首页import cv2 window_name = "Detected Objects in webcam" video = cv2.VideoCapture(0),这个VideoCapture函数为什么会是黄色
import cv2 window_name = "Detected Objects in webcam" video = cv2.VideoCapture(0),这个VideoCapture函数为什么会是黄色
时间: 2024-04-27 20:21:59 浏览: 75
`cv2.VideoCapture()` 函数被黄色标记可能是因为该函数未被正确导入。请确保你已经正确安装了 OpenCV 库,并且在代码的开头使用了正确的导入语句 `import cv2`。如果你已经正确导入了 OpenCV 库并且仍然看到黄色标记,则可能是你的 IDE 或文本编辑器无法正确解析该函数的定义。在这种情况下,你可以通过确保你的代码能够正常运行来验证该函数是否有效,或者尝试在其他 IDE 或编辑器中查看该函数是否被正确解析。
阅读全文
CSDN会员
开通CSDN年卡参与万元壕礼抽奖
最新推荐
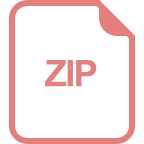
Android圆角进度条控件的设计与应用
资源摘要信息:"Android-RoundCornerProgressBar"
在Android开发领域,一个美观且实用的进度条控件对于提升用户界面的友好性和交互体验至关重要。"Android-RoundCornerProgressBar"是一个特定类型的进度条控件,它不仅提供了进度指示的常规功能,还具备了圆角视觉效果,使其更加美观且适应现代UI设计趋势。此外,该控件还可以根据需求添加图标,进一步丰富进度条的表现形式。
从技术角度出发,实现圆角进度条涉及到Android自定义控件的开发。开发者需要熟悉Android的视图绘制机制,包括但不限于自定义View类、绘制方法(如`onDraw`)、以及属性动画(Property Animation)。实现圆角效果通常会用到`Canvas`类提供的画图方法,例如`drawRoundRect`函数,来绘制具有圆角的矩形。为了添加图标,还需考虑如何在进度条内部适当地放置和绘制图标资源。
在Android Studio这一集成开发环境(IDE)中,自定义View可以通过继承`View`类或者其子类(如`ProgressBar`)来完成。开发者可以定义自己的XML布局文件来描述自定义View的属性,比如圆角的大小、颜色、进度值等。此外,还需要在Java或Kotlin代码中处理用户交互,以及进度更新的逻辑。
在Android中创建圆角进度条的步骤通常如下:
1. 创建自定义View类:继承自`View`类或`ProgressBar`类,并重写`onDraw`方法来自定义绘制逻辑。
2. 定义XML属性:在资源文件夹中定义`attrs.xml`文件,声明自定义属性,如圆角半径、进度颜色等。
3. 绘制圆角矩形:在`onDraw`方法中使用`Canvas`的`drawRoundRect`方法绘制具有圆角的进度条背景。
4. 绘制进度:利用`Paint`类设置进度条颜色和样式,并通过`drawRect`方法绘制当前进度覆盖在圆角矩形上。
5. 添加图标:根据自定义属性中的图标位置属性,在合适的时机绘制图标。
6. 通过编程方式更新进度:在Activity或Fragment中,使用自定义View的方法来编程更新进度值。
7. 实现动画:如果需要,可以通过Android的动画框架实现进度变化的动画效果。
标签中的"Android开发"表明,这些知识点和技能主要面向的是Android平台的开发人员。对于想要在Android应用中实现自定义圆角进度条的开发者来说,他们需要具备一定的Android编程基础,并熟悉相关的开发工具和库。
