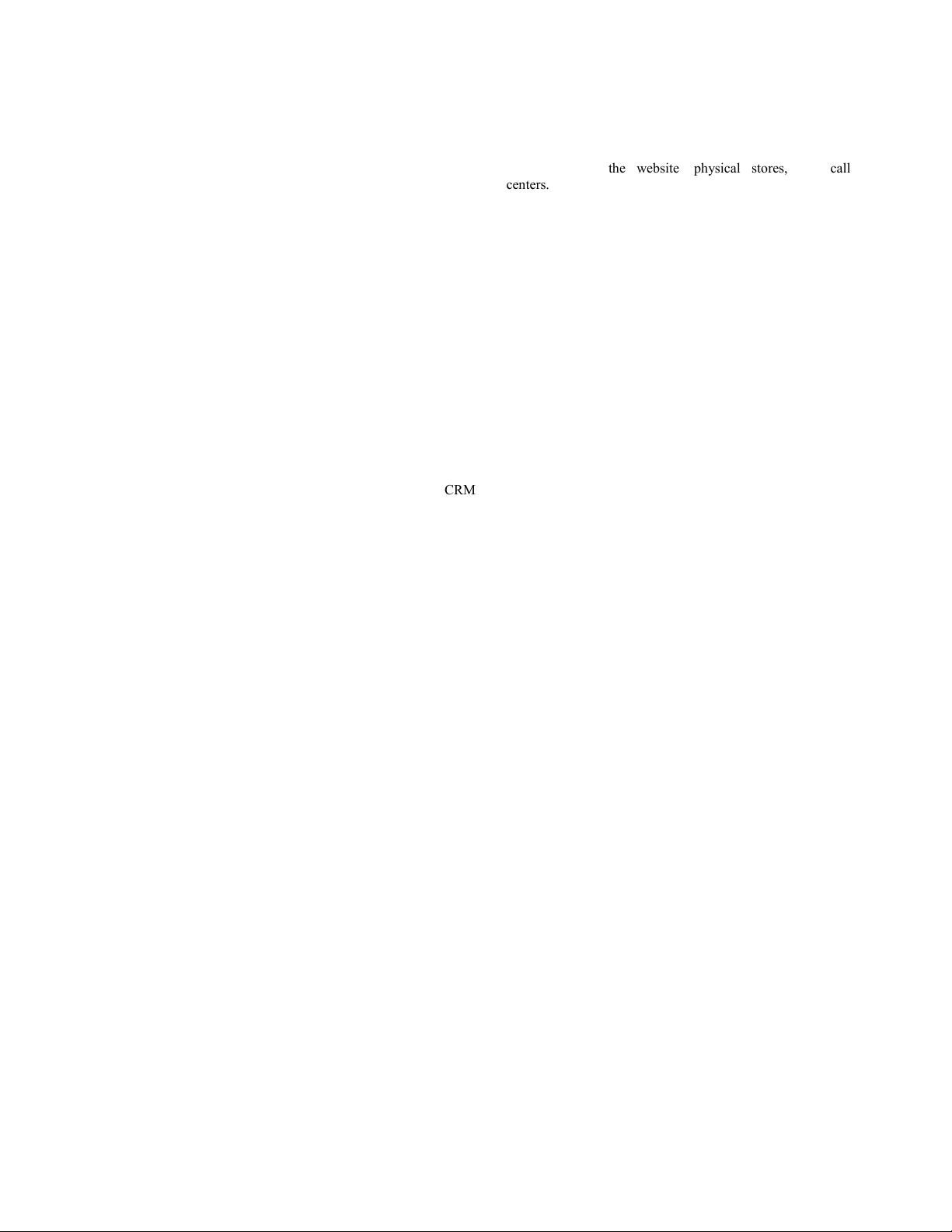
relationship activities. In a follow-up meeting, the CIO
suggested that as an immediate measure to boost sales in the
next quarter, they should utilize internal factors to identify
and predict service cancellation based on the history of a
customer’s communication with the company. This would
enable NHT to design effective strategies to curb the
customer churn.
