LinearSVC和LogisticRegression的区别
时间: 2024-05-19 18:17:15 浏览: 171
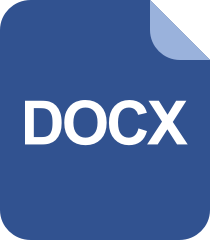
logistic模型预测人口.docx
LinearSVC和LogisticRegression都是常用的二分类算法,但是它们有一些区别:
1. 模型形式不同:LinearSVC使用支持向量机(SVM)的思想,采用线性核函数,将数据投影到高维空间中进行分类;而LogisticRegression使用逻辑斯蒂回归模型,通过对样本数据的特征进行线性组合得到分类结果。
2. 损失函数不同:LinearSVC使用的是hinge loss函数,而LogisticRegression使用的是logistic loss函数。
3. 输出结果不同:LinearSVC输出的是一个分类结果,即数据点属于哪一类;而LogisticRegression输出的是概率值,表示数据点属于某一类的概率。
4. 对异常值的处理不同:LinearSVC对异常值比较敏感,容易受到异常值的影响;而LogisticRegression对异常值比较鲁棒,可以一定程度上减弱异常值的影响。
5. 计算速度不同:LinearSVC的计算速度比LogisticRegression快,尤其是在大规模数据集上表现更加优秀。
阅读全文
相关推荐
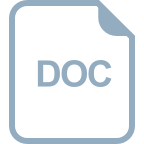
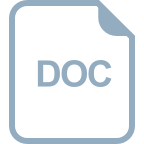





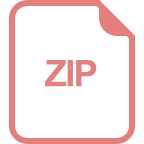
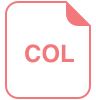


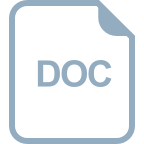
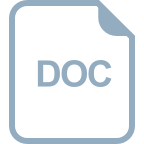
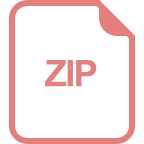
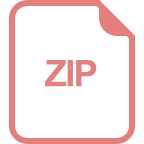
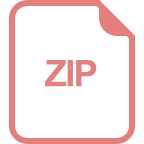
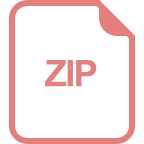