cluster p值聚类
时间: 2023-08-13 22:05:52 浏览: 105
cluster p值聚类是一种常见的聚类分析方法,主要用于生物信息学领域中的基因表达数据分析。该方法通过计算每个基因在不同样本之间的表达水平差异,然后根据这些差异的p值进行聚类分析。具体来说,该方法首先计算每个基因在不同样本中的表达水平,然后使用统计学方法比较不同样本之间的表达水平差异,得到每个基因的p值,即差异显著性水平。然后,将所有基因按照p值从小到大进行排序,将p值较小的基因聚在一起,形成一个聚类簇。这个过程不断重复,直到所有基因都被聚类到一个簇中。最终得到的结果是一棵聚类树,树的每个节点代表一个聚类簇,树的叶子节点代表每个基因。cluster p值聚类方法可以帮助研究人员快速发现在不同条件下表达差异显著的基因,从而进一步分析这些基因的生物学功能和调控机制。
相关问题
kmean聚类matlab
在MATLAB中,可以使用自带的kmeans函数进行k-means聚类。首先,使用kmeans函数将数据分成指定数量的簇,例如cluster_num=3,即将数据分成3个簇。然后,可以使用unique函数找出分类出的个数,并使用cell数组存储每个簇中的数据索引。接下来,遍历每个簇,获取对应的数据并使用scatter函数绘制散点图表示每个簇的数据。同时,可以使用plot函数绘制簇的中心点。最后,可以计算轮廓系数(SC)来评估聚类效果。轮廓系数可以使用mean函数计算silhouette函数返回的值。整个过程的代码示例如下:
```
cluster_num=3; %自定义分类数
[index_km,center_km]=kmeans(data,cluster_num); %MATLAB自带kmeans函数
a=unique(index_km); %找出分类出的个数
C=cell(1,length(a));
for i=1:length(a)
C(1,i)={find(index_km==a(i))};
end
figure
subplot(2,1,2)
for j=1:cluster_num
data_get=data(C{1,j},:);
scatter(data_get(:,1),data_get(:,2),100,'filled','MarkerFaceAlpha',.6,'MarkerEdgeAlpha',.9);
hold on
end
plot(center_km(:,1),center_km(:,2),'kd','LineWidth',2);
hold on
sc_k=mean(silhouette(data,index_km));
title_str1=['MATLAB自带kmeans函数',' 聚类数为:',num2str(cluster_num),' SC轮廓系数:',num2str(sc_k)];
title(title_str1);
```
可以在MATLAB中运行以上代码来进行k-means聚类,并可视化聚类结果。
此外,您还可以参考GitHub的一个示例代码,链接如下:[https://github.com/Qyokizzzz/AI-Algorithm/tree/master/K-means](https://github.com/Qyokizzzz/AI-Algorithm/tree/master/K-means) 。
另外,您还可以通过分析数据,选择最佳的k值进行聚类。例如,可以加载fisheriris数据集,并使用其meas的前两个维度来进行聚类。以下是一个示例脚本:
```
load fisheriris
data = normalization(meas);
[idx,C,~ = Kmeans(data(:,1:2),3,0,5000);
figure
gscatter(data(:,1),data(:,2),idx,'bgm')
hold on
plot(C(:,1),C(:,2),'kx')
legend('Cluster 1','Cluster 2','Cluster 3','ClusterCentroid')
```
上述代码将fisheriris数据集进行了归一化处理,并使用Kmeans函数将数据聚类成3个簇。通过gscatter函数绘制散点图表示不同簇的数据,并使用plot函数绘制簇的中心点。
最后,您还可以编写一个计算"有序属性"距离的函数来计算样本点之间的闵可夫斯基距离。例如,可以使用cal_dist函数计算两个样本点之间的欧氏距离或曼哈顿距离。以下是一个示例代码:
```
function dist = cal_dist(X,p)
dim = size(X);
sum = 0;
for i = 1:dim(2)
sum = sum + abs(X(1,i)-X(2,i))^p;
end
dist = sum^(1/p);
end
```
该函数可以根据指定的p值计算两个样本点之间的闵可夫斯基距离,其中p=2为欧氏距离,p=1为曼哈顿距离。<span class="em">1</span><span class="em">2</span><span class="em">3</span><span class="em">4</span>
给定100个二维随机坐标点,针对X坐标对坐标进行聚类,聚类阈值最小值为1,聚类阈值最大值为5,如果X坐标间的距离处于阈值范围内,则按密度情况对X坐标进行聚类,聚类情况用不同颜色标记出来,并把些点用不同的聚类颜色显示在窗体上,请给出以上的C#代码和详细注释
首先,我们需要定义一个二维坐标点的类:
```
public class Point2D
{
public double X { get; set; }
public double Y { get; set; }
}
```
接下来,我们定义一个聚类器的类,用于对坐标进行聚类:
```
public class Clustering
{
private double _minDistance;
private double _maxDistance;
public Clustering(double minDistance, double maxDistance)
{
_minDistance = minDistance;
_maxDistance = maxDistance;
}
public List<List<Point2D>> Cluster(List<Point2D> points)
{
List<List<Point2D>> clusters = new List<List<Point2D>>();
foreach (Point2D point in points)
{
bool added = false;
foreach (List<Point2D> cluster in clusters)
{
if (cluster.Any(p => Math.Abs(p.X - point.X) <= _maxDistance))
{
cluster.Add(point);
added = true;
break;
}
}
if (!added)
{
List<Point2D> newCluster = new List<Point2D>();
newCluster.Add(point);
clusters.Add(newCluster);
}
}
return clusters;
}
}
```
在聚类器的构造函数中,我们传入了最小和最大聚类距离。在 `Cluster` 方法中,我们遍历每个点,并检查它是否可以被添加到已有的聚类中。如果一个点的 X 坐标和某个聚类中的任一点的 X 坐标之差小于等于最大距离,则可以将该点添加到该聚类中。否则,我们将该点添加到一个新聚类中。
最后,我们可以在窗体上绘制聚类后的点:
```
private void DrawClusters(List<List<Point2D>> clusters)
{
Graphics g = pictureBox1.CreateGraphics();
Random random = new Random();
foreach (List<Point2D> cluster in clusters)
{
Color color = Color.FromArgb(random.Next(256), random.Next(256), random.Next(256));
Brush brush = new SolidBrush(color);
foreach (Point2D point in cluster)
{
int x = (int)point.X;
int y = (int)point.Y;
g.FillEllipse(brush, x, y, 5, 5);
}
}
}
```
在 `DrawClusters` 方法中,我们遍历每个聚类,并为每个聚类生成一个随机颜色。然后,我们遍历聚类中的每个点,并将其绘制为一个半径为 2.5 像素的圆。注意,我们使用了 `pictureBox1` 控件来绘制图形。
最后,我们可以在主函数中使用聚类器进行聚类,并调用 `DrawClusters` 方法来在窗体上显示聚类后的点:
```
List<Point2D> points = new List<Point2D>();
// 假设我们已经有了100个二维坐标点
Clustering clustering = new Clustering(1, 5);
List<List<Point2D>> clusters = clustering.Cluster(points);
DrawClusters(clusters);
```
阅读全文
相关推荐






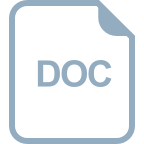










