深度可分离卷积 pytorch
时间: 2023-09-25 17:14:36 浏览: 159
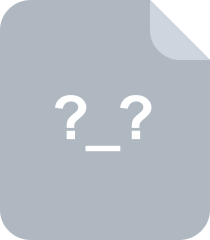
MixConv:混合感受野的深度可分离卷积(Pytorch实现)

深度可分离卷积是一种常用于减少模型参数量和计算量的卷积操作。在pytorch中,可以使用nn.Conv2d进行深度可分离卷积的实现。具体来说,深度可分离卷积包括两个步骤:depthwise卷积和pointwise卷积。
depthwise卷积是指将每个输入通道分别进行卷积操作,即每个卷积核只在一个通道上进行卷积。这样可以有效地减少参数量和计算量。在pytorch中,可以使用nn.Conv2d设置groups参数等于输入通道数,以实现depthwise卷积。
pointwise卷积是指在depthwise卷积之后,再对每个通道进行融合操作。这里的融合操作是通过使用1x1的卷积核实现的。在pytorch中,可以使用nn.Conv2d设置kernel_size参数为1,以实现pointwise卷积。
总体而言,深度可分离卷积能够显著减少模型的参数量和计算量,常用于轻量化的模型设计,例如MobileNet。在pytorch中,可以使用自定义的depthwise_separable_conv类来实现深度可分离卷积。该类中的depthwise和pointwise分别表示depthwise卷积和pointwise卷积的操作。通过调用forward函数即可完成深度可分离卷积的前向传播。
阅读全文
相关推荐















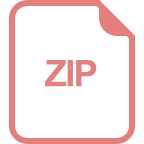