Roger Slatt. The
University of Oklahoma, School of geology and geophysics
Summary
Reservoir characterization of unconventional reservoirs is
challenging and highly time-consuming due to the detail
needed to identify key features when evaluating the rocks.
When using conventional methods to estimate porosity,
there are always limitations related to the resolution of the
tools to measure these parameters. This study proposes an
innovative technique to estimate porosity in thin section
images from the Mississippian strata in the Anadarko basin,
Oklahoma (USA). The method is developed to be applied to
other unconventional reservoirs where petrophysical
parameters such as porosity and permeability are important
to improve definition and prediction of reservoir quality.
Introduction
Analysis of petrographic thin-sections focuses on the
microscopic description and classification of rocks. The goal
is to describe the characteristics of the rock such as grain
size, structure, mineralogical, textural composition, cement
and porosity.
One of the most important uses of petrographic studies is to
define and characterize porosity in microfacies based on the
colored space observed within thin sections. Geologist can
characterize not only qualitative but also quantitively the
porous space within a thin section to estimate reservoir
quality related to the defined microfacies. However,
hundreds of thin sections must be evaluated trough a point
count process to classify and characterize microfacies and
estimate porosity values. We take advantage of the
technology advances in computer vision to automatize this
time-consuming process considering information generated
using traditional petrographic analysis and the values
obtained from core plug data. Furthermore, new thin sections
data will always be produced, thus the management of large
number of samples is an urgent problem for the geoscientist
community (Cheng et al., 2018). We find that convolutional
neural networks (CNN) are reliable models that can be used
to obtain accurate results in significantly less time. The
technique we present can greatly contribute to fast-paced
decision making required for development of
unconventional reservoirs in the oil and gas industry.
Methods
The methodology for this study is summarized in Figure 1.
We used petrographic thin-section slides to describe and
characterize microfacies and porosity. These thin sections
were stained to highlight calcite (pink) and porous space
(blue) that is a standard procedure in petrographic studies.
Figure 1. Workflow for the data preparation used in this study.
Then, we obtained high-resolution microscope photos for
every described thin section. In addition, we use information
collected from multiple core plugs that have lab porosity
measurements data (ambient porosity) and their
corresponding thin-sections. Using this data set three groups
were generated to describe and define quality of porosity
within the samples from the training data, 1) lower (less than
3%), 2) medium (3 – 5 %), and 3) high (> 5%). We made the
groups considering the number of total samples in the dataset
to obtain approximately an even number of samples within
each of the groups. The evaluated samples for training data
were taken only from the massive siltstone microfacies.
Bearing in mind that grain size plays a crucial role in the
petrographic analysis we selected only images with 10x
objective zoom to be part of the study. To compensate for
the different size and increase the number of images used as
input data resolution of most CNN models trained on the
ILSVRC, the annual ImageNet Large Scale Visual
Recognition Challenge (Russakovsky et al. 2015) dataset
(usually ranging from 200 by 200 to 300 by 300 pixels) we
resample the original thin-section photographs (1292 by 968
pixels) into sets of six smaller overlapping squared smaller
images (644 by 644 pixels, Figure 2).
10.1190/segam2019-3216898.1
Page 3181
SEG International Exposition and 89th Annual Meeting
Downloaded 08/01/22 to 111.42.93.151. Redistribution subject to SEG license or copyright; see Terms of Use at http://library.seg.org/page/policies/terms
DOI:10.1190/segam2019-3216898.1