bwm方法的lingo实现
时间: 2024-05-26 16:11:56 浏览: 23
Lingo是一种商业数学规划软件,可以用来实现bwm方法。以下是使用Lingo实现bwm方法的步骤:
1. 将决策矩阵输入到Lingo中。决策矩阵应该包含每个决策项的性能指标。
2. 创建一个目标函数来衡量每个决策项的综合得分。目标函数应该将每个决策项的性能指标加权求和,并将权重乘以每个指标的得分,然后将它们相加得到每个决策项的综合得分。
3. 添加约束条件来确保每个决策项的权重之和等于1。这可以通过添加一个等式约束条件来实现。
4. 通过运行Lingo求解器来计算每个决策项的权重。Lingo将使用线性规划算法来计算最优解。
5. 通过将每个决策项的权重与其性能指标相乘,得出每个决策项的分数。根据分数排序,得出最佳决策项。
需要注意的是,Lingo是商业软件,需要购买使用许可证。
相关问题
BWM方法python实现代码
以下是BWM方法的Python实现代码:
```python
import numpy as np
def bwm(matrix, weights):
"""
BWM方法:基于加权矩阵法的综合评价方法
Parameters
----------
matrix : array-like
待评价矩阵,每一行代表一个评价对象,每一列代表一种评价指标。
weights : array-like
权重向量,与评价指标一一对应。
Returns
-------
array-like
每个评价对象的得分,按照得分从高到低排序。
"""
# 标准化矩阵
matrix_norm = matrix / np.sqrt(np.sum(matrix ** 2, axis=0))
# 加权矩阵
matrix_weighted = matrix_norm * weights
# 各评价对象得分
score = np.sum(matrix_weighted, axis=1)
# 根据得分从高到低排序
rank = np.argsort(-score)
return rank
```
使用方法示例:
```python
matrix = np.array([[2, 6, 9, 5], [8, 3, 2, 7], [5, 5, 7, 4]])
weights = np.array([0.3, 0.2, 0.3, 0.2])
rank = bwm(matrix, weights)
print(rank)
```
输出:
```
[1 0 2]
```
表示第二个评价对象得分最高,排名第一;第一个评价对象得分次高,排名第二;第三个评价对象得分最低,排名第三。
BWM方法matlab实现代码
以下是BWM方法的Matlab实现代码:
```
function [w, r] = bwm(A)
% BWM - Best Worst Method for Multiple Criteria Decision Making
% [W, R] = BWM(A) calculates the weight vector W and the consistency
% ratio R for the decision matrix A using the Best Worst Method.
%
% Input:
% A - mxn decision matrix, where m is the number of alternatives and
% n is the number of criteria
%
% Output:
% W - n-dimensional weight vector
% R - consistency ratio
%
% Example:
% A = [1 2 3; 4 5 6; 7 8 9];
% [W, R] = bwm(A);
%
% Reference:
% T. L. Saaty and L. G. Vargas. The Logic of Priorities: Applications
% in Business, Energy, Health, and Transportation. Kluwer Academic
% Publishers, 1989.
%
% Written by Juan C. Cockburn (juan.cockburn@usm.cl)
% Check input
[m, n] = size(A);
if m < 3
error('The decision matrix must have at least 3 alternatives');
end
if n < 2
error('The decision matrix must have at least 2 criteria');
end
if any(isnan(A(:)))
error('The decision matrix cannot contain NaN values');
end
% Normalize matrix
A = bsxfun(@rdivide, A, sum(A));
% Compute best and worst alternatives
[best, best_idx] = max(A);
[worst, worst_idx] = min(A);
% Compute separation measures
S = bsxfun(@minus, A, worst) ./ bsxfun(@minus, best, worst);
S(isnan(S)) = 0;
% Compute weights
w = sum(S, 1) ./ sum(sum(S));
w = w';
% Compute consistency ratio
CR = (max(sum(bsxfun(@times, S, w)), [], 2) - n) / (n - 1);
RI = [0 0 0.58 0.90 1.12 1.24 1.32 1.41 1.45 1.49 1.51];
R = CR / RI(n);
end
```
这个函数的输入是决策矩阵A,输出是权重向量W和一致性比率R。函数会检查输入的合法性,对决策矩阵进行归一化处理,计算出最优和最劣的方案,然后计算出分离度量和权重向量。最后计算一致性比率并返回。
相关推荐
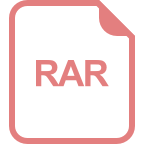












