cvtColor(model_, gray_model, cv::COLOR_RGB2GRAY);
时间: 2023-06-25 19:08:25 浏览: 55
这段代码是使用OpenCV库中的cvtColor函数将RGB格式的图像转换成灰度图像。具体来说,它将一个三通道(R、G、B)的图像转换成一个单通道(灰度)的图像。
第一个参数是原始的RGB图像,第二个参数是转换后的灰度图像,第三个参数是转换的类型,这里使用的是COLOR_RGB2GRAY,表示将RGB图像转换成灰度图像。
需要注意的是,这段代码中使用的model_和gray_model分别表示原始的RGB图像和转换后的灰度图像的变量名,具体命名可以根据具体情况进行修改。
相关问题
import cv2 import numpy as np import tensorflow as tf # 加载之前训练好的模型 model = tf.keras.models.load_model('mnist_cnn_model') for img in images_data: # 将RGB格式转换为BGR格式 img_bgr = cv2.cvtColor(img, cv2.COLOR_RGB2BGR) # 转换为灰度图像 gray = cv2.cvtColor(img_bgr, cv2.COLOR_BGR2GRAY) # 二值化处理 _, thresh = cv2.threshold(gray, 0, 255, cv2.THRESH_BINARY_INV+cv2.THRESH_OTSU) # 找到轮廓 contours, hierarchy = cv2.findContours(thresh, cv2.RETR_EXTERNAL, cv2.CHAIN_APPROX_SIMPLE) # 初始化计数器 count = 0 # 遍历所有轮廓 for contour in contours: # 计算轮廓面积 area = cv2.contourArea(contour) if area < 200 or area > 2000: # 如果轮廓面积小于10个像素,则忽略该轮廓 continue # 获取轮廓的外接矩形 x, y, w, h = cv2.boundingRect(contour) # 在原始图像上标记出抠出来的数字部分,并将BGR格式转换为RGB格式 cv2.rectangle(img_bgr, (x, y), (x+w, y+h), (0, 255, 0), 2) digit = cv2.cvtColor(img_bgr[y:y+h, x:x+w], cv2.COLOR_BGR2RGB) # 对数字图像进行预处理,使其与训练数据具有相同的格式 digit_resized = cv2.resize(digit, (28, 28)) digit_gray = cv2.cvtColor(digit_resized, cv2.COLOR_RGB2GRAY) digit_normalized = digit_gray / 255.0 digit_reshaped = np.reshape(digit_normalized, (1, 28, 28)) # 进行预测并输出最大概率对应的数字 prediction = model.predict(digit_reshaped) digit_class = np.argmax(prediction) print("抠出来的数字是:", digit_class) # 增加计数器 count += 1 # 在原始图像上显示标记过的抠出来的数字部分 #plt.figure(figsize=(20, 20)) #plt.imshow(cv2.cvtColor(img_bgr, cv2.COLOR_BGR2RGB)) #plt.show() # 显示抠出来的数字个数 print("抠出来了{}个数字".format(count)) 如何解决error Traceback (most recent call last) ~\AppData\Local\Temp/ipykernel_12928/408549256.py in <module> 8 for img in images_data: 9 # 将RGB格式转换为BGR格式 ---> 10 img_bgr = cv2.cvtColor(img, cv2.COLOR_RGB2BGR) 11 12 # 转换为灰度图像 error: OpenCV(4.7.0) :-1: error: (-5:Bad argument) in function 'cvtColor' > Overload resolution failed: > - src is not a numpy array, neither a scalar > - Expected Ptrcv::UMat for argument 'src'这个错误
根据错误提示,`cvtColor`函数的`src`参数不是一个numpy数组或标量。检查一下`images_data`中的图片格式是否正确。`images_data`中的图片应该是numpy数组格式,如果不是,你需要将其转换为numpy数组格式才能进行后续的操作。你可以使用`np.array()`函数将图片数据转换为numpy数组格式。代码示例如下:
```
images_data = [np.array(image) for image in images_data]
```
import dlib import numpy as np import cv2 predictor_model = 'shape_predictor_68_face_landmarks.dat' detector = dlib.get_frontal_face_detector() predictor = dlib.shape_predictor(predictor_model) img1 = cv2.imread('f.jpg') img_gray = cv2.cvtColor(img1, cv2.COLOR_RGB2GRAY) rects = detector(img_gray, 0) print(rects[0]) for i in range(len(rects)): landmarks = np.matrix([[p.x, p.y] for p in predictor(img1, rects[i]).parts()]) print(landmarks, type(landmarks)) for idx, point in enumerate(landmarks): pos = (point[0, 0], point[0, 1]) cv2.circle(img1, pos, 3, color=(0, 255, 0)) font = cv2.FONT_HERSHEY_SIMPLEX cv2.putText(img1, str(idx + 1), pos, font, 0.5, (0, 0, 255), 1, cv2.LINE_AA) cv2.imshow('img', img1) cv2.waitKey(0) cv2.destroyAllWindows()上述代码的作用
这段代码使用了dlib库和OpenCV库来识别一张人脸照片中的68个关键点,并在照片中用绿色圆圈和红色数字标注这些关键点的位置。具体流程如下:
1. 导入需要用到的库:dlib、numpy和OpenCV。
2. 定义模型文件路径和获取人脸检测器对象,这里使用dlib库提供的get_frontal_face_detector()函数获取一个人脸检测器。
3. 加载一张人脸照片并将其转换成灰度图像。
4. 使用人脸检测器对象对灰度图像进行人脸检测,返回一个由矩形框组成的列表,每个矩形框表示一个检测到的人脸。
5. 对于每一个检测到的人脸,使用dlib库中的shape_predictor()函数获取一个关键点检测器,该检测器能够检测出人脸的68个关键点。
6. 使用关键点检测器对人脸图像中的关键点进行检测,返回一个由68个关键点组成的矩阵,每个关键点由x、y坐标表示。
7. 遍历矩阵中的每个关键点,使用cv2.circle()函数在照片中绘制一个绿色的圆圈,并使用cv2.putText()函数在圆圈旁边绘制一个红色数字,表示该关键点的序号。
8. 使用cv2.imshow()函数将标注好关键点的照片显示出来。
9. 使用cv2.waitKey()函数等待用户按下任意键。
10. 使用cv2.destroyAllWindows()函数关闭所有窗口。
相关推荐
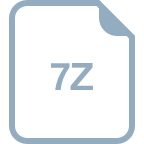












