Apply SVM model using Linear Kernel function
时间: 2023-12-06 19:39:39 浏览: 26
Sure, here are the steps to apply SVM model using Linear Kernel function:
1. Load the dataset and split it into training and testing sets.
2. Preprocess the data by scaling or normalizing the features.
3. Import the SVM module from scikit-learn.
4. Create an instance of the SVM model with the Linear Kernel function.
5. Train the model on the training set using the fit() method.
6. Predict the output for the testing set using the predict() method.
7. Evaluate the model's performance by computing metrics such as accuracy, precision, recall, and F1 score.
Here's some sample code to illustrate the process:
```python
from sklearn import datasets
from sklearn.model_selection import train_test_split
from sklearn.preprocessing import StandardScaler
from sklearn.svm import SVC
from sklearn.metrics import accuracy_score
# Load the Iris dataset
iris = datasets.load_iris()
X = iris.data
y = iris.target
# Split the data into training and testing sets
X_train, X_test, y_train, y_test = train_test_split(X, y, test_size=0.2, random_state=42)
# Preprocess the data by scaling the features
scaler = StandardScaler()
X_train = scaler.fit_transform(X_train)
X_test = scaler.transform(X_test)
# Create an SVM model with Linear Kernel function
svm = SVC(kernel='linear')
# Train the model on the training set
svm.fit(X_train, y_train)
# Predict the output for the testing set
y_pred = svm.predict(X_test)
# Evaluate the model's performance
accuracy = accuracy_score(y_test, y_pred)
print("Accuracy:", accuracy)
```
This code applies an SVM model with the Linear Kernel function to the Iris dataset, which is a multiclass classification problem. The code preprocesses the data by scaling the features using StandardScaler, trains the model on the training set using the fit() method, predicts the output for the testing set using the predict() method, and evaluates the model's performance using the accuracy_score() function.
相关推荐
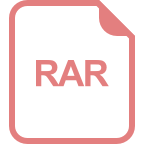














