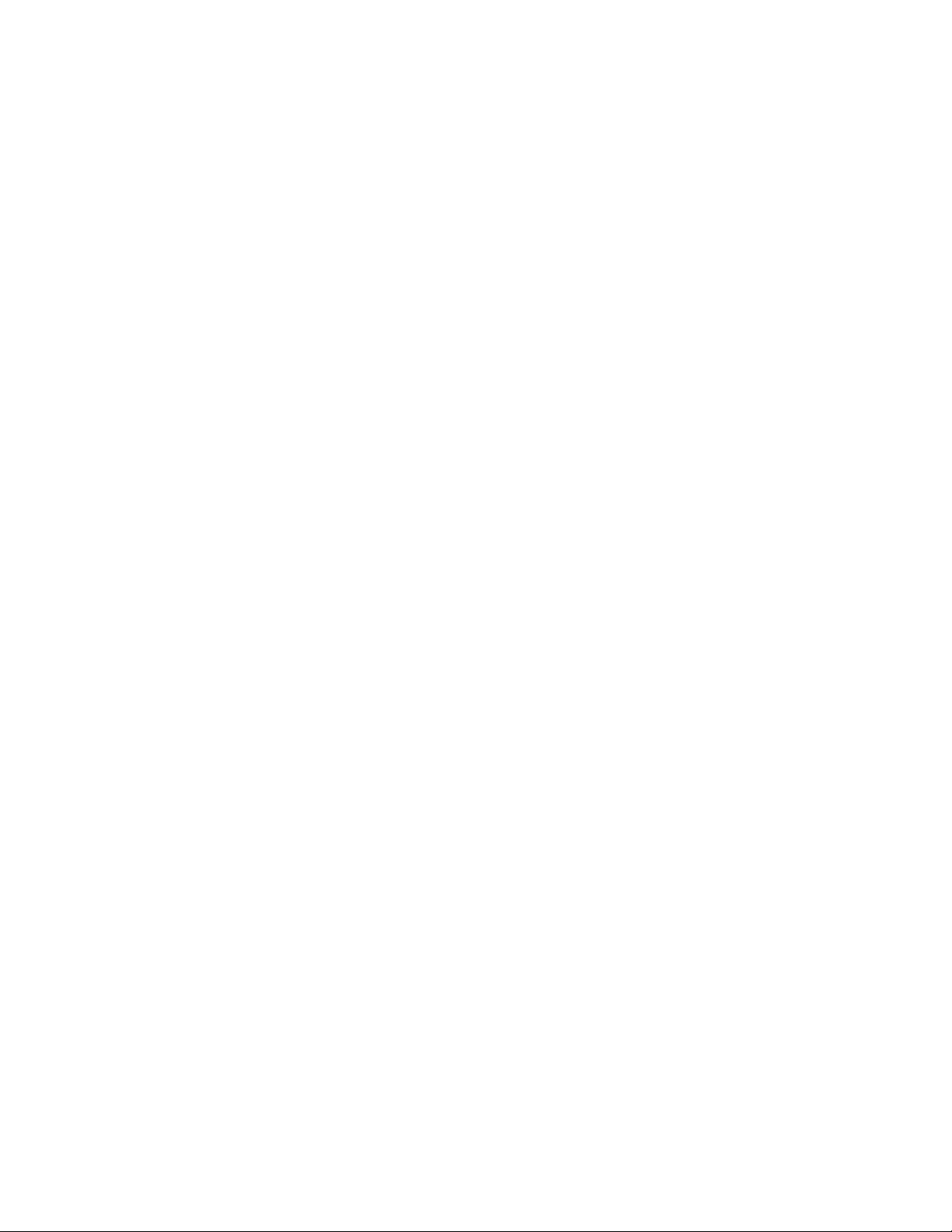
2 MMWR / September 26, 2014 / Vol. 63 / No. 3
Supplement
persons were assumed to eventually become symptomatic.
Data from reports of previous Ebola outbreaks were used to
model the daily change of patients’ status between the disease
states. For example, a probability distribution to characterize
the likelihood of incubating a given number of days was built
using previously published data (4). The resulting distribution
has a mean incubation period of approximately 6 days and a
99th percentile of 21 days (Appendix [Figure 4]). Based on
previous studies, patients were assumed to be infectious for a
period of 6 days (3,5).
