grid = self.get_grid(x.shape, x.device) x = torch.cat((x, grid), dim=-1) x = self.p(x) x = x.permute(0, 3, 1, 2)
时间: 2024-05-25 22:13:49 浏览: 19
这段代码的作用是什么?
这段代码的作用是在输入张量 x 的最后一个维度上拼接一个网格矩阵,然后将结果输入到一个卷积神经网络中。具体来说:
- self.get_grid(x.shape, x.device) 返回一个与 x 的形状相同的网格矩阵,其中每个元素的值表示该元素在张量中的坐标;
- torch.cat((x, grid), dim=-1) 在最后一个维度上将 x 和网格矩阵 grid 进行拼接;
- self.p(x) 对拼接后的张量进行卷积操作;
- x.permute(0, 3, 1, 2) 将通道维移动到第二个维度上,以符合 PyTorch 的张量表示规范。
这段代码可能用于图像分割模型中,将输入图像的每个像素点的坐标信息以及其他特征信息一起输入到卷积神经网络中进行处理,以提高模型的精度。
相关问题
H = self.hermite_domain.to(device=x.device, dtype=torch.float32)
这段代码中,`self.hermite_domain`是一个`HermiteDomain`对象,`to`方法将该对象移动到指定的设备上,并将其类型转换为`torch.float32`。其中,`device=x.device`表示使用与输入参数`x`所在的设备相同的设备,以确保张量之间的计算能够顺利进行。`dtype=torch.float32`则表示将类型转换为32位浮点型。
请分析下面代码 x1 = self.primary_conv(x) x2 = self.cheap_operation(x1) out = torch.cat([x1, x2], dim=1) return out[:, :self.oup, :, :]
这段代码是一个神经网络模型的一部分,它接受输入张量x并进行一系列操作后返回输出张量out。下面是对代码的分析:
1. 第一行代码`x1 = self.primary_conv(x)`表示通过`self.primary_conv`函数对输入x进行卷积操作,得到中间结果x1。
2. 第二行代码`x2 = self.cheap_operation(x1)`表示对x1进行一些廉价操作,得到中间结果x2。
3. 第三行代码`out = torch.cat([x1, x2], dim=1)`表示将x1和x2在维度1上进行拼接,得到拼接后的张量out。
4. 最后一行代码`return out[:, :self.oup, :, :]`表示返回out张量的一个子集。它通过切片操作选择out张量的前self.oup个通道,并保留所有的行和列。
总结起来,这段代码的功能是对输入张量进行卷积操作和廉价操作,然后将这两个操作的结果在通道维度上拼接,并返回部分通道的输出。
相关推荐
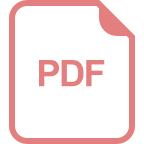
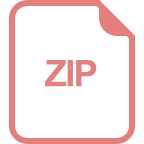
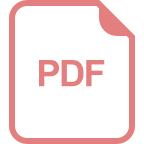













