可解释性 transformer的代码
时间: 2024-08-01 20:00:42 浏览: 76
可解释性Transformer是一种将传统的Transformer模型与解释技术相结合的设计,旨在提高神经网络模型的透明度和可理解性。这类模型通常会引入额外的结构或者机制,如注意力可视化、局部敏感性分析等,帮助我们理解模型预测背后的原因。
下面是一个简化版的Python代码片段,展示了如何使用Hugging Face Transformers库中的BERT模型并添加一些可解释性模块(例如LIME):
```python
from transformers import BertTokenizer, BertForSequenceClassification, lime
import torch
# 加载预训练的BERT模型和分词器
tokenizer = BertTokenizer.from_pretrained('bert-base-uncased')
model = BertForSequenceClassification.from_pretrained('bert-base-uncased', num_labels=2)
def encode_text(text):
inputs = tokenizer.encode_plus(text, padding='max_length', truncation=True, max_length=512)
return inputs['input_ids'], inputs['attention_mask']
# 获取输入数据
text = "这是一段文本"
ids, masks = encode_text(text)
# 将输入转化为PyTorch张量
inputs = torch.tensor([ids], dtype=torch.long), torch.tensor([masks], dtype=torch.bool)
# 预测并获取输出
with torch.no_grad():
outputs = model(**inputs)
prediction = torch.argmax(outputs.logits, dim=1).item()
# 使用LIME进行解释
explainer = lime.lime_text.LimeTextExplainer(kernel_width=0.7)
exp = explainer.explain_instance(inputs.numpy(), model.predict_proba, labels=[prediction])
```
在这个例子中,`explain_instance()`函数会对输入文本生成局部解释,显示哪些部分对最终分类决策影响最大。请注意,实际应用中可能需要更复杂的代码来处理模型的解释过程,并可能涉及到其他可解释性工具库。
相关推荐
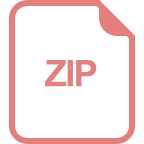
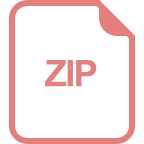
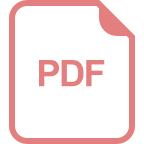