在"RoundCornerProgressBar-master"压缩包文件的文件名称列表中,我们可以推测这个资源包含了完整的项目代码,包括源代码、资源文件、布局文件、可能的示例代码以及必要的文档说明。开发者通过下载和解压缩这个包,可以得到一个完整的项目,从而可以直接查看代码实现细节,或是将其集成到自己的项目中。
最终,对于希望使用"Android-RoundCornerProgressBar"的开发者,关键在于理解自定义View的创建过程、圆角图形的绘制技术,以及如何在Android应用中集成和使用这些自定义控件。通过上述知识点的学习和实践,开发者能够掌握在Android应用中创建美观且功能丰富的用户界面所需的技能。

管理建模和仿真的文件
管理Boualem Benatallah引用此版本:布阿利姆·贝纳塔拉。管理建模和仿真。约瑟夫-傅立叶大学-格勒诺布尔第一大学,1996年。法语。NNT:电话:00345357HAL ID:电话:00345357https://theses.hal.science/tel-003453572008年12月9日提交HAL是一个多学科的开放存取档案馆,用于存放和传播科学研究论文,无论它们是否被公开。论文可以来自法国或国外的教学和研究机构,也可以来自公共或私人研究中心。L’archive ouverte pluridisciplinaire
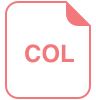
【R语言lattice包实战】:从案例到技巧,图形制作不再难

# 1. R语言与lattice包概述
R语言作为统计分析领域内广泛应用的编程语言,凭借其强大的图形功能而受到数据科学家的青睐。在R语言中,lattice包是绘图系统的重要组成部分,它基于trellis图形系统设计,能够高效地创建多变量条件图。本章节将引导读者对lattice包有一个初步的了解,并概述其功能和应用范围。
## 1.1 R语言中的图形系统
R语言提供了一系列的图形系统,基础图形系统(base graphics)是其

输入正整数n.打出长度为n的菱形
好的,以下是打印菱形的代码实现:
```
#include <stdio.h>
#include <stdlib.h>
int main() {
int n, i, j;
printf("请输入一个正整数n:");
scanf("%d", &n);
if(n % 2 == 0) { // 如果输入的n是偶数,将其加1变成奇数
n++;
}
for(i = 0; i < n / 2 + 1; i++) {
for(j = 0; j < n / 2 - i; j++) {
printf(
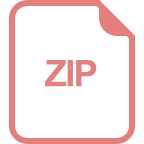
mui框架实现带侧边栏的响应式布局
资源摘要信息:"mui实现简单布局.zip"
mui是一个基于HTML5的前端框架,它采用了类似Bootstrap的语义化标签,但是专门为移动设备优化。该框架允许开发者使用Web技术快速构建高性能、可定制、跨平台的移动应用。此zip文件可能包含了一个用mui框架实现的简单布局示例,该布局具有侧边栏,能够实现首页内容的切换。
知识点一:mui框架基础
mui框架是一个轻量级的前端库,它提供了一套响应式布局的组件和丰富的API,便于开发者快速上手开发移动应用。mui遵循Web标准,使用HTML、CSS和JavaScript构建应用,它提供了一个类似于jQuery的轻量级库,方便DOM操作和事件处理。mui的核心在于其强大的样式表,通过CSS可以实现各种界面效果。
知识点二:mui的响应式布局
mui框架支持响应式布局,开发者可以通过其提供的标签和类来实现不同屏幕尺寸下的自适应效果。mui框架中的标签通常以“mui-”作为前缀,如mui-container用于创建一个宽度自适应的容器。mui中的布局类,比如mui-row和mui-col,用于创建灵活的栅格系统,方便开发者构建列布局。
知识点三:侧边栏实现
在mui框架中实现侧边栏可以通过多种方式,比如使用mui sidebar组件或者通过布局类来控制侧边栏的位置和宽度。通常,侧边栏会使用mui的绝对定位或者float浮动布局,与主内容区分开来,并通过JavaScript来控制其显示和隐藏。
