怎么在resnet50加入cbam
时间: 2023-11-27 19:05:38 浏览: 112
要在ResNet-50中添加CBAM(Convolutional Block Attention Module),你需要对ResNet-50的基本结构进行修改。
首先,导入所需的库和模块:
```python
import torch
import torch.nn as nn
import torch.nn.functional as F
```
接下来,定义CBAM模块的两个子模块:通道注意力模块(Channel Attention Module)和空间注意力模块(Spatial Attention Module)。
```python
class ChannelAttention(nn.Module):
def __init__(self, in_planes, ratio=16):
super(ChannelAttention, self).__init__()
self.avg_pool = nn.AdaptiveAvgPool2d(1)
self.max_pool = nn.AdaptiveMaxPool2d(1)
self.fc1 = nn.Conv2d(in_planes, in_planes // ratio, 1, bias=False)
self.relu1 = nn.ReLU()
self.fc2 = nn.Conv2d(in_planes // ratio, in_planes, 1, bias=False)
def forward(self, x):
avg_out = self.fc2(self.relu1(self.fc1(self.avg_pool(x))))
max_out = self.fc2(self.relu1(self.fc1(self.max_pool(x))))
out = avg_out + max_out
return torch.sigmoid(out)
class SpatialAttention(nn.Module):
def __init__(self, kernel_size=7):
super(SpatialAttention, self).__init__()
assert kernel_size in (3, 7), 'kernel size must be 3 or 7'
padding = 3 if kernel_size == 7 else 1
self.conv1 = nn.Conv2d(2, 1, kernel_size, padding=padding, bias=False)
def forward(self, x):
avg_out = torch.mean(x, dim=1, keepdim=True)
max_out, _ = torch.max(x, dim=1, keepdim=True)
x = torch.cat([avg_out, max_out], dim=1)
x = self.conv1(x)
return torch.sigmoid(x)
```
然后,定义包含CBAM模块的ResNet-50网络。
```python
class CBAMResNet(nn.Module):
def __init__(self, num_classes=1000):
super(CBAMResNet, self).__init__()
self.in_planes = 64
# ResNet-50 layers (without fully connected layers)
self.conv1 = nn.Conv2d(3, 64, kernel_size=7, stride=2, padding=3, bias=False)
self.bn1 = nn.BatchNorm2d(64)
self.relu = nn.ReLU(inplace=True)
self.maxpool = nn.MaxPool2d(kernel_size=3, stride=2, padding=1)
self.layer1 = self._make_layer(64, 3)
self.layer2 = self._make_layer(128, 4, stride=2)
self.layer3 = self._make_layer(256, 6, stride=2)
self.layer4 = self._make_layer(512, 3, stride=2)
# CBAM attention modules
self.channel_att = ChannelAttention(512)
self.spatial_att = SpatialAttention()
# Fully connected layer
self.avgpool = nn.AdaptiveAvgPool2d(1)
self.fc = nn.Linear(512, num_classes)
def _make_layer(self, planes, blocks, stride=1):
downsample = None
if stride != 1 or self.in_planes != planes * 4:
downsample = nn.Sequential(
nn.Conv2d(self.in_planes, planes * 4, kernel_size=1, stride=stride, bias=False),
nn.BatchNorm2d(planes * 4)
)
layers = []
layers.append(Bottleneck(self.in_planes, planes, stride, downsample))
self.in_planes = planes * 4
for _ in range(1, blocks):
layers.append(Bottleneck(self.in_planes, planes))
return nn.Sequential(*layers)
def forward(self, x):
x = self.conv1(x)
x = self.bn1(x)
x = self.relu(x)
x = self.maxpool(x)
x = self.layer1(x)
x = self.layer2(x)
# Apply CBAM attention modules
x = self.channel_att(x) * x
x = self.spatial_att(x) * x
x = self.layer3(x)
x = self.layer4(x)
x = self.avgpool(x)
x = x.view(x.size(0), -1)
x = self.fc(x)
return x
```
相关推荐
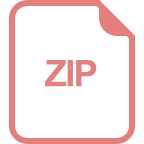
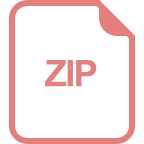













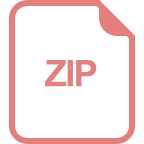
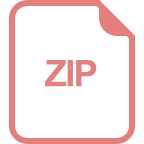