哈哈: df_content[ label ] = data.label 哈哈: label_map = { 体育 :1, 财经 :2, 房产 :3, 家居 :4, 教育 :5, 科技 :6, 时尚 :7, 时政 :8, 游戏 :9, 娱乐 :10} 哈哈: df_content[ label ] = df_content[ label ]
时间: 2024-04-06 10:33:54 浏览: 32
看起来你在描述一个数据处理的过程。你的代码中似乎有一些语法错误,我猜测你想要给一个名为 "df_content" 的数据框添加一个名为 "label" 的列,并将其值设置为 "data.label"。之后又创建了一个字典 "label_map",其中包含了标签名和其对应的数字编号。最后,你将 "df_content" 中的 "label" 列的值替换为其对应的数字编号。这个过程可能是为了将文本数据转化为机器学习模型可以处理的数字形式。
相关问题
封装这段函数while True: data_str = input('请输入黏度(mp*s)与密度(kg/l),以逗号分隔:(输入exit以停止)') if data_str == 'exit': break data = np.array([list(map(float, data_str.split(',')))]) label = kmeans.predict(data)[0] center_idx = np.argmin(np.sum(np.square(centers - data), axis=1)) print('该数据所属类别为:', label, ',模糊判断到聚类中心:', center_idx) print('对应表格中的转速和时间范围为:', df.iloc[kmeans.labels_ == label, 3:5].values)
好的,这里是一个封装后的函数,它可以接收用户的输入,使用k-means算法对输入的数据进行聚类,并输出该数据所属的类别以及对应的转速和时间范围。函数的名称为`cluster_and_print`,它接收一个参数`kmeans`,表示已经训练好的k-means模型。
```python
import numpy as np
def cluster_and_print(kmeans):
"""
从用户输入中获取黏度和密度,使用k-means算法对数据进行聚类,并输出聚类结果及对应的转速和时间范围。
参数kmeans为已经训练好的k-means模型。
"""
while True:
data_str = input('请输入黏度(mp*s)与密度(kg/l),以逗号分隔:(输入exit以停止)')
if data_str == 'exit':
break
data = np.array([list(map(float, data_str.split(',')))])
label = kmeans.predict(data)[0]
center_idx = np.argmin(np.sum(np.square(kmeans.cluster_centers_ - data), axis=1))
print('该数据所属类别为:', label, ',模糊判断到聚类中心:', center_idx)
print('对应表格中的转速和时间范围为:', df.iloc[kmeans.labels_ == label, 3:5].values)
```
这个函数使用了一个无限循环,每次循环都会让用户输入一组黏度和密度数据,使用k-means算法对数据进行聚类,并输出聚类结果及对应的转速和时间范围。如果用户输入`exit`,函数会退出循环。注意,在这个函数中,我们使用了`kmeans.cluster_centers_`来获取聚类中心,而不是之前的`centers`变量。
param = {'num_leaves': 31, 'min_data_in_leaf': 20, 'objective': 'binary', 'learning_rate': 0.06, "boosting": "gbdt", "metric": 'None', "verbosity": -1} trn_data = lgb.Dataset(trn, trn_label) val_data = lgb.Dataset(val, val_label) num_round = 666 # clf = lgb.train(param, trn_data, num_round, valid_sets=[trn_data, val_data], verbose_eval=100, # early_stopping_rounds=300, feval=win_score_eval) clf = lgb.train(param, trn_data, num_round) # oof_lgb = clf.predict(val, num_iteration=clf.best_iteration) test_lgb = clf.predict(test, num_iteration=clf.best_iteration)thresh_hold = 0.5 oof_test_final = test_lgb >= thresh_hold print(metrics.accuracy_score(test_label, oof_test_final)) print(metrics.confusion_matrix(test_label, oof_test_final)) tp = np.sum(((oof_test_final == 1) & (test_label == 1))) pp = np.sum(oof_test_final == 1) print('accuracy1:%.3f'% (tp/(pp)))test_postive_idx = np.argwhere(oof_test_final == True).reshape(-1) # test_postive_idx = list(range(len(oof_test_final))) test_all_idx = np.argwhere(np.array(test_data_idx)).reshape(-1) stock_info['trade_date_id'] = stock_info['trade_date'].map(date_map) stock_info['trade_date_id'] = stock_info['trade_date_id'] + 1tmp_col = ['ts_code', 'trade_date', 'trade_date_id', 'open', 'high', 'low', 'close', 'ma5', 'ma13', 'ma21', 'label_final', 'name'] stock_info.iloc[test_all_idx[test_postive_idx]] tmp_df = stock_info[tmp_col].iloc[test_all_idx[test_postive_idx]].reset_index() tmp_df['label_prob'] = test_lgb[test_postive_idx] tmp_df['is_limit_up'] = tmp_df['close'] == tmp_df['high'] buy_df = tmp_df[(tmp_df['is_limit_up']==False)].reset_index() buy_df.drop(['index', 'level_0'], axis=1, inplace=True)buy_df['buy_flag'] = 1 stock_info_copy['sell_flag'] = 0tmp_idx = (index_df['trade_date'] == test_date_min+1) close1 = index_df[tmp_idx]['close'].values[0] test_date_max = 20220829 tmp_idx = (index_df['trade_date'] == test_date_max) close2 = index_df[tmp_idx]['close'].values[0]tmp_idx = (stock_info_copy['trade_date'] >= test_date_min) & (stock_info_copy['trade_date'] <= test_date_max) tmp_df = stock_info_copy[tmp_idx].reset_index(drop=True)from imp import reload import Account reload(Account) money_init = 200000 account = Account.Account(money_init, max_hold_period=20, stop_loss_rate=-0.07, stop_profit_rate=0.12) account.BackTest(buy_df, tmp_df, index_df, buy_price='open')tmp_df2 = buy_df[['ts_code', 'trade_date', 'label_prob', 'label_final']] tmp_df2 = tmp_df2.rename(columns={'trade_date':'buy_date'}) tmp_df = account.info tmp_df['buy_date'] = tmp_df['buy_date'].apply(lambda x: int(x)) tmp_df = tmp_df.merge(tmp_df2, on=['ts_code', 'buy_date'], how='left')最终的tmp_df是什么?tmp_df[tmp_df['label_final']==1]又选取了什么股票?
根据代码逐行分析,tmp_df 是一个 DataFrame,包含了股票的信息以及回测结果。其中,选取了 label_final 为 1 的股票,也就是模型预测为涨的股票,并且过滤掉了当天涨停的股票。最终买入的股票信息保存在 buy_df 中,回测时将其与 stock_info_copy、index_df 进行合并,得到了回测的结果,并将买入的股票信息与回测结果合并,生成了最终的 tmp_df。
相关推荐
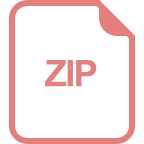
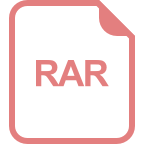
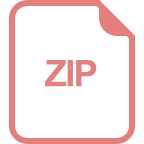