知识点四:首页内容切换功能
实现首页可切换的功能,通常需要结合mui的JavaScript库来控制DOM元素的显示和隐藏。这可以通过mui提供的事件监听和动画效果来完成。开发者可能会使用mui的开关按钮或者tab标签等组件来实现这一功能。
知识点五:mui的文件结构
该压缩包文件包含的目录结构说明了mui项目的基本结构。其中,"index.html"文件是项目的入口文件,它将展示整个应用的界面。"manifest.json"文件是应用的清单文件,它在Web应用中起到了至关重要的作用,定义了应用的名称、版本、图标和其它配置信息。"css"文件夹包含所有样式表文件,"unpackage"文件夹可能包含了构建应用后的文件,"fonts"文件夹存放字体文件,"js"文件夹则是包含JavaScript代码的地方。
知识点六:mui的打包和分发
mui框架支持项目的打包和分发,开发者可以使用其提供的命令行工具来打包项目,生成可以部署到服务器的静态资源。这一步通常涉及到资源的压缩、合并和优化。打包后,开发者可以将项目作为一个Web应用分发,也可以将其打包为原生应用,比如通过Cordova、PhoneGap等工具打包成可在iOS或Android设备上安装的应用。
知识点七:mui的兼容性和性能优化
mui框架对老旧设备也做了兼容性考虑,保证应用在低端设备上也有较好的性能表现。性能优化方面,mui提供了多种工具和最佳实践,例如使用懒加载、避免全局变量污染、减少DOM操作等策略来提高应用的运行速度和用户体验。
以上内容是根据标题、描述以及文件名称列表推测出的关于mui实现简单布局的知识点。开发者可以通过分析和实践上述知识点来更好地理解和运用mui框架,从而构建出高效且用户友好的移动应用界面。

"互动学习:行动中的多样性与论文攻读经历"
多样性她- 事实上SCI NCES你的时间表ECOLEDO C Tora SC和NCESPOUR l’Ingén学习互动,互动学习以行动为中心的强化学习学会互动,互动学习,以行动为中心的强化学习计算机科学博士论文于2021年9月28日在Villeneuve d'Asq公开支持马修·瑟林评审团主席法布里斯·勒菲弗尔阿维尼翁大学教授论文指导奥利维尔·皮耶昆谷歌研究教授:智囊团论文联合主任菲利普·普雷教授,大学。里尔/CRISTAL/因里亚报告员奥利维耶·西格德索邦大学报告员卢多维奇·德诺耶教授,Facebook /索邦大学审查员越南圣迈IMT Atlantic高级讲师邀请弗洛里安·斯特鲁布博士,Deepmind对于那些及时看到自己错误的人...3谢谢你首先,我要感谢我的两位博士生导师Olivier和Philippe。奥利维尔,"站在巨人的肩膀上"这句话对你来说完全有意义了。从科学上讲,你知道在这篇论文的(许多)错误中,你是我可以依
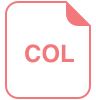
【交互式图形】:Shiny应用中lattice包的巧妙应用指南

# 1. Shiny与lattice包基础介绍
在数据科学与统计分析的世界中,可视化是一个至关重要的环节,它能够帮助我们直观地理解数据、展示研究成果并有效地传达信息。R语言作为数据分析领域的一个重要工具,其强大的图形能力得到了广泛的认可。在R语言家族中,`lattice`包和`Shiny`应用框架尤其受到关注。本章我们将简

安装包部署到docker中
安装包部署到Docker容器中通常包含以下几个步骤:
1. **准备镜像**:首先,你需要有一个基础的Dockerfile,这个文件描述了如何从基础镜像创建一个新的镜像,并在其中安装所需的软件和服务。例如,你可以基于官方的`alpine`或`ubuntu`等轻量级镜像开始。
```Dockerfile
# 使用官方的Python运行时作为基础镜像
FROM python:3.8-slim
# 设置工作目录
WORKDIR /app
# 将应用源码复制到容器内
COPY . .
