SQL 错误 [1010] [HY000]: Error dropping database (can't rmdir '.\qa_pfmea_2022', errno: 41) Error dropping database (can't rmdir '.\qa_pfmea_2022', errno: 41) Error dropping database (can't rmdir '.\qa_pfmea_2022', errno: 41)
时间: 2024-03-04 14:49:14 浏览: 21
这个错误是由于数据库无法删除导致的,可能是由于数据库文件正在被其他进程占用,或者数据库文件权限不足而导致无法删除。您可以尝试以下解决方法:
1. 检查数据库是否被其他进程占用,可以通过关闭其他应用程序或重启计算机来尝试解决该问题。
2. 检查数据库文件的权限,确保您有足够的权限来删除数据库文件。
3. 如果您使用的是 MySQL 数据库,可以尝试使用 DROP DATABASE IF EXISTS 命令来删除数据库:
```
DROP DATABASE IF EXISTS qa_pfmea_2022;
```
如果问题仍然存在,您可以尝试手动删除数据库文件或者联系数据库管理员来解决该问题。
相关问题
[err] [modeldatabase.cc:390] unable to parse model.config for model[http://gazebosim.org/models/bin_4_dropping_task]
这个错误提示是说 Gazebo 无法解析模型配置文件 model.config,具体是针对模型 http://gazebosim.org/models/bin_4_dropping_task 的。可能是配置文件格式有误或者文件本身损坏导致的。建议检查一下该模型的配置文件是否正确,并尝试重新下载或者重新安装该模型。
kernel: nf_conntrack: table full, dropping packet
这是一个内核消息,意味着在网络连接跟踪表中的条目数量超过了内核设置的限制。这可能是因为服务器正在处理太多的连接,或者因为设置的连接跟踪表大小太小。为了解决这个问题,您可以尝试增加连接跟踪表的大小并重新启动服务器,或者优化服务器以处理更少的连接。您还可以尝试使用连接跟踪表的其他工具,如IPset或Conntrackd,来帮助管理连接跟踪表。
相关推荐
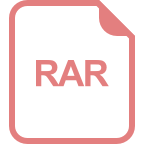












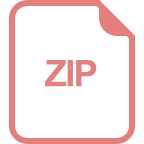