def test(): # 加载测试数据 test_loader = torch.utils.data.DataLoader( datasets.CIFAR10(root = args.data, train=False, transform=transforms.Compose([ transforms.ToTensor(), transforms.Normalize((0.4914, 0.4822, 0.4465), (0.2023, 0.1994, 0.2010))])), batch_size=8, shuffle=False, num_workers=0) model.eval() correct = 0 for data, target in test_loader: if not args.cpu: data, target = data.cuda(), target.cuda() data, target = Variable(data), Variable(target) output = model(data) pred = output.data.max(1, keepdim=True)[1] torch.squeeze() correct += pred.eq(target.data.view_as(pred)).cpu().sum()
时间: 2023-07-16 21:13:07 浏览: 48
这段代码是一个用于测试模型准确率的函数。首先,它加载了 CIFAR10 数据集的测试数据,这些数据已经通过了预处理(使用 transforms.ToTensor() 将图像转换为张量,使用 transforms.Normalize() 对张量进行归一化)。然后,它将模型设置为评估模式(model.eval()),并初始化正确分类的计数器(correct)。
接下来,它迭代测试数据集中的每个批次,并将数据和标签分别存储在 data 和 target 变量中。如果 GPU 可用,数据和目标将移动到 GPU 上。然后,它将数据和目标封装在变量中(Variable(data) 和 Variable(target))。接着,它使用模型对数据进行预测,并将输出存储在 output 变量中。然后,它使用 output.data.max(1, keepdim=True)[1] 获取预测标签,并将其存储在 pred 变量中。接着,它使用 torch.squeeze() 函数去除 pred 张量中的所有尺寸为 1 的维度。最后,它将正确分类的数量加上 pred 与 target 相等的数量,并将结果存储在 correct 变量中。
该函数返回正确分类的数量。
相关问题
def test(): # 加载测试数据 test_loader = torch.utils.data.DataLoader( datasets.CIFAR10(root = args.data, train=False, transform=transforms.Compose([ transforms.ToTensor(), transforms.Normalize((0.4914, 0.4822, 0.4465), (0.2023, 0.1994, 0.2010))])), batch_size=8, shuffle=False, num_workers=0) model.eval() correct = 0
这段代码定义了一个名为 `test` 的函数,该函数用于测试训练好的模型在 CIFAR10 数据集上的准确率。
首先,通过 `torch.utils.data.DataLoader` 加载 CIFAR10 数据集,并进行了数据预处理,包括将图片转化为张量,并进行归一化处理。
然后,将模型设置为评估模式,即 `model.eval()`。
最后,定义了一个变量 `correct`,用于存储分类正确的样本数。
import torch, os, cv2 from model.model import parsingNet from utils.common import merge_config from utils.dist_utils import dist_print import torch import scipy.special, tqdm import numpy as np import torchvision.transforms as transforms from data.dataset import LaneTestDataset from data.constant import culane_row_anchor, tusimple_row_anchor if __name__ == "__main__": torch.backends.cudnn.benchmark = True args, cfg = merge_config() dist_print('start testing...') assert cfg.backbone in ['18','34','50','101','152','50next','101next','50wide','101wide'] if cfg.dataset == 'CULane': cls_num_per_lane = 18 elif cfg.dataset == 'Tusimple': cls_num_per_lane = 56 else: raise NotImplementedError net = parsingNet(pretrained = False, backbone=cfg.backbone,cls_dim = (cfg.griding_num+1,cls_num_per_lane,4), use_aux=False).cuda() # we dont need auxiliary segmentation in testing state_dict = torch.load(cfg.test_model, map_location='cpu')['model'] compatible_state_dict = {} for k, v in state_dict.items(): if 'module.' in k: compatible_state_dict[k[7:]] = v else: compatible_state_dict[k] = v net.load_state_dict(compatible_state_dict, strict=False) net.eval() img_transforms = transforms.Compose([ transforms.Resize((288, 800)), transforms.ToTensor(), transforms.Normalize((0.485, 0.456, 0.406), (0.229, 0.224, 0.225)), ]) if cfg.dataset == 'CULane': splits = ['test0_normal.txt', 'test1_crowd.txt', 'test2_hlight.txt', 'test3_shadow.txt', 'test4_noline.txt', 'test5_arrow.txt', 'test6_curve.txt', 'test7_cross.txt', 'test8_night.txt'] datasets = [LaneTestDataset(cfg.data_root,os.path.join(cfg.data_root, 'list/test_split/'+split),img_transform = img_transforms) for split in splits] img_w, img_h = 1640, 590 row_anchor = culane_row_anchor elif cfg.dataset == 'Tusimple': splits = ['test.txt'] datasets = [LaneTestDataset(cfg.data_root,os.path.join(cfg.data_root, split),img_transform = img_transforms) for split in splits] img_w, img_h = 1280, 720 row_anchor = tusimple_row_anchor else: raise NotImplementedError for split, dataset in zip(splits, datasets): loader = torch.utils.data.DataLoader(dataset, batch_size=1, shuffle = False, num_workers=1) fourcc = cv2.VideoWriter_fourcc(*'MJPG') print(split[:-3]+'avi') vout = cv2.VideoWriter(split[:-3]+'avi', fourcc , 30.0, (img_w, img_h)) for i, data in enumerate(tqdm.tqdm(loader)): imgs, names = data imgs = imgs.cuda() with torch.no_grad(): out = net(imgs) col_sample = np.linspace(0, 800 - 1, cfg.griding_num) col_sample_w = col_sample[1] - col_sample[0] out_j = out[0].data.cpu().numpy() out_j = out_j[:, ::-1, :] prob = scipy.special.softmax(out_j[:-1, :, :], axis=0) idx = np.arange(cfg.griding_num) + 1 idx = idx.reshape(-1, 1, 1) loc = np.sum(prob * idx, axis=0) out_j = np.argmax(out_j, axis=0) loc[out_j == cfg.griding_num] = 0 out_j = loc # import pdb; pdb.set_trace() vis = cv2.imread(os.path.join(cfg.data_root,names[0])) for i in range(out_j.shape[1]): if np.sum(out_j[:, i] != 0) > 2: for k in range(out_j.shape[0]): if out_j[k, i] > 0: ppp = (int(out_j[k, i] * col_sample_w * img_w / 800) - 1, int(img_h * (row_anchor[cls_num_per_lane-1-k]/288)) - 1 ) cv2.circle(vis,ppp,5,(0,255,0),-1) vout.write(vis) vout.release()
这段代码使用了PyTorch、OpenCV等库,从模型模块中导入了parsingNet模型,从常用工具模块中导入了merge_config和dist_print等函数。代码还使用了LaneTestDataset数据集和culane_row_anchor、tusimple_row_anchor常量。在if __name__ == "__main__":中,代码设置了torch.backends.cudnn.benchmark为True,合并了配置信息args和cfg,并输出了“start testing...”信息。然后根据配置信息中的backbone选择了不同的通道数,并初始化了一个parsingNet模型。
相关推荐
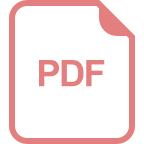
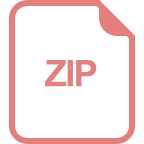













