class Stack:#堆栈 class Node: def __init__(self, val, next=None): self.val = val self.next = next def __init__(self): self.top = None def push(self, val): self.top = Stack.Node(val, self.top) def pop(self): assert self.top, 'Stack is empty' val = self.top.val self.top = self.top.next return val def peek(self): return self.top.val if self.top else None def empty(self): return self.top == None def __bool__(self): return not self.empty() def __repr__(self): if not self.top: return '' return '--> ' + ', '.join(str(x) for x in self) def __iter__(self): n = self.top while n: yield n.val n = n.next
时间: 2024-02-14 22:20:49 浏览: 35
这是一个简单的堆栈实现,使用Python语言编写。堆栈是一种常见的数据结构,它具有后进先出(LIFO)的特点。这个堆栈类包含了Node类,用于创建节点,以及push、pop、peek、empty等方法,用于操作堆栈。其中push方法用于将元素压入堆栈,pop方法用于从堆栈中弹出元素并返回,peek方法用于获取堆栈顶部的元素,empty方法用于判断堆栈是否为空。此外,还实现了__bool__和__repr__方法,用于判断堆栈是否为空,并以字符串形式表示堆栈中的元素。
相关问题
class AvgMeter(object): def __init__(self, num=40): self.num = num self.reset() def reset(self): self.val = 0 self.avg = 0 self.sum = 0 self.count = 0 self.losses = [] def update(self, val, n=1): self.val = val self.sum += val * n self.count += n self.avg = self.sum / self.count self.losses.append(val) def show(self): return torch.mean(torch.stack(self.losses[np.maximum(len(self.losses)-self.num, 0):]))
这段代码定义了一个名为"AvgMeter"的类,用于计算平均值和记录损失值。它具有以下方法:
- `__init__(self, num=40)`:类的构造函数,用于初始化对象。接受一个可选参数num,表示要计算平均值时使用的损失值的数量,默认为40。
- `reset(self)`:重置计算器的状态,将所有值重置为初始状态。
- `update(self, val, n=1)`:更新计算器的状态,接受两个参数val和n,分别表示要更新的值和更新的次数。通过累加val * n来更新计算器的总和sum、计数count和平均值avg,并将val添加到losses列表中。
- `show(self)`:计算并返回最近num个损失值的平均值。它首先使用切片操作从losses列表中选择最近的num个值,然后使用torch.stack将它们堆叠成一个张量,最后使用torch.mean计算平均值。
这个类可以用于跟踪和计算损失函数的平均值,并提供了一个用于展示最近损失值平均值的方法。
分析代码:class TreeNode: def __init__(self, val): self.val = val self.left = None self.right = None def build_tree(postorder): if not postorder: return None root = TreeNode(postorder[-1]) i = 0 while i < len(postorder) - 1 and postorder[i] < root.val: i += 1 root.left = build_tree(postorder[:i]) root.right = build_tree(postorder[i:-1]) return root def preorder_traversal(root): if not root: return [] stack, res = [root], [] while stack: node = stack.pop() res.append(node.val) if node.right: stack.append(node.right) if node.left: stack.append(node.left) return res # 测试代码 postorder = [1, 3, 2, 5, 7, 6, 4] root = build_tree(postorder) print(preorder_traversal(root))
这段 Python 代码实现了根据二叉搜索树的后序遍历序列构建二叉搜索树,并输出其先序遍历序列。以下是代码的分析:
1. 定义了一个 `TreeNode` 类,表示二叉树的节点,包含一个值属性 `val` 和两个指针属性 `left` 和 `right`,分别指向左子树和右子树。
2. `build_tree` 函数用于根据输入的后序遍历序列 `postorder` 构建二叉搜索树,返回根节点。如果 `postorder` 为空,则返回 `None`。
3. 在 `build_tree` 函数中,首先根据 `postorder` 的最后一个元素创建根节点 `root`。然后找到第一个大于等于根节点值的元素的下标 `i`,将 `postorder` 分为左右两个部分,左部分为该节点的左子树的后序遍历序列,右部分为该节点的右子树的后序遍历序列。递归调用 `build_tree` 函数构建左子树和右子树,并将它们分别设为 `root` 的左孩子和右孩子。
4. `preorder_traversal` 函数用于输出二叉搜索树的先序遍历序列。如果输入的根节点 `root` 为空,则返回一个空列表。
5. 在 `preorder_traversal` 函数中,使用栈来实现先序遍历,将根节点压入栈中,并在每次出栈时将当前节点的值加入结果列表 `res` 中。同时,依次将当前节点的右孩子和左孩子压入栈中,保证先遍历左子树,再遍历右子树。
6. 最后,通过输入一个后序遍历序列 `postorder`,调用 `build_tree` 函数构建二叉搜索树,并将根节点传入 `preorder_traversal` 函数,输出先序遍历序列。
综上所述,这段 Python 代码实现了根据二叉搜索树的后序遍历序列构建二叉搜索树,并输出其先序遍历序列的功能。
相关推荐
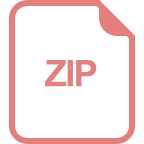
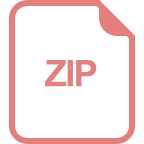
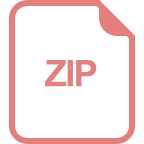













