Bayesian Color Constancy Revisited
时间: 2024-05-28 17:12:51 浏览: 11
Bayesian Color Constancy is a computational framework that attempts to solve the problem of color constancy in computer vision. Color constancy refers to the ability of the human visual system to perceive the colors of objects as relatively constant, despite changes in the spectral composition of the illuminating light.
The Bayesian Color Constancy framework is based on the idea that the visual system should use prior knowledge about the statistical properties of the colors in the scene, as well as the statistical properties of the illuminant, to infer the true colors of the objects in the scene. This is done by formulating a probabilistic model of the relationship between the colors of the objects and the illuminant, and then using Bayesian inference to estimate the most likely illuminant given the observed colors.
The paper "Bayesian Color Constancy Revisited" presents a review of the Bayesian Color Constancy framework, and proposes several improvements to the original model. One of the main contributions of the paper is the development of a new method for estimating the prior distribution of illuminants, based on a statistical analysis of the colors in a large set of natural images. The authors also propose several modifications to the likelihood function used in the model, in order to better capture the properties of real-world images.
Overall, the paper provides a comprehensive overview of the Bayesian Color Constancy framework, and presents several important improvements and extensions to the original model. These developments have the potential to improve the accuracy and robustness of color constancy algorithms in computer vision applications.
相关推荐
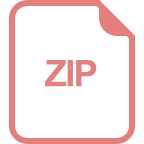














