create_model中的 base_model
时间: 2023-05-20 11:01:47 浏览: 71
在 create_model 中的 base_model 是指模型的基础模型,通常是预训练模型,如 GPT-2、BERT 等。在创建自己的模型时,可以选择不同的基础模型来进行 fine-tuning 或者进行进一步的训练。
相关问题
oss = self._train_model(input_fn, hooks, saving_listeners) File "E:\pycharm-workspace\BERT\BERT-BiLSTM-CRF-NER-master\venv\lib\site-packages\tensorflow_estimator\python\estimator\estimator.py", line 1161, in _train_model return self._train_model_default(input_fn, hooks, saving_listeners) File "E:\pycharm-workspace\BERT\BERT-BiLSTM-CRF-NER-master\venv\lib\site-packages\tensorflow_estimator\python\estimator\estimator.py", line 1191, in _train_model_default features, labels, ModeKeys.TRAIN, self.config) File "E:\pycharm-workspace\BERT\BERT-BiLSTM-CRF-NER-master\venv\lib\site-packages\tensorflow_estimator\python\estimator\estimator.py", line 1149, in _call_model_fn model_fn_results = self._model_fn(features=features, **kwargs) File "E:\pycharm-workspace\BERT\BERT-BiLSTM-CRF-NER-master\bert_base\train\bert_lstm_ner.py", line 405, in model_fn total_loss, learning_rate, num_train_steps, num_warmup_steps, False) File "E:\pycharm-workspace\BERT\BERT-BiLSTM-CRF-NER-master\bert_base\bert\optimization.py", line 27, in create_optimizer global_step = tf.train.get_or_create_global_step() UnboundLocalError: local variable 'tf' referenced before assignment 进程已结束,退出代码1
根据你提供的错误信息,看起来是在创建优化器时出现了问题。
错误提示 `UnboundLocalError: local variable 'tf' referenced before assignment` 表明在引用变量 `tf` 之前没有对其进行赋值。
这通常发生在没有正确导入 TensorFlow 库的情况下。请确保你的代码中包含了正确的 TensorFlow 导入语句,例如:
```python
import tensorflow as tf
```
如果你已经正确导入了 TensorFlow,但仍然遇到此错误,请检查你的代码中是否存在命名冲突或变量重复定义的情况。
另外,请确保你的代码中使用的 TensorFlow 版本与 transformers 库兼容。如果你使用的是较新版本的 transformers 库,可能需要更新你的代码以适应最新的 API 更改。
如果问题仍然存在,请提供更多的上下文信息,以便我能够更好地帮助你解决问题。
Create a model def create_LSTM_model(X_train,n_steps,n_length, n_features): # instantiate the model model = Sequential() model.add(Input(shape=(X_train.shape[1], X_train.shape[2]))) model.add(Reshape((n_steps, 1, n_length, n_features))) model.add(ConvLSTM2D(filters=64, kernel_size=(1,3), activation='relu', input_shape=(n_steps, 1, n_length, n_features))) model.add(Flatten()) # cnn1d Layers # 添加lstm层 model.add(LSTM(64, activation = 'relu', return_sequences=True)) model.add(Dropout(0.5)) #添加注意力层 model.add(LSTM(64, activation = 'relu', return_sequences=False)) # 添加dropout model.add(Dropout(0.5)) model.add(Dense(128)) # 输出层 model.add(Dense(1, name='Output')) # 编译模型 model.compile(optimizer='adam', loss='mse', metrics=['mae']) return model # lstm network model = create_LSTM_model(X_train,n_steps,n_length, n_features) # summary print(model.summary())修改该代码,解决ValueError Traceback (most recent call last) <ipython-input-56-6c1ed99fa3ed> in <module> 53 # lstm network 54 ---> 55 model = create_LSTM_model(X_train,n_steps,n_length, n_features) 56 # summary 57 print(model.summary()) <ipython-input-56-6c1ed99fa3ed> in create_LSTM_model(X_train, n_steps, n_length, n_features) 17 model = Sequential() 18 model.add(Input(shape=(X_train.shape[1], X_train.shape[2]))) ---> 19 model.add(Reshape((n_steps, 1, n_length, n_features))) 20 21 ~\anaconda3\lib\site-packages\tensorflow\python\trackable\base.py in _method_wrapper(self, *args, **kwargs) 203 self._self_setattr_tracking = False # pylint: disable=protected-access 204 try: --> 205 result = method(self, *args, **kwargs) 206 finally: 207 self._self_setattr_tracking = previous_value # pylint: disable=protected-access ~\anaconda3\lib\site-packages\keras\utils\traceback_utils.py in error_handler(*args, **kwargs) 68 # To get the full stack trace, call: 69 # tf.debugging.disable_traceback_filtering() ---> 70 raise e.with_traceback(filtered_tb) from None 71 finally: 72 del filtered_tb ~\anaconda3\lib\site-packages\keras\layers\reshaping\reshape.py in _fix_unknown_dimension(self, input_shape, output_shape) 116 output_shape[unknown] = original // known 117 elif original != known: --> 118 raise ValueError(msg) 119 return output_shape 120 ValueError: Exception encountered when calling layer "reshape_5" (type Reshape). total size of new array must be unchanged, input_shape = [10, 1], output_shape = [10, 1, 1, 5] Call arguments received by layer "reshape_5" (type Reshape): • inputs=tf.Tensor(shape=(None, 10, 1), dtype=float32)问题
将Reshape层的输出形状修改为(n_steps, n_length, n_features)即可解决此问题,代码如下:
```
def create_LSTM_model(X_train, n_steps, n_length, n_features):
# instantiate the model
model = Sequential()
model.add(Input(shape=(X_train.shape[1], X_train.shape[2])))
model.add(Reshape((n_steps, n_length, n_features)))
model.add(ConvLSTM2D(filters=64, kernel_size=(1,3), activation='relu', input_shape=(n_steps, n_length, n_features)))
model.add(Flatten())
# lstm Layers
model.add(LSTM(64, activation='relu', return_sequences=True))
model.add(Dropout(0.5))
model.add(LSTM(64, activation='relu', return_sequences=False))
model.add(Dropout(0.5))
model.add(Dense(128))
# output layer
model.add(Dense(1, name='Output'))
# compile model
model.compile(optimizer='adam', loss='mse', metrics=['mae'])
return model
# lstm network
model = create_LSTM_model(X_train, n_steps, n_length, n_features)
# summary
print(model.summary())
```
相关推荐
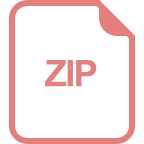
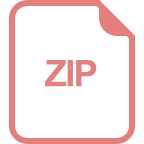
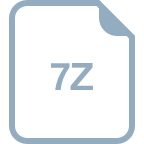











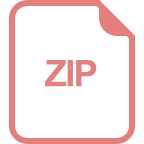
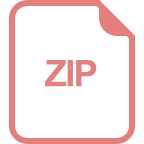