停用词表stopwords.txt 下载
时间: 2023-06-07 12:01:58 浏览: 421
在自然语言处理(Natural Language Processing, NLP)中,停用词(stopwords)是指那些在文本中出现频率很高但对文本的含义并没有太大贡献的单词。例如,英语中的“a”,“the”,“in”,“of”等。这些词汇对于文本的主旨理解没有贡献,却会占用计算资源、拖慢处理速度,甚至会影响结果的准确性,因此在文本分析中通常被去掉。
停用词表stopwords.txt是一个常见的存储英文停用词的文件。下载之后可以导入到NLP工具中,如Python中的NLTK库,然后在文本分析前进行去除。例如,假设有一句话:“The quick brown fox jumps over the lazy dog”,如果直接进行文本分析,停用词"a","the"和"over"等词汇会出现在结果中,对结果的准确性有所影响。但如果使用停用词表将这些词汇去掉,则分析的结果就会更加准确。
需要注意的是,停用词表是基于英文的,对于其他语言来说可能需要根据语言特点来制定相应的停用词表。同时,停用词表并非完美的,有时会误删一些具有特定情境含义的单词,因此在具体应用中还需要视情况选择是否使用停用词表。
相关问题
帮我写一个现成的停用词表stopwords.txt
当然可以,以下是一个常用的英文停用词表stopwords.txt:
```
a
an
and
are
as
at
be
but
by
for
if
in
into
is
it
no
not
of
on
or
such
that
the
their
then
there
these
they
this
to
was
will
with
```
如果你需要其他语言的停用词表,可以在网上搜索相关资源,或者告诉我需要哪种语言的停用词表,我可以帮你找到。
已知有用户自定义中文词典,名称为中文分词词典(作业一用).txt;有用户自定义停用词表stoplis.txt;有需要进行中文分词的测试样本,名为测试样本.TXT,现在需要对测试样本进行分词,分词结果写入作业(1).TXT。给出python代码
为了实现这个任务,你可以使用Python的jieba库来进行中文分词,并结合用户自定义的词典和停用词表。以下是一个示例代码:
```python
# 引入必要的库
import jieba
from collections import Counter
# 定义读取自定义词典和停用词的方法
def load_custom_dict(filename):
custom_dict = {}
with open(filename, 'r', encoding='utf-8') as f:
for line in f:
term, explanation = line.strip().split('\t')
custom_dict[term] = explanation
return custom_dict
def load_stopwords(filename):
stop_words = set()
with open(filename, 'r', encoding='utf-8') as f:
for word in f:
stop_words.add(word.strip())
return stop_words
# 加载词典和停用词
custom_dict = load_custom_dict('中文分词词典(作业一用).txt')
stop_words = load_stopwords('stoplis.txt')
# 对测试样本进行分词
with open('测试样本.TXT', 'r', encoding='utf-8') as test_file:
content = test_file.read()
# 使用自定义词典进行分词,并过滤掉停用词
custom_segmented_content = [word for word in jieba.cut(content, cut_all=False)
if word not in stop_words and word in custom_dict]
# 写入分词结果到新的文件
with open('作业(1).TXT', 'w', encoding='utf-8') as output_file:
for segment in custom_segmented_content:
output_file.write(segment + '\n')
```
这段代码首先加载了用户自定义的词典和停用词表,然后对测试样本的内容进行分词,只保留出现在词典中的非停用词。最后,将分词结果写入指定的输出文件。
阅读全文
相关推荐
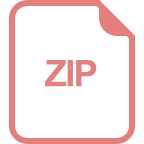
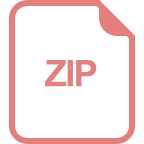
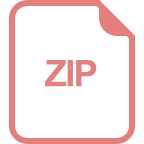
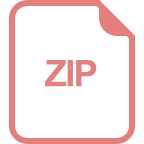
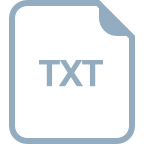
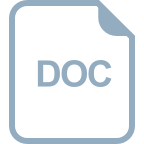
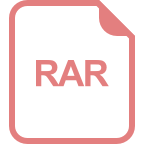
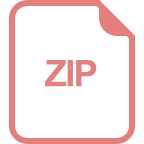



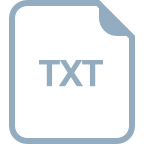