# 安装依赖
RUN pip install -r requirements.txt
# 暴露端口
EXP
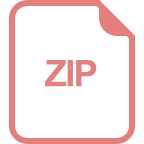
Android仿知乎横线直线进度条实现教程
资源摘要信息:"仿知乎的横线直线progressbar.zip是一个包含Android平台下自定义ProgressBar样式的资源文件包。该资源包可能包含了实现类似知乎应用中横线直线型进度条的源代码,用于在Android应用中提供用户界面的进度反馈。文件包的目的是帮助开发者学习和使用自定义的UI组件,同时促进技术交流。考虑到文件声明中提到了版权问题的免责声明,使用该资源时应确保遵守相关法律法规,尊重原作者的知识产权。"
知识点详细说明:
1. Android UI开发:
Android UI开发是指使用Android SDK提供的工具和API创建用户界面的过程。进度条(ProgressBar)是Android中用于展示任务进度的一种常见控件。在Android中,ProgressBar通常有两种形式:圆形和水平线性。开发者可以根据实际需要选择合适的样式,并且可以通过自定义来创建符合特定设计需求的进度条。
2. 自定义ProgressBar:
自定义ProgressBar涉及到对进度条控件外观和行为的修改。开发者可以通过修改ProgressBar的XML属性来自定义其样式,也可以通过重写其绘图方法来创建完全自定义的动画和图形效果。这通常需要一定的Android绘图知识,包括对Canvas、Drawable对象的操作等。
3. 横线直线型ProgressBar:
横线直线型ProgressBar是指进度条在显示时形状为水平的直线。这种样式在视觉上给人以直观的进度展示,适用于需要在界面上表现出线性增长或完成度的场景。这种ProgressBar的实现可能涉及到线性布局(LinearLayout)中子视图的动态更新,或者使用自定义的Drawable资源来绘制进度条的线条和填充。
4. Android源码学习:
Android源码学习指的是深入研究Android操作系统、应用框架和库的源代码。通过学习源码,开发者可以更好地理解Android系统的内部机制,提升软件架构设计和问题诊断的能力。源码学习也是一种了解和掌握新技术、新特性的有效途径。
5. 资源收集与整理:
资源收集与整理通常指的是将互联网上分散的有价值信息和资料汇总到一起,便于开发者学习和使用。在技术社区如CSDN,经常有开发者分享自己的学习心得、源码和教程。这些资源对于初学者和有经验的开发者来说都是非常宝贵的。
6. 知识产权和版权:
在进行技术学习和交流时,知识产权和版权问题非常关键。无论是从互联网上获取资源还是分享自己的作品,都应尊重原作者的版权,避免侵犯他人的知识产权。这通常意味着使用资源时应遵守相应的许可协议,并在必要时进行适当的署名或请求授权。
7. CSDN平台:
CSDN是中国的一个大型IT技术社区,提供技术文章发布、代码共享、资源下载等多种服务。该平台鼓励开发者分享知识、交流经验,并为开发者提供了一个互相学习、合作的社区环境。对于IT行业人士,CSDN是获取最新技术信息、解决方案和行业动态的重要渠道。

关系数据表示学习
关系数据卢多维奇·多斯桑托斯引用此版本:卢多维奇·多斯桑托斯。关系数据的表示学习机器学习[cs.LG]。皮埃尔和玛丽·居里大学-巴黎第六大学,2017年。英语。NNT:2017PA066480。电话:01803188HAL ID:电话:01803188https://theses.hal.science/tel-01803188提交日期:2018年HAL是一个多学科的开放存取档案馆,用于存放和传播科学研究论文,无论它们是否被公开。论文可以来自法国或国外的教学和研究机构,也可以来自公共或私人研究中心。L’archive ouverte pluridisciplinaireUNIVERSITY PIERRE和 MARIE CURIE计算机科学、电信和电子学博士学院(巴黎)巴黎6号计算机科学实验室D八角形T HESIS关系数据表示学习作者:Ludovic DOS SAntos主管:Patrick GALLINARI联合主管:本杰明·P·伊沃瓦斯基为满足计算机科学博士学位的要求而提交的论文评审团成员:先生蒂埃里·A·退休记者先生尤尼斯·B·恩