中文本文分词、去停用词、关键词提取、向量化、文本相似度计算代码
时间: 2023-07-10 20:30:04 浏览: 52
以下是Python代码实现,需要使用jieba、pandas、sklearn等库:
1. 分词
```python
import jieba
def cut_words(text):
words = jieba.cut(text)
return ' '.join(words)
```
2. 去停用词
```python
import pandas as pd
def remove_stopwords(words):
stopwords = pd.read_csv('stopwords.txt', index_col=False, quoting=3, sep='\t', names=['stopword'], encoding='utf-8')
words = words.split(' ')
words = [word for word in words if word not in stopwords]
return ' '.join(words)
```
3. 关键词提取
```python
from sklearn.feature_extraction.text import TfidfVectorizer
def get_keywords(text):
vectorizer = TfidfVectorizer(max_features=200)
X = vectorizer.fit_transform([text])
feature_names = vectorizer.get_feature_names()
keywords = [feature_names[index] for index in X.toarray()[0].argsort()[::-1][:10]]
return keywords
```
4. 向量化
```python
def get_vectors(text1, text2):
vectorizer = TfidfVectorizer()
X = vectorizer.fit_transform([text1, text2])
return X.toarray()
```
5. 文本相似度计算
```python
from sklearn.metrics.pairwise import cosine_similarity
def get_similarity(text1, text2):
vectors = get_vectors(text1, text2)
similarity = cosine_similarity(vectors[0].reshape(1, -1), vectors[1].reshape(1, -1))[0][0]
return similarity
```
以上代码仅供参考,实际应用时需要根据具体需求进行修改。
相关推荐
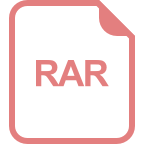
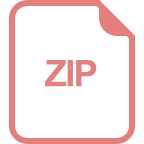














