ValueError: Exception encountered when calling layer "model_22" " f"(type Functional). Input 0 of layer "bidirectional_46" is incompatible with the layer: expected ndim=3, found ndim=4. Full shape received: (100, 200, 28, 128) Call arguments received by layer "model_22" " f"(type Functional): • inputs=tf.Tensor(shape=(100, 200, 28), dtype=float32) • training=True • mask=None
时间: 2024-02-14 11:35:21 浏览: 26
这个错误是由于模型的输入形状与期望的形状不匹配所导致的。具体来说,您的模型期望的输入是一个三维的张量,而您提供了一个四维的张量。这可能是由于您在输入数据中添加了额外的维度或数据格式不正确所导致的。
建议检查您的输入数据的形状和数据类型是否正确,并确保它们与模型期望的形状和数据类型相匹配。如果问题仍然存在,请提供更详细的代码和数据,以便更好地理解问题并提供帮助。
相关问题
ValueError: Exception encountered when calling layer 'rnn_model' (type RNNModel).
引用\[1\]中的代码是一个线性模型的定义,它继承了tf.keras.Model类,并重写了call方法来定义模型的前向传播过程。如果label_index为None,则直接返回输入;否则,返回输入的第label_index列,并在最后添加一个维度。引用\[2\]中的代码是实例化一个baseline模型,并编译了该模型的损失函数和评估指标。引用\[3\]中的代码是对线性模型进行了优化,定义了一个RepeatBaseline模型,并编译了该模型的损失函数和评估指标。然后,对该模型在验证集和测试集上进行了评估,并将评估结果保存在multi_val_performance和multi_performance中。最后,使用multi_window.plot方法绘制了repeat_baseline模型的图形。
根据提供的信息,无法确定ValueError异常的具体原因。ValueError通常表示输入的值不符合预期,可能是由于数据类型不匹配、维度不一致等问题引起的。建议检查代码中的输入数据是否正确,并确保模型的输入与数据的维度相匹配。另外,还可以尝试查看完整的错误信息以获取更多的上下文信息,以便更好地定位问题所在。
#### 引用[.reference_title]
- *1* *2* *3* [tensorflow 时间序列分析以及多个模型效果对比](https://blog.csdn.net/qq_42886846/article/details/126670703)[target="_blank" data-report-click={"spm":"1018.2226.3001.9630","extra":{"utm_source":"vip_chatgpt_common_search_pc_result","utm_medium":"distribute.pc_search_result.none-task-cask-2~all~insert_cask~default-1-null.142^v91^control_2,239^v3^insert_chatgpt"}} ] [.reference_item]
[ .reference_list ]
ValueError: Exception encountered when calling layer "rnn_7" " f"(type RNN).
这个错误通常表示在使用RNN(循环神经网络)时出现了值错误。可能的原因有很多,比如输入数据的形状不正确、模型架构不正确、训练数据集不完整等等。你可以尝试检查你的输入数据的形状是否正确,模型架构是否正确,还可以尝试使用更完整的数据集进行训练。如果这些方法都不起作用,你可能需要进一步调试代码以找出错误的根本原因。
相关推荐
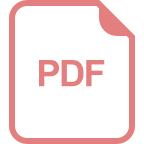
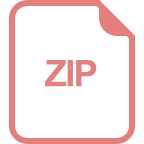
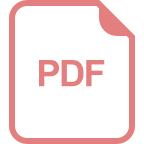












