for k in range(epochs):#k在第几次的循环中 i = np.random.randint(X.shape[0]) a = [X[i]] for l in range(len(self.weights)): #going forward network, for each layer a.append(self.activation(np.dot(a[l], self.weights[l]))) #Computer the node value for each layer (O_i) using activation function error = y[i] - a[-1] #Computer the error at the top layer deltas = [error * self.activation_deriv(a[-1])] #For output layer, Err calculation (delta is updated error) #Staring backprobagation for l in range(len(a) - 2, 0, -1): # we need to begin at the second to last layer #Compute the updated error (i,e, deltas) for each node going from top layer to input layer deltas.append(deltas[-1].dot(self.weights[l].T)*self.activation_deriv(a[l])) deltas.reverse() for i in range(len(self.weights)): layer = np.atleast_2d(a[i]) delta = np.atleast_2d(deltas[i]) self.weights[i] += learning_rate * layer.T.dot(delta) 代码含义
时间: 2023-02-12 11:23:16 浏览: 65
在Python中,"for k in range(epochs):" 意味着进行一个循环,循环变量 k 的值将在范围 [0, epochs) 中变化,每次循环都会执行其后面的语句。在这里,epochs 是一个变量或常量,表示循环的次数。
相关问题
for epoch in range(epochs): # 生成随机噪声 noise = np.random.normal(0, 1, size=(batch_size, latent_dim)) # 生成器生成假数据 fake_X = my_generator.predict(noise) # 随机选择真实数据 idx = np.random.randint(0, X_train.shape[0], batch_size) real_X = X_train[idx] # 训练判别器 discriminator.trainable = True discriminator.train_on_batch(real_X, np.ones((batch_size, 1))) discriminator.train_on_batch(fake_X, np.zeros((batch_size, 1))) # 训练生成器 discriminator.trainable = False gan.train_on_batch(noise, np.ones((batch_size, 1))) # 每 10 个 epoch 打印一次损失 if epoch % 10 == 0: print('Epoch %d: loss_d=%.4f, loss_g=%.4f' % (epoch, loss_d, loss_g))
在这段代码中,您正在使用一个GAN(生成对抗网络)来训练一个生成器和一个判别器。GAN是一种深度学习模型,用于生成逼真的假数据,它由两个部分组成:生成器和判别器。生成器负责生成假数据,判别器负责区分真实数据和假数据。
在每个epoch循环中,您正在执行以下操作:
1. 生成随机噪声。
2. 通过生成器生成假数据。
3. 随机选择真实数据。
4. 训练判别器,让它对真实数据和假数据进行分类。
5. 训练生成器,让它生成更逼真的假数据。
6. 每10个epoch打印一次损失。
请注意,此处的`my_generator`是一个生成器模型,用于生成假数据。在这段代码中,您正在使用`predict`方法来让生成器生成假数据。`real_X`表示从训练集中随机选择的真实数据。您还可以看到,判别器在训练假数据时使用0作为标签,而在训练真实数据时使用1作为标签。
当然,这段代码还缺少了一些关键部分,例如定义生成器和判别器模型,以及编译GAN模型。如果您需要完整的GAN代码示例,请参考相关教程或文档。
def train(generator, discriminator, gan, X_train, latent_dim, epochs=90, batch_size=70, loss_d=None, loss_g=None): for epoch in range(epochs): # 生成随机噪声 noise = np.random.normal(0, 1, size=(batch_size, latent_dim)) # 生成器生成假数据 fake_X = generator.predict(noise) # 随机选择真实数据 idx = np.random.randint(0, X_train.shape[0], batch_size) real_X = X_train[idx] # 训练判别器 discriminator.trainable = True discriminator.train_on_batch(real_X, np.ones((batch_size, 1))) discriminator.train_on_batch(fake_X, np.zeros((batch_size, 1))) # 训练生成器 discriminator.trainable = False gan.train_on_batch(noise, np.ones((batch_size, 1))) # 每 10 个 epoch 打印一次损失 if epoch % 10 == 0: print('Epoch %d: loss_d=%.4f, loss_g=%.4f' % (epoch, loss_d, loss_g))
这段代码是一个简单的 GAN(生成对抗网络)的训练过程,包括生成器、判别器和整个 GAN 的训练。其中,生成器用随机噪声生成假数据,判别器用于判别真实数据和假数据的真伪,并对两种数据进行训练。整个 GAN 的训练过程则是先训练判别器,再固定判别器的参数,训练生成器,使生成的假数据更接近于真实数据。每 10 个 epoch 打印一次损失。
相关推荐
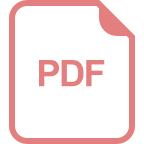
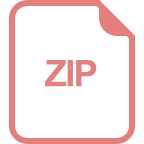
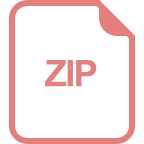












