用pytorch写一个使用了nn.Softmax的代码
时间: 2024-03-16 12:42:10 浏览: 131
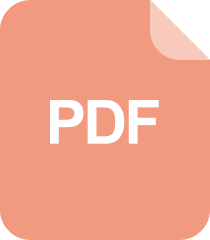
Pytorch损失函数nn.NLLLoss2d()用法说明
下面是一个使用PyTorch实现softmax分类的示例代码,其中使用了`nn.Softmax`:
```
import torch
import torch.nn as nn
import torch.optim as optim
# 构建数据集
data = [[1, 2, 1, 1],
[2, 1, 3, 2],
[3, 1, 3, 4],
[4, 1, 5, 5],
[1, 7, 5, 5],
[1, 2, 5, 6],
[1, 6, 6, 6],
[1, 7, 7, 7]]
# 构建标签
labels = [2, 2, 2, 1, 1, 1, 0, 0]
num_classes = 3
# 转换为Tensor
x_train = torch.FloatTensor(data)
y_train = torch.LongTensor(labels)
# 定义模型
class SoftmaxClassifier(nn.Module):
def __init__(self):
super(SoftmaxClassifier, self).__init__()
self.linear = nn.Linear(4, num_classes)
self.softmax = nn.Softmax(dim=1) # 在第二个维度上进行softmax
def forward(self, x):
out = self.linear(x)
out = self.softmax(out)
return out
model = SoftmaxClassifier()
# 定义损失函数和优化器
criterion = nn.CrossEntropyLoss()
optimizer = optim.SGD(model.parameters(), lr=0.1)
# 训练模型
num_epochs = 1000
for epoch in range(num_epochs):
optimizer.zero_grad()
outputs = model(x_train)
loss = criterion(outputs, y_train)
loss.backward()
optimizer.step()
if (epoch+1) % 100 == 0:
print('Epoch [{}/{}], Loss: {:.4f}'.format(epoch+1, num_epochs, loss.item()))
# 测试模型
model.eval()
test_data = torch.FloatTensor([[1, 2, 1, 1]])
with torch.no_grad():
outputs = model(test_data)
_, predicted = torch.max(outputs.data, 1)
print('Predicted class:', predicted.item())
```
该代码实现了一个简单的softmax分类器,使用交叉熵损失函数进行训练,并使用随机梯度下降进行优化。在模型的`forward`方法中,使用了`nn.Softmax`对模型的输出进行了softmax操作。在测试阶段,输入一个新的数据点进行分类预测。
阅读全文
相关推荐
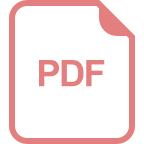
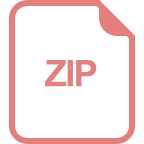















