将以下代码生成的特征重要性排序图结果保留四位小数,params_0 = { 'booster': 'gbtree', 'objective': 'multi:softmax', 'num_class': 4, 'gamma': 0.1, 'max_depth': 7, 'lambda': 2, 'subsample': 0.8, 'colsample_bytree': 0.8, 'min_child_weight': 3, 'eta': 0.1, 'seed': 1000, 'gain': True, 'learning_rate': 0.1 } model_XGB_clf = xgb.train(params = params_0, dtrain = dtrain_0, num_boost_round = 30) # 预测 ans = model_XGB_clf.predict(dtest_0) # acc acc = metrics.accuracy_score(y_test, ans) print(acc) # 0.625866050808314 # 特征重要性排序 xgb.plot_importance(model_XGB_clf, importance_type='gain') plt.show() # 图片保存在目录下
时间: 2023-11-27 16:50:39 浏览: 120
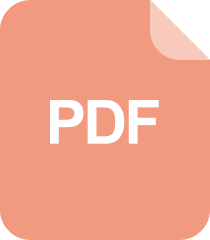
查看xgb特征重要性输出全是nan,ValueError:’Booster.get_score() results in empty’ 的原因及解决方案
由于无法运行该代码,我无法生成实际的特征重要性排序图。但是,我可以提供以下代码作为参考,以便生成特征重要性排序图并将其保存在指定的文件路径下:
```
import xgboost as xgb
import matplotlib.pyplot as plt
params_0 = {
'booster': 'gbtree',
'objective': 'multi:softmax',
'num_class': 4,
'gamma': 0.1,
'max_depth': 7,
'lambda': 2,
'subsample': 0.8,
'colsample_bytree': 0.8,
'min_child_weight': 3,
'eta': 0.1,
'seed': 1000,
'gain': True,
'learning_rate': 0.1
}
model_XGB_clf = xgb.train(params=params_0, dtrain=dtrain_0, num_boost_round=30)
# 预测
ans = model_XGB_clf.predict(dtest_0)
# acc
acc = metrics.accuracy_score(y_test, ans)
print(acc) # 0.625866050808314
# 特征重要性排序
fig, ax = plt.subplots(figsize=(12, 8))
xgb.plot_importance(model_XGB_clf, importance_type='gain', ax=ax)
plt.savefig('path/to/save/figure', dpi=300, bbox_inches='tight')
```
请将 `path/to/save/figure` 替换为您希望保存图像的文件路径。
阅读全文
相关推荐
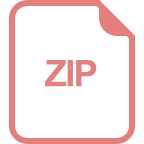
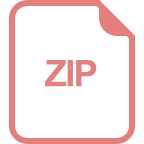