Patients were categorized into three levels: 1) hospitalized
in an Ebola treatment unit (ETU) or medical care facility,
2) home or in a community setting such that there is a reduced
risk for disease transmission (including safe burial when
needed), and 3) home with no effective isolation (Appendix
[Figure 5]). Hospitalized refers to facilities such as ETUs where
medical care is provided. Ideally, such facilities have infection-
control protocols that prevent additional disease transmission.
However, this is not always the case. Therefore, the average
daily risk for transmission is greater than zero in these facilities
(i.e., transmission does occur), but the risk is fewer than one
person infected per infectious patient (Appendix [Table 1]).
The risk for onward disease transmission by patient category
was calculated (Appendix [Table 1]). The ability to add
imported cases (whole numbers) every 10 days (approximately
the sum of the average incubation and infectiousness periods)
was built into the EbolaResponse modeling tool (Appendix
[Table 2]). Imported cases represent either cases in persons who
travel into the community undetected from other outbreak-
affected areas or persons who might represent previously
undetected chains of transmission. To estimate the daily
number of beds in use (i.e., beds in medical care facilities, such
as ETUs), previously published data were adapted to provide
both the likelihood of a patient going to an ETU and the
number of days that a patient in each patient category would
spend in the hospital (6) (Appendix [Table 3]).
Substantial underreporting of cases might be occurring both
in Liberia and Sierra Leone (7). To correct for underreporting,
EbolaResponse was used to predict the number of beds in use
on August 28, 2014. This number was then compared with the
actual number of beds in use (from expert opinion estimates).
The difference between the two estimates is the potential
underreporting correction factor of 2.5 (Appendix [Table 4]).
Accuracy of the estimates produced by EbolaResponse was
assessed by comparing the model-predicted number of cases
to the reported cases (Appendix [Figure 6, Table 5]). The
difference in estimates calculated using the uncorrected data
and the estimates using the data corrected for underreporting
reflects the potential range of uncertainty regarding the actual
number of cases that might occur.
Accuracy of the model forecasts was tested by comparing the
latest available reported cases at time of writing to estimated
cases (estimated using uncorrected data). The last date of
reported data used to fit the model was August 28, 2014, for
Sierra Leone and August 29, 2014, for Liberia. Reported cases
were compared with estimated cases as of September 9, 2014,
for Liberia and September 13, 2014, for Sierra Leone.
In the absence of a universal preventive intervention (e.g.,
vaccine), control of the epidemic consists of having as many
patients as possible receiving care in hospitals or, when ETUs
are at capacity, at home or in a community setting such that
there is a reduced risk for disease transmission (including safe
burial when needed). Suitable methods to enhance protection
and minimize disease transmission are under development. To
illustrate how increasing the percentage of patients in these
two categories can control and eventually end the epidemic in
Liberia, the first scenario was created. Starting on August 24,
2014, and for the following 30 days, the percentage of patients
in ETUs was increased from 10% of all patients to 17%. In
the subsequent 30 days (starting September 23, 2014), that
percentage was increased to 25% and left at that level for the
remainder of the period covered by the model (Appendix
[Figure 7, Figure 8]). Starting on August 24, 2014, and for the
following 30 days, the percentage of patients at home or in a
community setting such that there is a reduced risk for disease
transmission was increased from 8% of all patients to 20%.
Additional increases were included so that by December 22,
2014, a total of 70% of patients were in either one of the
two patient settings (25% in ETUs and 45% at home or in a
community setting such that there is a reduced risk for disease
transmission) (Appendix [Figure 8]).
To illustrate the cost of delay, in terms of additional cases and
the resulting need for additional resources to end the epidemic, in
starting to increase interventions that can control and eventually
stop the epidemic, a second separate control-and-stop scenario
was first constructed as follows. Starting on September 23, 2014,
and for the next 30 days, the percentage of all patients in ETUs
was increased from 10% to 13%. This percentage was again
increased on October 23, 2014, to 25%, on November 22, 2014,
to 40%, and finally on December 22, 2014, to 70% (Appendix
[Figure 9]) (i.e., it takes 90 days for the percentage of patients in
ETUs to reach 70% of all patients). The percentage of patients
at home or in a community setting such that there is a reduced
risk for disease transmission was kept at 8% from September 23,
2014, through the remainder of the period covered by the model.
The impact of delay of starting the increase in interventions was
then estimated by twice repeating the above scenario but setting
the start day on either October 23, 2014, or November 22, 2014.