function [c,y] = aPCE(po,input,input_mc) % input: number of variables (raw) x number of data (column) % number of random variables [nrv,~] = size(input); % multi index alpha = multi_index(nrv,po); % number of samples to make an aPCE model ns_param = 2; num_pol_term = length(alpha); % number of polynomial basis functions ns = ns_param*num_pol_term; % allocate input x1 = input(1,:); x2 = input(2,:); x3 = input(3,:); % training samples % inputs x1_sample = datasample(input(1,:),ns,'Replace',true); x2_sample = datasample(input(2,:),ns,'Replace',true); x3_sample = datasample(input(3,:),ns,'Replace',true); % output y_sample = ishigami([x1_sample;x2_sample;x3_sample]); y_sample = y_sample'; % MCS samples x1_mc = input_mc(1,:); x2_mc = input_mc(2,:); x3_mc = input_mc(3,:); % coefficients of polynomial basis functions for cp = 0:po c1 = aPCE_coef(cp,x1); c2 = aPCE_coef(cp,x2); c3 = aPCE_coef(cp,x3); Psi1(cp+1,:) = polyval(c1,x1_sample); Psi2(cp+1,:) = polyval(c2,x2_sample); Psi3(cp+1,:) = polyval(c3,x3_sample); Psi1_mc(cp+1,:) = polyval(c1,x1_mc); Psi2_mc(cp+1,:) = polyval(c2,x2_mc); Psi3_mc(cp+1,:) = polyval(c3,x3_mc); end % multivariate polynomial function for j = 1:num_pol_term Psi(:,j) = Psi1( alpha(j,1)+1,: ).*Psi2( alpha(j,2)+1,: ).*Psi3( alpha(j,3)+1,: ); Psi_mc(:,j) = Psi1_mc( alpha(j,1)+1,: ).*Psi2_mc( alpha(j,2)+1,: ).*Psi3_mc( alpha(j,3)+1,: ); end % PCE coefficients by ordinary least square c = double( lscov(Psi, y_sample) ); % MCS y = Psi_mc*c;
时间: 2024-04-18 15:26:16 浏览: 98
这段代码是用于实现基于多项式混沌展开(aPCE)的模型训练和预测。下面是代码的主要步骤解释:
1. 获取输入变量的数量和样本数据的数量。
2. 使用多重指标函数计算多重指标 alpha。
3. 定义用于构建 aPCE 模型的样本数量和多项式基函数的数量。
4. 对每个输入变量进行随机采样,得到训练样本。
5. 使用采样的训练样本作为输入,计算相应的输出样本。
6. 对每个输入变量的多项式基函数进行求解,并在采样的训练样本和 Monte Carlo 模拟样本上进行求解。
7. 构建多变量多项式函数,通过乘积形式组合各个单变量多项式基函数。
8. 使用普通最小二乘法(Ordinary Least Squares)计算 PCE 的系数 c。
9. 使用 Monte Carlo 模拟样本和计算得到的系数 c,预测输出 y。
希望对你有所帮助!如果还有其他问题,请随时提问。
相关问题
function [C,raw_mom]=aPCE_coef(po,x) % input % po: polynomial order % x: data (1 column) % % output % C: coefficients of a polynomial (highest to lowest) % raw_mom: raw momoments used for getting C % k-th raw moments krm = zeros(1,2*po); for rm = 0:2*po-1 krm(rm+1) = mean( x.^rm ); end % form matrices to get the coefficients A = zeros(po+1,po+1); for br = 1:po A(br,:) = krm(br:br+po); end A(end,end) = 1; B = zeros(po+1,1); B(end) = 1; % determine coefficients C = flipud(A^(-1)*B); % output option if nargout == 2 raw_mom = krm; end
这段代码是用于计算多项式混沌展开(aPCE)的系数和原始矩的函数。下面是代码的主要步骤解释:
1. 计算输入数据 x 的 k 阶原始矩,其中 k 的范围为 0 到 2*po-1。
2. 构建用于求解系数的矩阵 A 和向量 B。矩阵 A 的每一行都是 k 阶原始矩的一部分,矩阵 B 是一个全零向量,只有最后一个元素为 1。
3. 使用矩阵 A 和向量 B,通过求解线性方程组得到多项式的系数 C。注意,这里使用了矩阵的逆来求解。
4. 如果输出选项为 2,那么同时返回原始矩 raw_mom。
希望对你有所帮助!如果还有其他问题,请随时提问。
function [C, raw_mom] aPCE_coef(po, x)这个是什么意思
这段代码定义了一个函数 `aPCE_coef(po, x)`,用于计算多项式混沌展开的系数。
函数的输入参数为 `po` 和 `x`,分别表示多项式的阶数和数据(1列)。
函数的输出结果是 `C` 和 `raw_mom`。其中,`C` 是多项式的系数(从高到低排序),`raw_mom` 是用于计算系数 `C` 的原始矩。
通过调用这个函数,并传入适当的参数,你可以得到多项式混沌展开的系数和原始矩。
希望这解释清楚了这段代码的意义。如果还有其他问题,请随时提问。
阅读全文
相关推荐
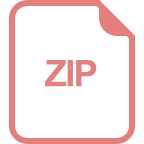
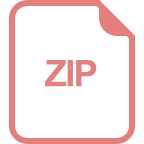
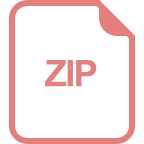
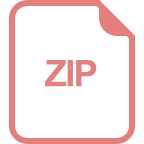
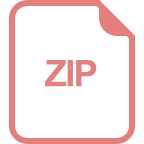
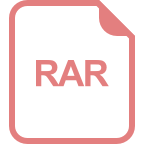
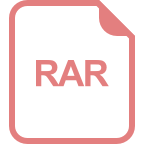
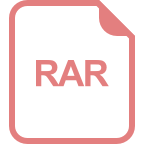
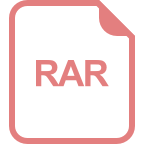
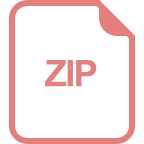
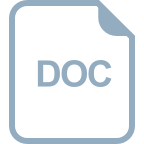
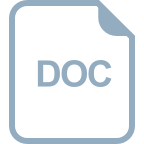

