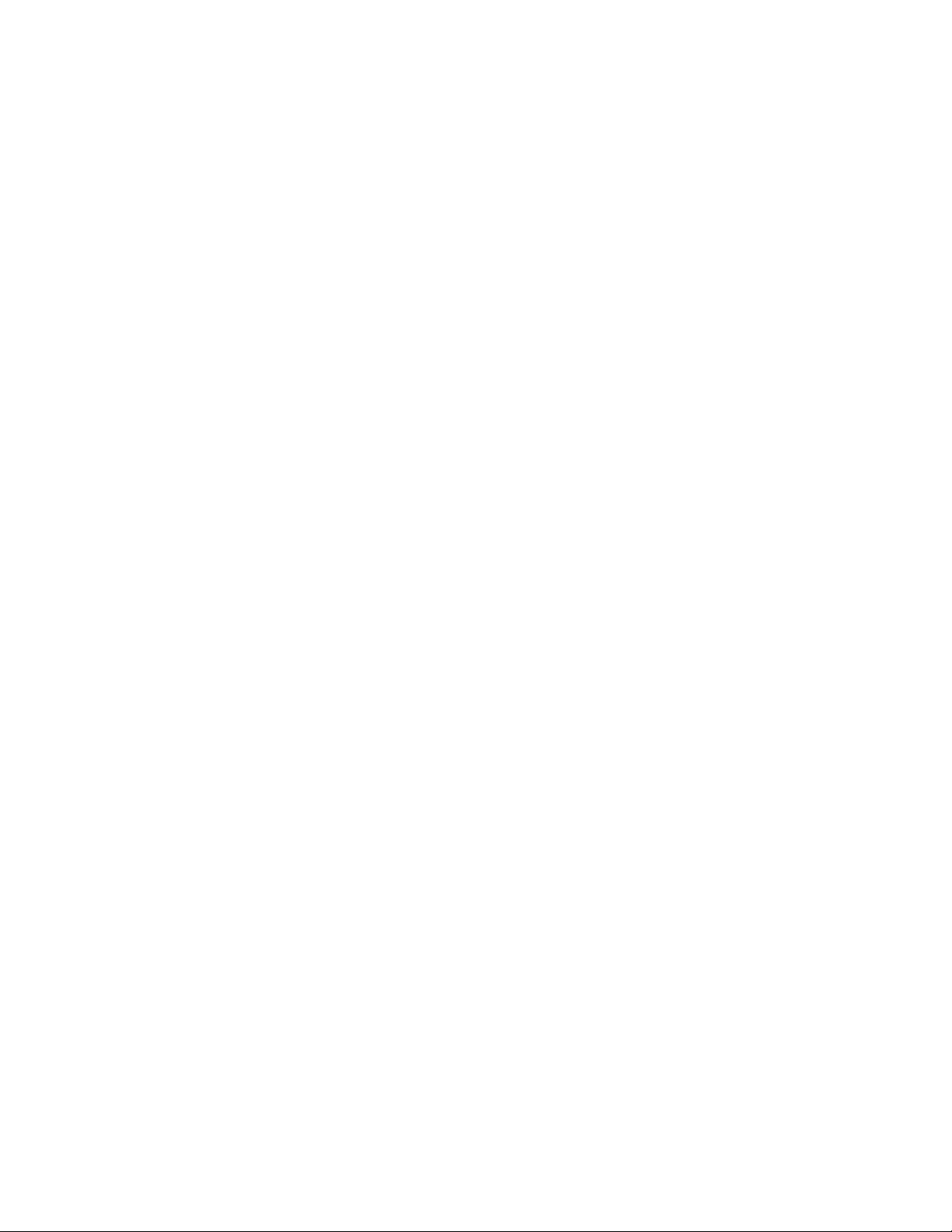
Support vector machines (SVMs) have proved to be a good alternative compared to other machine
learning techniques specically for classication problems. However just like articial neural networks
(ANN), SVMs are also black box in nature because of its inability to explain the knowledge learnt in
the process of training, which is very crucial in some applications like medical diagnosis, security and
bankruptcy prediction etc. In this chapter a novel hybrid approach for fuzzy rule extraction based on
SVM is proposed. This approach handles rule-extraction as a learning task, which proceeds in two major
steps. In the rst step the authors use labeled training patterns to build an SVM model, which in turn
yields the support vectors. In the second step extracted support vectors are used as input patterns to fuzzy
rule based systems (FRBS) to generate fuzzy “if-then” rules. To study the effectiveness and validity of
the extracted fuzzy rules, the hybrid SVM+FRBS is compared with other classication techniques like
decision tree (DT), radial basis function network (RBF) and adaptive network based fuzzy inference
system. To illustrate the effectiveness of the hybrid developed, the authors applied it to solve a bank
bankruptcy prediction problem. The dataset used pertain to Spanish, Turkish and US banks. The quality
of the extracted fuzzy rules is evaluated in terms of delity, coverage and comprehensibility.
Chapter 20
Data Mining Experiences in Steel Industry ........................................................................................ 427
Joaquín Ordieres-Meré, Universidad Politécnica de Madrid, Spain
Ana González-Marcos, Universidad de La Rioja, Spain
Manuel Castejón-Limas, Universidad de León, Spain
Francisco J. Martínez-de-Pisón, Universidad de La Rioja, Spain
This chapter reports ve experiences in successfully applying different data mining techniques in a hot-
dip galvanizing line. Engineers working in steelmaking have traditionally built mathematical models
either for their processes or products using classical techniques. Their need to continuously cut costs
down while increasing productivity and product quality is now pushing the industry into using data
mining techniques so as to gain deeper insights into their manufacturing processes. The authors’ work
was aimed at extracting hidden knowledge from massive data bases in order to improve the existing
control systems. The results obtained, though small at rst glance, lead to huge savings at such high
volume production environment. The effective solutions provided by the use of data mining techniques
along these projects encourages the authors to continue applying this data driven approach to frequent
hard-to-solve problems in the steel industry.
Chapter 21
Application of Neural Networks in Animal Science ........................................................................... 440
Emilio Soria, Valencia University, Spain
Carlos Fernández, Valencia Polytechnic University, Spain
Antonio J. Serrano, Valencia University, Spain
Marcelino Martínez, Valencia University, Spain
José R. Magdalena, Valencia University, Spain
Juan F. Guerrero, Valencia University, Spain
Stock breeding has been one of the most important sources of food and labour throughout human his-
tory. Every advance in this eld has always led to important and benecial impacts on human society.