def farthest_point_sample(xyz, npoint): """ Input: xyz: pointcloud data, [B, N, 3] npoint: number of samples Return: centroids: sampled pointcloud index, [B, npoint, 3] """ device = xyz.device B, N, C = xyz.shape centroids = torch.zeros(B, npoint, dtype=torch.long).to(device) # 采样点矩阵(B, npoint) distance = torch.ones(B, N).to(device) * 1e10 # 采样点到所有点距离(B, N) farthest = torch.randint(0, N, (B,), dtype=torch.long).to(device) # 最远点,初试时随机选择一点点 batch_indices = torch.arange(B, dtype=torch.long).to(device) # batch_size 数组 for i in range(npoint): centroids[:, i] = farthest # 更新第i个最远点 centroid = xyz[batch_indices, farthest, :].view(B, 1, 3) # 取出这个最远点的xyz坐标 dist = torch.sum((xyz - centroid) ** 2, -1) # 计算点集中的所有点到这个最远点的欧式距离 mask = dist < distance distance[mask] = dist[mask] # 更新distances,记录样本中每个点距离所有已出现的采样点的最小距离 farthest = torch.max(distance, -1)[1] # 返回最远点索引 return centroids 解释以上代码并说明需要的库
时间: 2024-04-27 19:21:41 浏览: 110
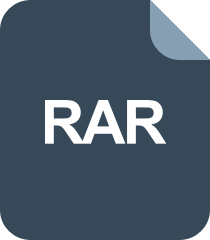
farthest point sampling

以上代码使用了PyTorch库来实现farthest point sampling(FPS)算法,用于从点云数据中均匀地采样一定数量的点。该算法首先随机选择一个点作为第一个采样点,然后计算所有点到这个采样点的距离,选择距离当前采样点最远的点作为下一个采样点,重复这个过程直到选出指定数量的采样点。
具体解释如下:
- 首先导入了PyTorch库,用于实现深度学习模型。
- farthest_point_sample函数中,xyz是输入的点云数据(B表示batch_size,N表示点云中点的数量,C表示每个点的坐标),npoint是需要采样的点的数量。
- 创建一个空的采样点矩阵centroids,用于存储选中的采样点的索引。
- 初始化距离矩阵distance,将其设置为一个非常大的值,用于存储每个点到所有已选采样点的最小距离。
- 随机选择一个点作为第一个采样点,将其赋值给变量farthest。
- 创建一个batch_size数组batch_indices,用于指定每个点所属的batch。
- 循环npoint次,每次选出距离当前采样点最远的点作为下一个采样点。
- 将当前采样点的索引farthest存储到采样点矩阵centroids中。
- 从点云数据xyz中取出当前采样点的坐标centroid。
- 计算所有点到当前采样点的欧式距离dist。
- 根据距离更新距离矩阵distance,记录样本中每个点距离所有已出现的采样点的最小距离。
- 选出距离所有已选采样点最远的点作为下一个采样点,更新变量farthest。
- 最终返回采样点矩阵centroids。
需要使用的库是PyTorch。
阅读全文
相关推荐
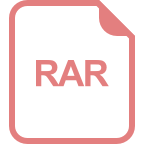
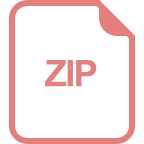
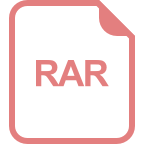
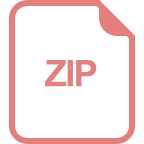
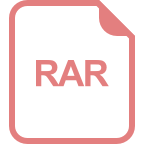
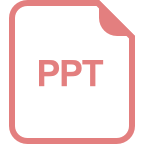
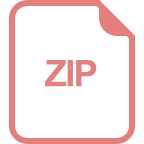










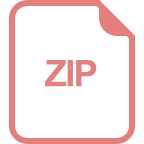