层次聚类中的DIANA 与K_means的关系
时间: 2024-06-07 11:11:01 浏览: 15
层次聚类和 K-means 是两种不同的聚类算法,它们有一些不同之处,DIANA 是层次聚类中的一种方法。
K-means 聚类是一种基于质心的聚类算法,它需要预先指定聚类数 k,然后从数据集中随机选择 k 个中心点,并将每个数据点分配给最近的中心点,最后重新计算每个簇的中心点,不断迭代直到收敛。K-means 聚类的优点是计算速度快,但需要提前指定聚类数,对于不同的数据集需要尝试不同的聚类数才能得到较好的聚类结果。
层次聚类是一种基于树状结构的聚类方法,它可以根据数据点之间的相似性构建一个树形结构。层次聚类分为两种:凝聚层次聚类(AGNES)和分裂层次聚类(DIANA)。其中 DIANA 是分裂层次聚类的一种,它的聚类过程是自顶向下的,从整个数据集开始,每次选择最不相似的数据点进行划分,直到满足一定的条件为止。层次聚类的优点是不需要预先指定聚类数,可以得到一颗完整的树形结构,但计算复杂度较高。
在实际应用中,K-means 和层次聚类都有各自的优缺点,需要根据具体的问题选择适当的算法。同时,DIANA 也可以看做是一种层次聚类算法的优化,它可以通过一些启发式方法加快聚类速度,同时避免 AGNES 中出现的链式效应问题。
相关问题
如何对文本进行层次聚类
对文本进行层次聚类,一般可以采用以下几个步骤:
1.将文本数据表示为向量,可以使用词袋模型、tf-idf模型、word2vec模型等方法将文本转化为向量。
2.选择合适的聚类算法,例如k-means算法、层次聚类算法、DBSCAN算法等。
3.根据聚类算法和相似度度量标准,对文本进行聚类。
4.根据聚类结果,对文本进行可视化和解释。
层次聚类算法中,一般采用自底向上(AGNES算法)或自顶向下(DIANA算法)两种方法。自顶向下方法的优点是速度快,缺点是聚类结果不够准确;自底向上方法的优点是聚类结果准确,缺点是速度相对较慢。根据数据集的大小和复杂度,可以选择合适的算法来对文本进行层次聚类。
对K-Means、AGNES、DIANA算法进行比较
K-Means、AGNES、DIANA算法都是聚类算法,但它们的思想和实现方式不同。
K-Means算法是一种基于距离的聚类算法,它将数据集分成K个簇,每个簇的中心点是该簇中所有点的均值。K-Means算法的优点是简单易用,但缺点是需要预先指定簇的数量K,且对于不同的初始中心点选择,最终的聚类结果可能不同。
AGNES(Agglomerative Nesting)算法是一种自下而上的层次聚类算法,其思想是将每个数据点看成一个簇,然后将距离最近的两个簇合并为一个新的簇,直到所有数据点都被合并到一个簇中。AGNES算法的优点是不需要预先指定簇的数量K,但缺点是合并过程中需要计算所有簇之间的距离,计算量较大,且容易受到异常值的影响。
DIANA(Divisive Analysis)算法是一种自上而下的分裂聚类算法,其思想是将所有数据点看成一个簇,然后递归地将簇一分为二,直到每个簇只包含一个数据点为止。DIANA算法的优点是不需要预先指定簇的数量K,但缺点是分裂过程中需要计算所有点之间的距离,计算量也较大。
综上所述,K-Means、AGNES、DIANA算法各有优缺点,选择哪种算法取决于具体的应用场景和数据特点。
相关推荐
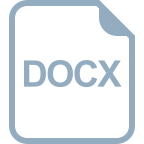
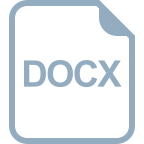
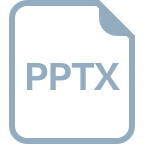









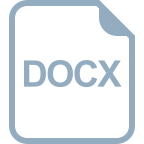
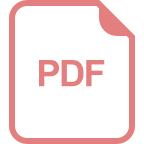
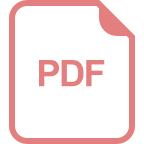
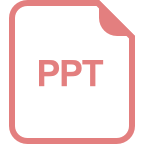