随机森林网格搜索调参绘图
时间: 2023-06-30 09:23:00 浏览: 121
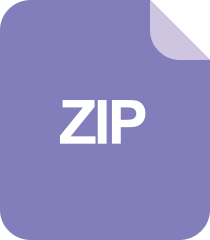
机器学习\深度学习绘图模板
好的,针对你的问题,我可以给出以下的建议。
首先,需要导入必要的库和数据集。以Python为例,可以使用以下代码:
```python
import numpy as np
import pandas as pd
from sklearn.ensemble import RandomForestRegressor
from sklearn.model_selection import GridSearchCV
import matplotlib.pyplot as plt
# 导入数据集
data = pd.read_csv('data.csv')
X = data.drop('target', axis=1)
y = data['target']
```
接着,我们可以对随机森林模型进行网格搜索调参。这里以最大深度和最小叶子节点数作为调参参数,代码如下:
```python
# 定义参数范围
param_grid = {
'max_depth': [10, 20, 30],
'min_samples_leaf': [1, 2, 4]
}
# 定义随机森林模型
rf = RandomForestRegressor(n_estimators=100)
# 进行网格搜索调参
grid_search = GridSearchCV(estimator=rf, param_grid=param_grid, cv=5)
grid_search.fit(X, y)
# 输出最优参数
print(grid_search.best_params_)
```
最后,我们可以利用Matplotlib库绘制调参过程中随着参数变化的模型性能曲线。代码如下:
```python
# 获取参数范围
param_range = param_grid['max_depth']
# 获取交叉验证结果
cv_results = grid_search.cv_results_
# 绘制曲线
plt.figure()
plt.title("GridSearchCV evaluating using multiple scorers simultaneously")
plt.xlabel("max_depth")
plt.ylabel("Score")
plt.ylim(0, 1)
# 获取不同评估指标的结果
plt.grid()
scoring = 'neg_mean_squared_error'
plt.plot(param_range, cv_results['mean_test_score'],
label="MSE",
color='navy')
scoring = 'r2'
plt.plot(param_range, cv_results['mean_test_score'],
label="R2",
color='darkorange')
plt.legend(loc="best")
plt.show()
```
这样就可以得到一张随着最大深度变化和评估指标变化的模型性能曲线图。
希望这些代码可以帮助你实现随机森林网格搜索调参绘图。
阅读全文
相关推荐
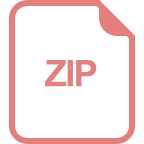
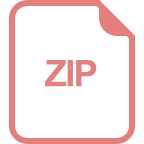

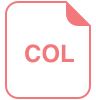
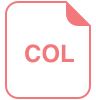
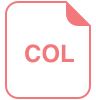




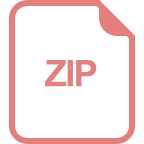
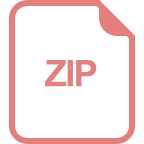
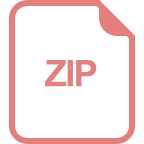
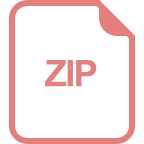