The CIO further argued that the rich sets of
communication between the customers and CRM department
could help identify behavioral patterns that led to churn. The
CRM department communicated with the customer through
multiple channels and in different formats. These multiple
means of communication presented data management
challenges to the churn analysis phase. The challenges posed
are discussed in the next section.
The executives agreed that exploring churn analysis from
the perspective of the customer relations department was key
to reducing cancellations. This was because many of the
cancellations involved a series of back-and-forth
communications with the customer service department.
Hence, the NHT executives set up a task force that reported
directly to the CIO to look further into this problem and
devise a means of tackling it. The task force was made up of
members from the customer relations management (CRM),
the marketing, and the information technology (IT)
departments. Initially, the team explored multiple ways to
study the churn problem. Various methods were proposed to
prevent customer churn and boost revenue. These solutions
ranged from creating reactive market strategies to reduce
churn to building decision support tools that provide real-
time ability to generate insights about churn trends. The task
force also considered contemporary approaches, such as the
use of social network theory as a means of studying churn
analysis as was used in Dasgupta et al. (2008). Such
approaches utilize the strength of ties and other
communication patterns between individuals as a way of
analyzing churn activities among telecom customers.
Even though such methods could have been used, the
task force members recognized that the exponential increase
in the amount and variety of data they are collecting gave
them an opportunity to go far beyond current analytics
techniques. For example, NHT has multiple sources of data
about customer interactions. When customers have an issue
about a bill, payment, call quality, or the device itself, they
can interact with the company in many different ways:
visiting a physical store or a company website or calling the
company call center. Each channel creates its own records of
customer interactions. Thus, a lot of data is collected about
customer interactions. However, given the different formats
and data stores in which these interactions are recorded, a
single view of the interactions by the same customer may not
be visible. Thus, not much analysis is done to identify the
common sequences of customer attempts to resolve their
issues before cancelling their services. The task force
realized that existing predictive analytics approaches have
not been able to utilize all these sources and volume of data
in a holistic manner to generate actionable insights that are
both efficient and cost-effective.
3.2 The Challenge of Data
The CRM department at NHT had customer communication
records for each of three different channels of
communication: the website, physical stores, and call
centers. The challenges posed by the data structure to the
analysis of service cancellation were categorized into three
areas: data from multiple sources, data variety, and data
volume.
1. Data from Multiple Sources: As noted above, a
customer could connect with NHT by accessing their
accounts on the company’s website, allowing NHT
to generate weblog information on customer activity.
For instance, weblog tracking allowed the company
to know if and when a customer reviewed his current
plan, submitted a complaint, or checked his bill
online. Customers could also walk into an NHT
customer service center to lodge a service complaint,
request a plan change, or cancel the service. Lastly, a
customer could call the customer service center on
the phone and transact business just like he or she
would do in person at a customer service center. Any
one of these sources can support the analysis of
churn trends among customers using that channel.
However, only limited insight would be generated
and, hence, a remediation strategy would not be
comprehensive enough to solve the churn problem.
The task force deemed a combination of data from
all three sources as the best way of gaining a broad
scale and in-depth insights into the churn problem. In
effect, whatever methodology was implemented had
to leverage customer data from all three channels of
data in order to generate rich insights.
2. Data Variety: An attendant issue, based on the
multiple sources of data, was the variety of data that
was generated by each means of customer
communication. That is, data derived from online,
call center, and walk-in activities were all of
different structures. The original data from the three
sources were in an unstructured format. For instance,
the call center data was originally audio recordings
which had to be first transcribed into text before
being transformed into a structured format. In order
to perform meaningful analysis on data from all
sources, the individual data sets had to be converted
into similar structured formats.
3. Data Volume: The third hurdle that was created by
the data was the vast amount of data that had to be
analyzed. Previous data analytics projects mostly
utilized a small sample set of data for analysis.
However, the company wanted to leverage the
multiple variety and sources of data to generate as
much insights as possible.
Journal of Information Systems Education, Vol. 27(4) Fall 2016