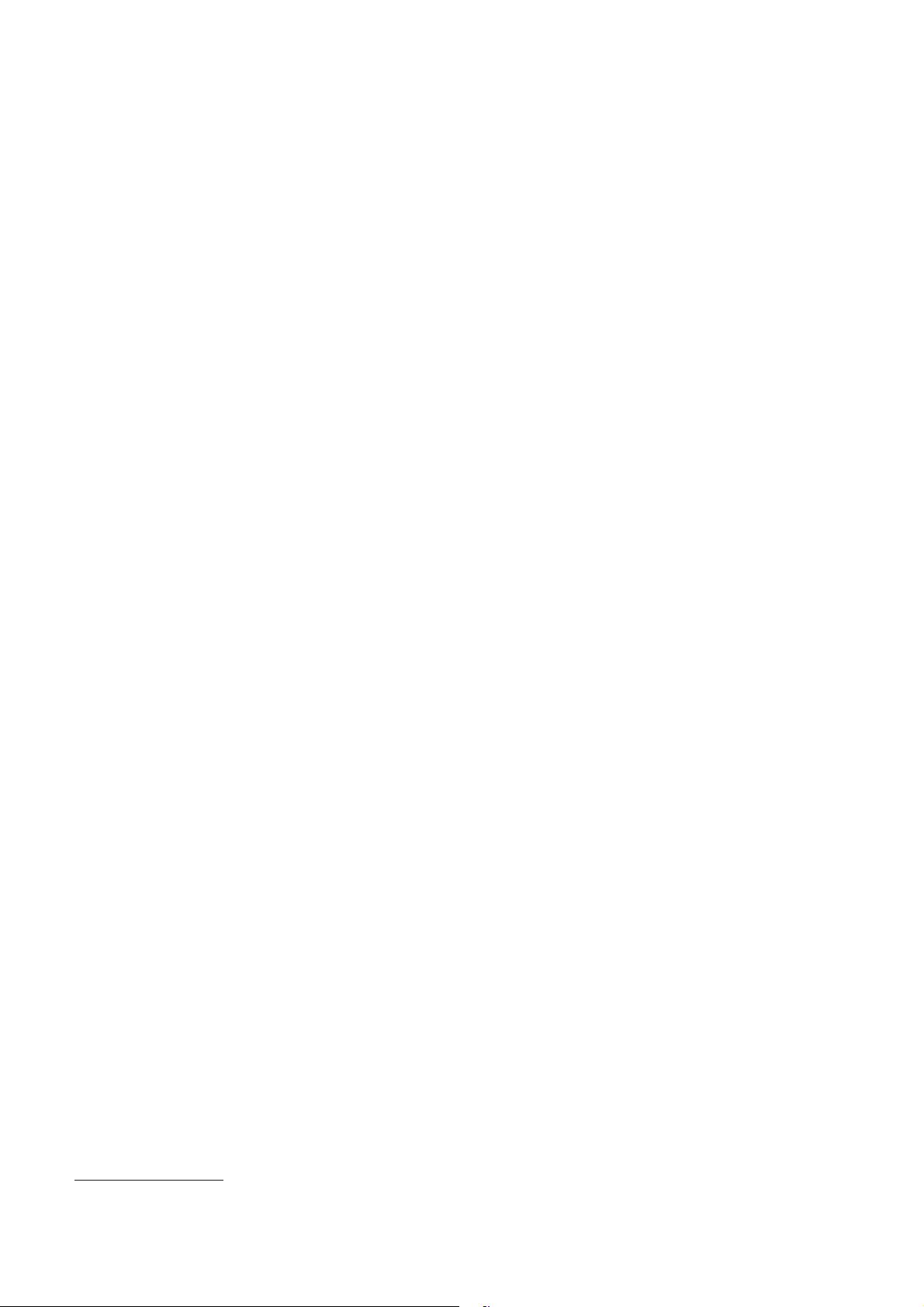
APrognosticApproachforSystemsSubjecttoWiener
DegradationProcessZithCumulative-WypeRandomShocks
ZhengxinZhang,ChanghuaHu,XiaoshengSi,Jian[unZhang,QuanShi
DepartmentofAutomationEngineering,Xi’anResearchInstituteofHigh-Tech,Xi’an,710025
E-mail:zhangzhengxin13@gmail.com
Abstract:Conditionmonitoringdatahavebeenwidelyusedtoevaluatethehealthstateandreliability,aswellasestimatethe
remainingusefullife(RUL)fordegradingsystems.AmongvariousdegradationmodelingandRULestimatingmethods,Wiener
processbasedmodelsL
Vrecognizedbybothscholarsandengineersastheoneofthemosteffecttools,andthusbecomesvery
popular nowadays. In this paper, a prognostic approach is developed for degrading systems whose performance evolution is
captureGbyanintegrationofWienerprocessandcumulative-typeofrandomshocksdepictedbyacompoundPoissonpro-cess.
