使用OpenML对anneal数据集进行分析,我需要python代码
时间: 2024-05-13 11:14:12 浏览: 126
好的,以下是使用Python的OpenML库对anneal数据集进行分析的代码:
```python
import openml
import pandas as pd
# 获取数据集
dataset = openml.datasets.get_dataset(38)
# 获取数据集的详细信息
print(dataset.description)
# 获取数据集的特征和标签
X, y, _, _ = dataset.get_data(target=dataset.default_target_attribute)
# 转换为Pandas DataFrame
df = pd.DataFrame(X, columns=dataset.feature_names)
df['class'] = y
# 输出前5行数据
print(df.head())
```
运行代码后,会输出数据集的详细信息和前5行数据。你可以根据需要对数据集进行进一步的分析和处理。
相关问题
生成一段python代码:1导入PCA 2使用sklearn.datasets导入fetch_openml数据集 3# 通过fetch_openml("mnist_784")得到mnist数据,并使用data和target索引得到数据与标签 4划分mnist数据集,训练集60000,测试集10000 5查看训练集和测试集维度
下面是您需要的Python代码:
```python
# 导入PCA
from sklearn.decomposition import PCA
# 使用sklearn.datasets导入fetch_openml数据集
from sklearn.datasets import fetch_openml
# 通过fetch_openml("mnist_784")得到mnist数据,并使用data和target索引得到数据与标签
mnist = fetch_openml("mnist_784")
X = mnist.data
y = mnist.target
# 划分mnist数据集,训练集60000,测试集10000
X_train, X_test = X[:60000], X[60000:]
y_train, y_test = y[:60000], y[60000:]
# 查看训练集和测试集维度
print("训练集维度:", X_train.shape)
print("测试集维度:", X_test.shape)
```
这段代码首先导入了PCA和fetch_openml,然后使用fetch_openml函数获取了mnist数据集,并使用data和target索引得到了数据和标签。接着将数据集划分为训练集和测试集,最后输出了训练集和测试集的维度。
用决策树对minist数据集进行识别python代码
以下是使用决策树对MNIST数据集进行识别的Python代码:
```python
from sklearn.datasets import fetch_openml
from sklearn.model_selection import train_test_split
from sklearn.tree import DecisionTreeClassifier
from sklearn.metrics import accuracy_score
# 获取MNIST数据集
mnist = fetch_openml('mnist_784')
X, y = mnist["data"], mnist["target"]
X_train, X_test, y_train, y_test = train_test_split(X, y, test_size=0.2, random_state=42)
# 训练决策树模型
tree_clf = DecisionTreeClassifier(random_state=42)
tree_clf.fit(X_train, y_train)
# 在测试集上评估模型
y_pred = tree_clf.predict(X_test)
accuracy = accuracy_score(y_test, y_pred)
print("Accuracy:", accuracy)
```
注意,这段代码使用的是sklearn库内置的MNIST数据集。如果你使用的是其他来源的MNIST数据集,可能需要对数据进行一些预处理。
阅读全文
相关推荐
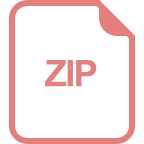
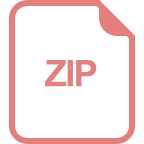
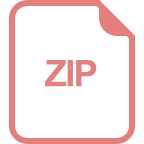










