fetch_openml怎么读取fashion-mnist数据集
时间: 2023-10-28 12:06:08 浏览: 264
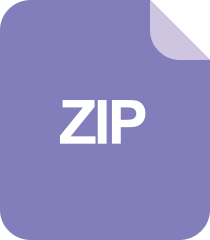
Python库 | fetch_latest_file-0.0.5-py2.py3-none-any.whl
你可以使用`fetch_openml`函数来读取fashion-mnist数据集。下面是一个示例代码:
```python
from sklearn.datasets import fetch_openml
# 读取fashion-mnist数据集
X, y = fetch_openml('Fashion-MNIST', version=1, return_X_y=True)
```
在这个例子中,`fetch_openml`函数的第一个参数是数据集的名称,即"Fashion-MNIST"。`version`参数指定要使用的数据集版本,这里使用版本1。`return_X_y`参数设置为True,表示返回特征矩阵和标签向量。
读取后,`X`将是一个形状为(70000, 784)的NumPy数组,其中每一行表示一张28x28像素的灰度图像。`y`将是一个形状为(70000,)的NumPy数组,其中每个元素是一个整数,表示对应图像的类别标签。
阅读全文
相关推荐
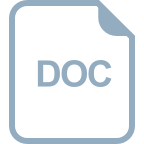
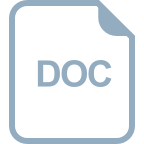