Under the concept of first hitting time, an approximate lifetime distribution in analytical form, which greatly reduces the
computingtimewhileprovinganadequatelyaccurateresult,hasbeenformulated. Theparameterestimationframeworkbased
expectation maximization (EM) algorithm has been derived. Simulations and caseV study of the degradation of Li-ion
batteriesareexecutedtoillustrateandvalidatetheproposedmethod.Theresultsdemonstratethattheapproachinthispapercan
notonlyhandlethepositiveshocksbutalsoprocessthenegativeshocks,whichoutperformsmostoftheexistingmodels.
Key Words: Reliability, Wiener Process, Degradation-shock Models, Compound Poisson Process, RUL Estimation
1 Introduction
The performance of an industrial system usually decreas-
es with its service time, due to the interaction of its inner
deterioration in materials performance with its working en-
vironment. The system fails once it cannot provide an an-
ticipant performance, and the unexpected failure of system
will cause economical losses and environmental damage. If
the lifetime or remaining useful lifetime (RUL) can be esti-
mated before failure, sequential managerial activities will be
arranged, to avoid disastrous failure, cut down costs and pro-
tect the environment. However, it is costly in both time and
money to obtain adequate lifetime data to evaluate the relia-
bility and estimate the lifetime and RUL for these degrading
systems, especially the ones with extremely long life or high
price. Fortunately, the development of condition monitor-
ing (CM) techniques makes it possible to collect sufficient
data revealing system’s health state, which further leads to
the boom stage of degradation modeling based approaches
[1, 2].
Indeed, there are various type of models for degradation
data analysis and RUL estimation, such as the failure-of-
physics based models, the data-driven models, the hybrid
methods, etc [3]. Since the degradation mechanism of a
system is often quite complicated, data-driven models draw
much for favor. The stochastic dynamics involved in the
degradation path, which usually caused by the uncertainty
during the degradation process, expedites the widest utiliza-
tion of stochastic process based models among other data-
driven methods. Particularly, Markovian chain [4], Gamma
process [5], Inverse Gaussian process[6], and Wiener pro-
cess [8] are all applied to degradation modeling. We con-
centrates on the Wiener process based degradation models,
because of its capacity to capture non-monotonous degrada-
tion processes which are frequently encountered in industry.
Besides, the degradation process may exhibit some ran-
dom shocks which suddenly increase or decrease the degra-
This work is supported by National Natural Science Foundation (NNS-
F) of China under Grants 61603398,61374126, 61104223, and 61573365.
dation level of the system. The underlying causes of the
random shocks are various, i.e., shocks in the environmen-
t, repair activities with ignorable time, recovery of material
nature, can all introduce shocks into the degradation pro-
cess. According to the work of in [7], there are general-
ly fine types of random shocks mentioned in the literature,
including the extreme shock, cumulative shock, 𝑚-shock,
run shock, and 𝛿 shock. Extreme shocks cause system fail-
ure when the size of any shock is beyond a specific critical
threshold. Systems experience cumulative shocks will fail
when the cumulated damage due to shocks exceeds a fail-
ure threshold. 𝑚-shocks are defined as the 𝑚 shocks whose
amplitude is larger than a threshold and will cause the fail-
ure of system, while run shock means a run of 𝑛 consec-
utive shock that a greater than a threshold. 𝛿 shocks are
two sequential shocks whose inter-arrival time is less than
a threshold 𝛿. Among all these kinds of random shocks,
cumulative-type kind of random shocks are most frequently-
encountered in industrial practice. Therefore, we carried out
our study based on cumulative-type of random shocks. Al-
though, some researchers have reported their work regard-
ing the degradation-shock prognostic model [10, 11], there
are still some issues deserving further address. At the very
first, compared to most degradation-shock models construct-
ed in the framework of general path models without consid-
ering the temporal dynamics of the degradation paths, ran-
dom shocks have seldom been incorporated into stochas-
tic process model, especially Wiener process based model.
Then, the computation of lifetime and RUL probability den-
sity functions (PDFs) in the existing method requires trun-
cation and is time costly. Additionally, the parameter es-
timation procedure for degradation-shock models is greatly
desired to make the degradation-shock model more practical.
Motivated by the aforementioned discussions, we pro-
posed a prognostic approach for degrading systems whose
performance evolution is described by a Wiener process with
cumulative-type of random-shocks. The lifetime and RUL
distributions are derived under the concept of the first hitting
time (FHT). Also, an approximate to the derived lifetime and
2017 IEEE 6th Data Driven Control and Learning Systems Conference
Ma
26-27, 2017, Chon
qin
, China
978-1-5090-5461-9/17/$31.00 ©2017 IEEE
DDCLS'17
694