解释这段代码: int id = blockIdx.x * blockDim.x + threadIdx.x; int r = id / N;
时间: 2024-06-02 11:07:15 浏览: 178
这段代码是在GPU编程中使用的,其中blockIdx.x和blockDim.x是CUDA编程中的变量,分别表示当前线程块的索引和线程块中线程的数量。而threadIdx.x则表示当前线程在该线程块中的索引。这段代码的作用是计算线程的唯一编号id,使用这个编号可以对线程进行唯一的标识和索引。接着,根据id计算出r的值,其中N是一个常量,表示一个矩阵的大小。这段代码常用于矩阵运算相关的GPU并行计算中。
相关问题
#include <stdio.h> #include <cuda.h> // Kernel that executes on the CUDA device __global__ void square_array(float *a, int N) { int idx = blockIdx.x * blockDim.x + threadIdx.x; if (idx<N) a[idx] = a[idx] * a[idx]; } int main() { float *a_h, *a_d; // Pointer to host & device arrays const int N = 10; // Number of elements in arrays size_t size = N * sizeof(float); a_h = (float *)malloc(size); // Allocate array on host cudaMalloc((void **) &a_d, size); // Allocate array on device // Initialize host array and copy it to CUDA device for (int i=0; i<N; i++) a_h[i] = (float)i; cudaMemcpy(a_d, a_h, size, cudaMemcpyHostToDevice); // Do calculation on device: int block_size = 32; int n_blocks = N/block_size + (N%block_size == 0 ? 0:1); square_array <<< n_blocks, block_size >>> (a_d, N); // Retrieve result from device and store it in host array cudaMemcpy(a_h, a_d, sizeof(float)*N, cudaMemcpyDeviceToHost); // Print results for (int i=0; i<N; i++) printf("%d %f\n", i, a_h[i]); // Cleanup free(a_h); cudaFree(a_d); return 0; }
这段代码是一个简单的 CUDA 程序,它使用 GPU 加速了一个数组元素的平方计算。程序首先在 CPU 上分配了一个大小为 N 的浮点数数组 a_h,并初始化为 0 到 N-1 的整数。然后,它在 GPU 上分配了一个与 a_h 相同大小的浮点数数组 a_d,并将 a_h 的数据复制到 a_d。接下来,程序定义了一个名为 square_array 的 GPU 内核函数,该函数将执行实际的计算。此内核函数采用两个参数:指向 a_d 数组的指针和数组大小 N。在内核函数中,每个线程根据其在线程块中的索引和线程块的索引计算其在 a_d 数组中的索引,并将该位置的元素平方。最后,程序从 GPU 将计算结果复制回 a_h 数组,并打印出所有元素的平方值。
值得注意的是,要使用 CUDA 进行 GPU 编程,需要安装 CUDA 工具包并使用适当的编译器和链接器来构建程序。此外,还需要理解 GPU 内核函数和线程块之间的映射关系,以及如何管理内存和数据传输。
// cudaPi.cpp : Defines the entry point for the console application. // #include "stdafx.h" #include <stdio.h> #include <cuda.h> #include <math.h> #define NUM_THREAD 1024 #define NUM_BLOCK 1 __global__ void cal_pi(double *sum, long long nbin, float step, long long nthreads, long long nblocks) { long long i; float x; long long idx = blockIdx.x*blockDim.x+threadIdx.x; for (i=idx; i< nbin; i+=nthreads*nblocks) { x = (i+0.5)*step; sum[idx] = sum[idx]+4.0/(1.+x*x); } } int _tmain(int argc, _TCHAR* argv[]) { long long tid; double pi = 0; long long num_steps = 100000000; float step = 1./(float)num_steps; long long size = NUM_THREAD*NUM_BLOCK*sizeof(double); clock_t before, after; double *sumHost, *sumDev; sumHost = (double *)malloc(size); cudaMalloc((void **)&sumDev, size); // Initialize array in device to 0 cudaMemset(sumDev, 0, size); before = clock(); // Do calculation on device printf("Before Compute \n\n"); dim3 numBlocks(NUM_BLOCK,1,1); dim3 threadsPerBlock(NUM_THREAD,1,1); cal_pi <<<numBlocks, threadsPerBlock>>> (sumDev, (int)num_steps, step, NUM_THREAD, NUM_BLOCK); // call CUDA kernel printf("After Compute \n\n"); // Retrieve result from device and store it in host array cudaMemcpy(sumHost, sumDev, size, cudaMemcpyDeviceToHost); printf("After Copy \n\n"); for(tid=0; tid<NUM_THREAD*NUM_BLOCK; tid++){ pi = pi+sumHost[tid]; } pi = pi*step; after = clock(); printf("The value of PI is %15.12f\n",pi); printf("The time to calculate PI was %f seconds\n",((float)(after - before)/1000.0)); free(sumHost); cudaFree(sumDev); return 0; }
这段代码是用 CUDA 计算圆周率的程序。程序使用了 GPU 并行计算的能力,加快了计算速度。在主函数中,程序会先在主机端分配一个数组 sumHost,然后在设备端分配一个与之对应的数组 sumDev。然后程序会将 sumDev 数组清零,调用 cal_pi 函数执行并行计算,在执行过程中将计算结果存储到 sumDev 数组中。最后程序将 sumDev 数组中的结果拷贝到 sumHost 数组中,然后在主函数中将 sumHost 数组中的结果相加,计算出圆周率的值。程序最终输出计算得到的圆周率的值和计算所用的时间。
阅读全文
相关推荐
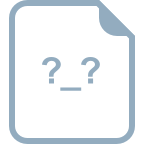
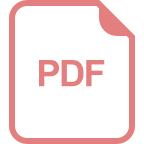
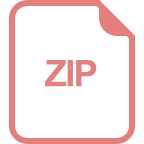


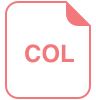
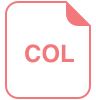









