高斯核函数的python代码
时间: 2023-12-15 11:30:45 浏览: 35
高斯核函数是一种常用的核函数,它可以将数据映射到高维空间中,从而更好地进行分类或回归。以下是高斯核函数的Python代码:
```python
import numpy as np
from scipy.spatial.distance import pdist, squareform
def gaussian_kernel(X, sigma=1):
"""
高斯核函数
:param X: 输入数据,形状为(n_samples, n_features)
:param sigma: 高斯核函数的参数
:return: 高斯核矩阵,形状为(n_samples, n_samples)
"""
pairwise_dists = squareform(pdist(X, 'euclidean'))
K = np.exp(-pairwise_dists ** 2 / (2 * sigma ** 2))
return K
```
其中,`X`是输入数据,`sigma`是高斯核函数的参数。`pairwise_dists`是输入数据两两之间的欧几里得距离,`K`是高斯核矩阵,它的每个元素都是两个样本之间的相似度。
相关问题
高斯核函数python代码
高斯核函数也称为径向基函数(RBF)核函数,是常用的核函数之一,它在机器学习中被广泛应用。以下是实现高斯核函数的 Python 代码:
```python
import numpy as np
def gaussian_kernel(x1, x2, sigma):
"""
Computes the Gaussian kernel between two vectors x1 and x2.
Parameters:
x1 -- array_like, shape (n, )
x2 -- array_like, shape (n, )
sigma -- float, parameter for the Gaussian kernel
Returns:
sim -- float, similarity score
"""
# Convert to numpy arrays if necessary
x1, x2 = np.array(x1), np.array(x2)
# Compute the squared Euclidean distance between the two vectors
dist_squared = np.sum((x1 - x2) ** 2)
# Compute the Gaussian kernel
sim = np.exp(-dist_squared / (2 * sigma ** 2))
return sim
```
其中,x1 和 x2 是两个向量,sigma 是高斯核函数的参数。函数返回两个向量之间的相似度得分。
构建高斯核函数python代码
高斯核函数(也称为径向基函数)是一种常用的核函数,用于支持向量机(SVM)等机器学习算法中的非线性分类和回归任务。下面是一个简单的Python代码示例,用于构建高斯核函数:
```python
import numpy as np
def gaussian_kernel(x1, x2, sigma):
"""
高斯核函数计算
:param x1: 向量1
:param x2: 向量2
:param sigma: 高斯核函数的标准差
:return: 高斯核函数的值
"""
return np.exp(-np.linalg.norm(x1 - x2) ** 2 / (2 * (sigma ** 2)))
# 示例使用
x1 = np.array([1, 2, 3])
x2 = np.array([4, 5, 6])
sigma = 1.0
result = gaussian_kernel(x1, x2, sigma)
print(result)
```
在上述代码中,`gaussian_kernel`函数接受两个向量`x1`和`x2`,以及高斯核函数的标准差`sigma`作为参数。它使用NumPy库计算两个向量之间的欧氏距离,并将其作为指数的指数部分。最后,通过调用`np.exp`函数计算高斯核函数的值。
相关推荐
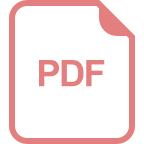
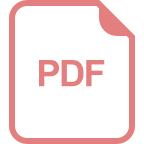
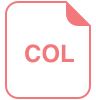
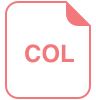










